Computationally Expensive High-Dimensional Multiobjective Optimization via Surrogate-Assisted Reformulation and Decomposition
IEEE Transactions on Evolutionary Computation(2024)
摘要
In recent decades, various surrogate-assisted evolutionary algorithms (SAEAs) have been proposed to solve computationally expensive multiobjective optimization problems (EMOPs). Nevertheless, designing an SAEA to handle high-dimensional EMOPs and balance convergence, diversity, and computational complexity remains challenging. Here, we propose a two-phase SAEA (TP-SAEA), which follows the idea of convergence first and diversity second, for solving high-dimensional EMOPs. In Phase I, a surrogate-assisted problem reformulation method is proposed to fast-track the Pareto optimal set in association with some reference solutions. Specifically, the high-dimensional EMOP is reformulated into an expensive single-objective one with low-dimensional decision space. Then, the surrogate-assisted optimization is utilized to obtain well-converged solutions. In Phase II, the high-dimensional EMOP is decomposed into two subproblems to explore subregions of the decision space that can effectively promote the diversity of the solutions. The two subproblems are optimized independently via surrogate-assisted optimization, aiming to push the population towards different regions of the Pareto optimal front. Experiments are conducted on EMOPs with 100 to 500 decision variables compared with four state-of-the-art SAEAs. The proposed TP-SAEA obtains well-converged and diverse solutions with only 509 real function evaluations. Moreover, its superiority is examined in six real-world instances with up to 12,000 decision variables.
更多查看译文
关键词
Multiobjective optimization,surrogate-assisted optimization,high-dimensional optimization
AI 理解论文
溯源树
样例
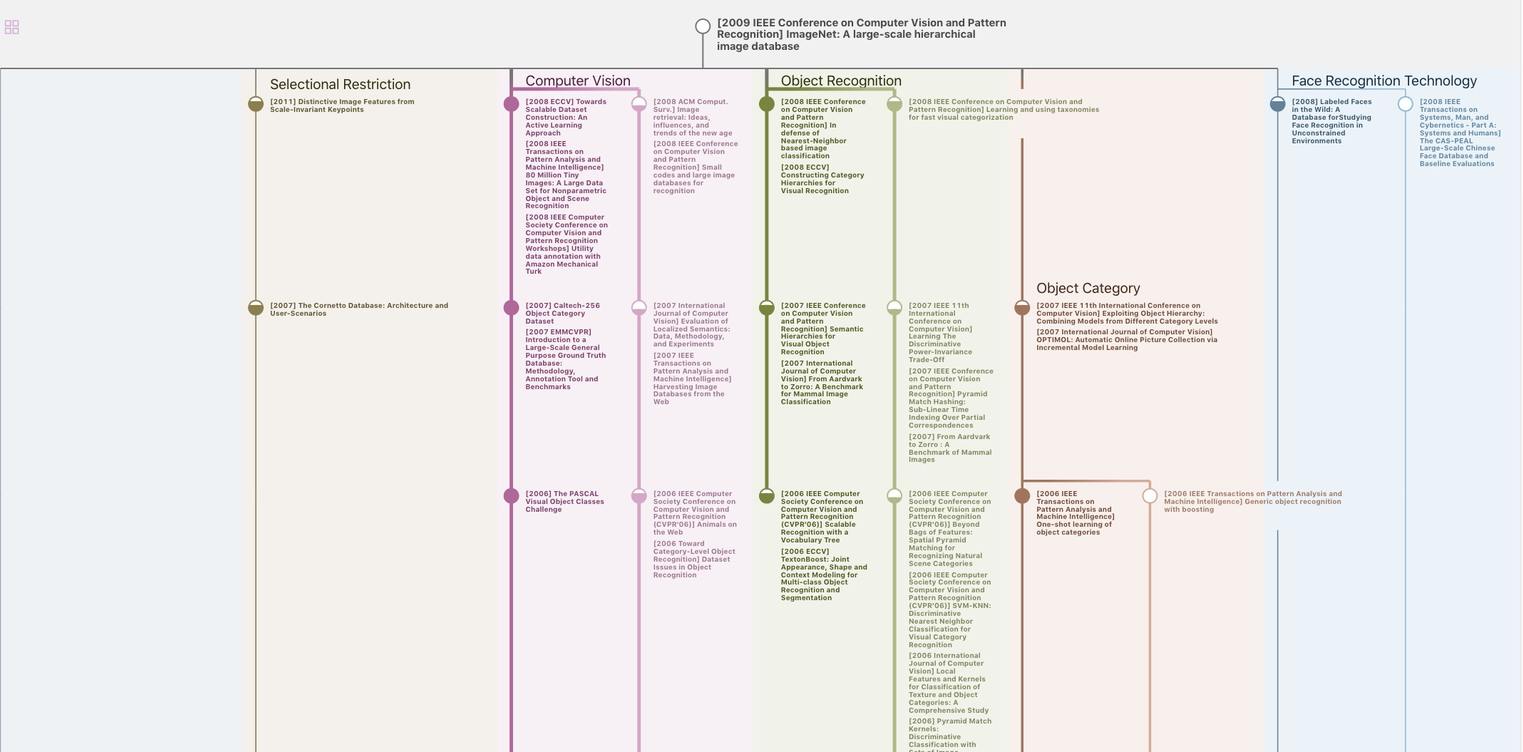
生成溯源树,研究论文发展脉络
Chat Paper
正在生成论文摘要