A Model-Based MR Parameter Mapping Network Robust to Substantial Variations in Acquisition Settings
MEDICAL IMAGE ANALYSIS(2024)
关键词
Magnetic resonance imaging,Parameter mapping,Deep learning,Regularization
AI 理解论文
溯源树
样例
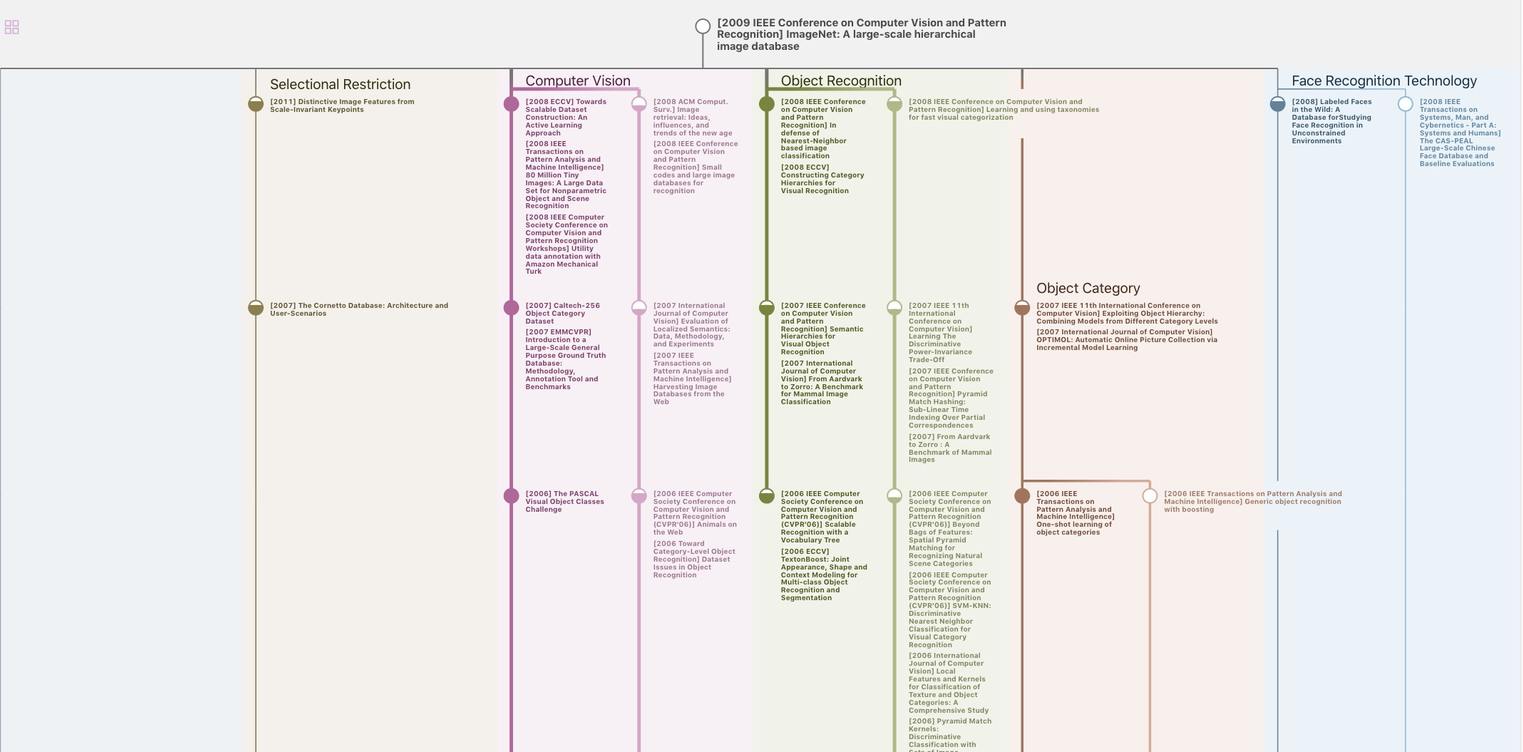
生成溯源树,研究论文发展脉络
Chat Paper
正在生成论文摘要