MCICT: Graph convolutional network-based end-to-end model for multi-label classification of imbalanced clinical text
BIOMEDICAL SIGNAL PROCESSING AND CONTROL(2024)
摘要
The rapid growth of clinical text data requires accurate and powerful automated classification methods to support medical decision making and personalized healthcare. The multi-label classification task for clinical texts is designed to assign the most relevant set of labels to each clinical text. However, this task presents two significant challenges: (1) how to accurately extract fine-grained semantic features from complex clinical texts, and (2) how to effectively mitigate the issue of label class imbalance. To overcome these problems, we innovatively propose a novel Multi-label Classification of Imbalanced Clinical Text (MCICIT) model. In order to obtain fine-grained semantic features from clinical texts, we utilize the specialized pre-trained language model BioBERT, tailored for biomedical texts. To tackle the challenge of label class imbalance, we present a Co-occurrence Based and Embeddings with Additional Information Enhanced Graph Convolutional Network (CoEAI-GCN) module. On one hand, we enrich the label content by incorporating additional information to acquire more accurate word embeddings as the feature matrix. On the other hand, we combine the cooccurrence relationship of labels to construct a correlation matrix. Ultimately, label representations are learned through a graph convolutional network. By conducting multi-label classification experiments on two clinical text datasets extracted from real medical systems, our model achieves a 3.2% and 0.5% improvement in F1 scores, respectively, compared to state -of -the -art deep learning models. Additionally, we conduct ablation studies to explore the behaviors of the proposed model. These results together demonstrate that our proposed MCICT effectively enhances the classification performance of imbalanced clinical texts.
更多查看译文
关键词
Multi-label text classification,Clinical text classification,Graph convolutional neural network,Label co-occurrence,Additional information
AI 理解论文
溯源树
样例
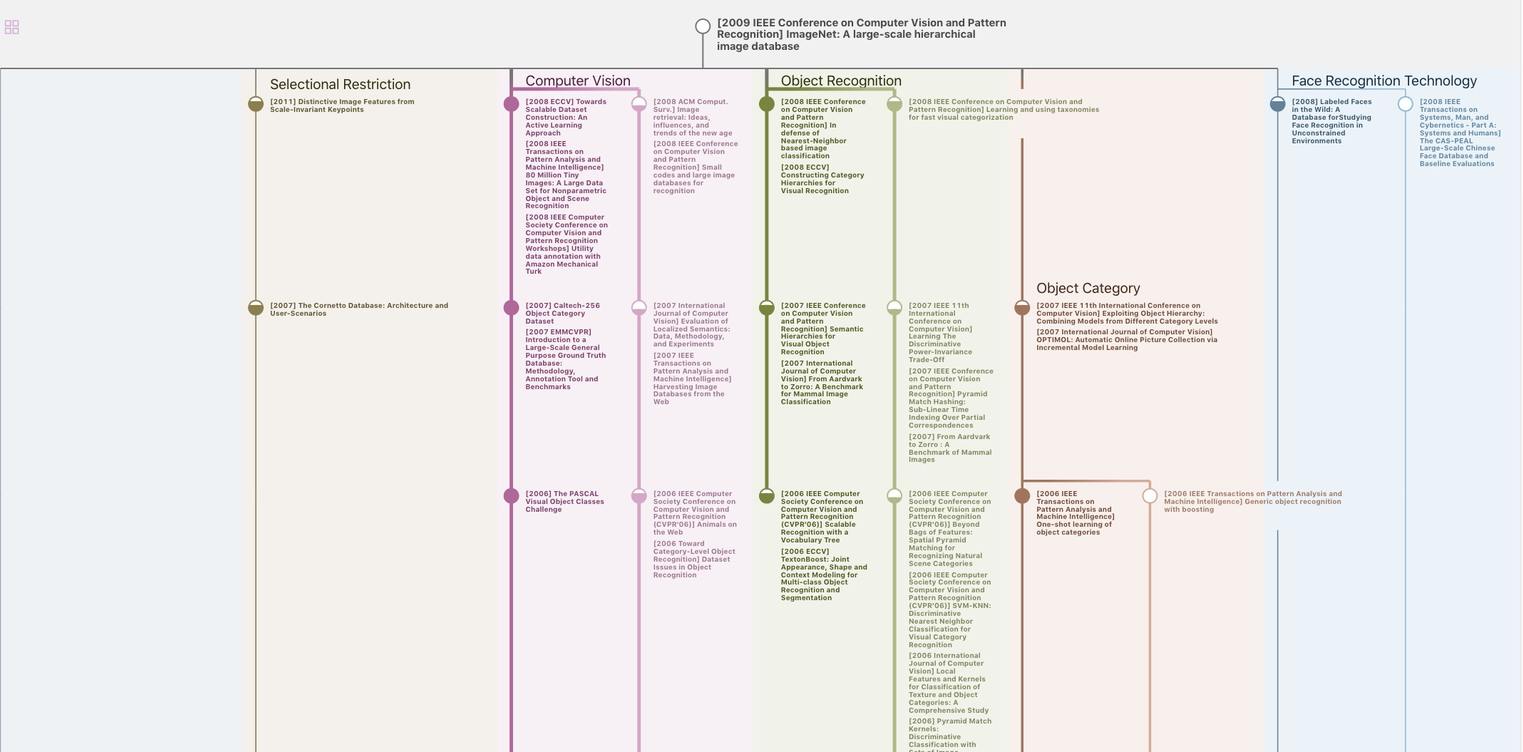
生成溯源树,研究论文发展脉络
Chat Paper
正在生成论文摘要