The Dawn of AI-Native EDA: Promises and Challenges of Large Circuit Models
arxiv(2024)
摘要
Within the Electronic Design Automation (EDA) domain, AI-driven solutions
have emerged as formidable tools, yet they typically augment rather than
redefine existing methodologies. These solutions often repurpose deep learning
models from other domains, such as vision, text, and graph analytics, applying
them to circuit design without tailoring to the unique complexities of
electronic circuits. Such an AI4EDA approach falls short of achieving a
holistic design synthesis and understanding, overlooking the intricate
interplay of electrical, logical, and physical facets of circuit data. This
perspective paper argues for a paradigm shift from AI4EDA towards AI-native
EDA, integrating AI at the core of the design process. Pivotal to this vision
is the development of a multimodal circuit representation learning technique,
poised to provide a comprehensive understanding by harmonizing and extracting
insights from varied data sources, such as functional specifications, RTL
designs, circuit netlists, and physical layouts.
We champion the creation of large circuit models (LCMs) that are inherently
multimodal, crafted to decode and express the rich semantics and structures of
circuit data, thus fostering more resilient, efficient, and inventive design
methodologies. Embracing this AI-native philosophy, we foresee a trajectory
that transcends the current innovation plateau in EDA, igniting a profound
shift-left in electronic design methodology. The envisioned advancements herald
not just an evolution of existing EDA tools but a revolution, giving rise to
novel instruments of design-tools that promise to radically enhance design
productivity and inaugurate a new epoch where the optimization of circuit
performance, power, and area (PPA) is achieved not incrementally, but through
leaps that redefine the benchmarks of electronic systems' capabilities.
更多查看译文
AI 理解论文
溯源树
样例
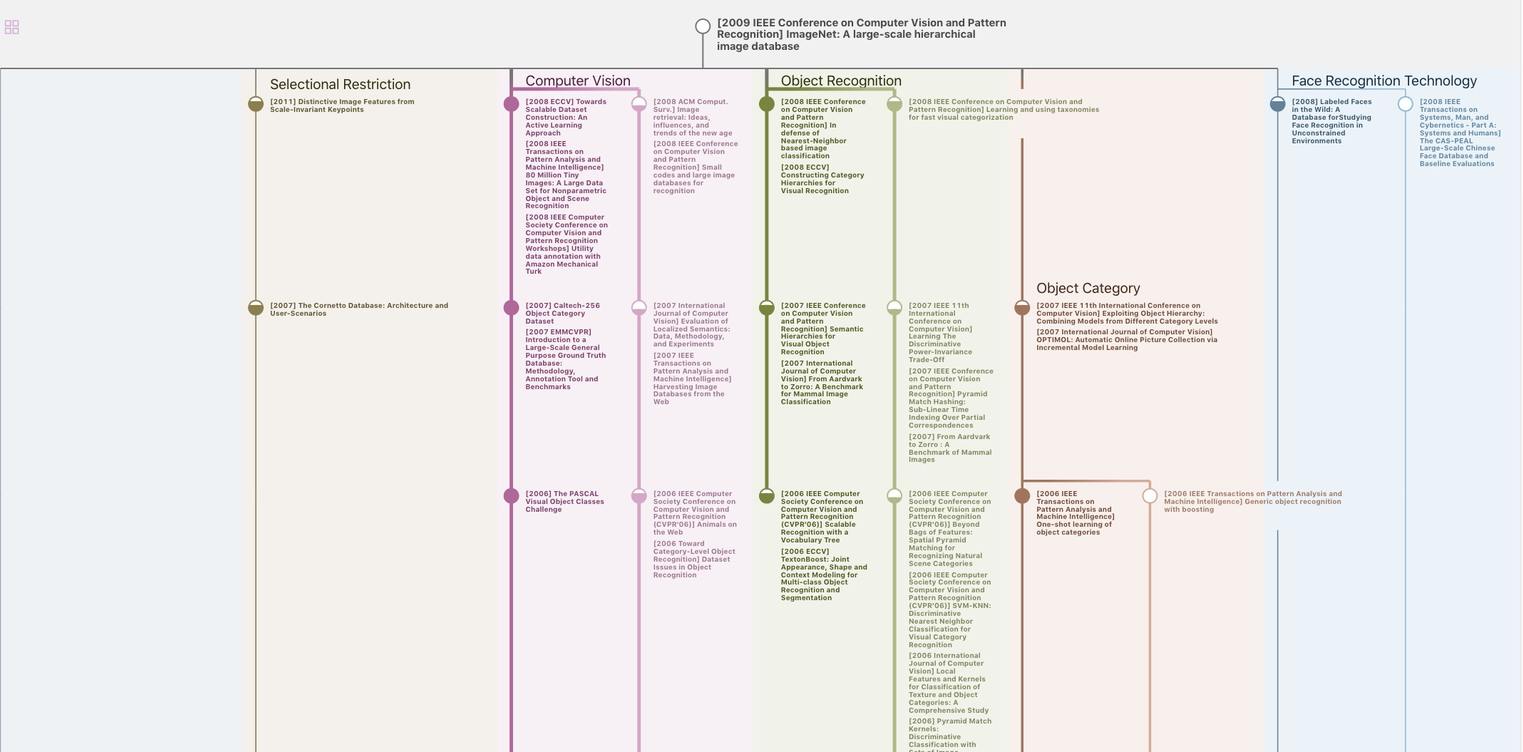
生成溯源树,研究论文发展脉络
Chat Paper
正在生成论文摘要