HyperDID: Hyperspectral Intrinsic Image Decomposition with Deep Feature Embedding
IEEE TRANSACTIONS ON GEOSCIENCE AND REMOTE SENSING(2024)
Chinese Acad Mil Sci
Abstract
The dissection of hyperspectral images (HSIs) into intrinsic components through hyperspectral intrinsic image decomposition (HIID) enhances the interpretability of hyperspectral data, providing a foundation for more accurate classification outcomes. However, the classification performance of HIID is constrained by the model's representational ability. To address this limitation, this study rethinks HIID for classification tasks by introducing deep feature embedding. The proposed framework, HyperDID, incorporates the environmental feature module (EFM) and categorical feature module (CFM) to extract intrinsic features. In addition, a feature discrimination module (FDM) is introduced to separate environment-related and category-related features. Experimental results across three commonly used datasets validate the effectiveness of HyperDID in improving HSI classification performance. This novel approach holds promise for advancing the capabilities of HSI analysis by leveraging deep feature embedding principles. The implementation of the proposed method can be accessed soon at https://github.com/shendu-sw/HyperDID for the sake of reproducibility.
MoreTranslated text
Key words
Convolutional neural networks (CNNs),deep learning,feature embedding,hyperspectral image (HSI) classification,intrinsic image decomposition
PDF
View via Publisher
AI Read Science
Must-Reading Tree
Example
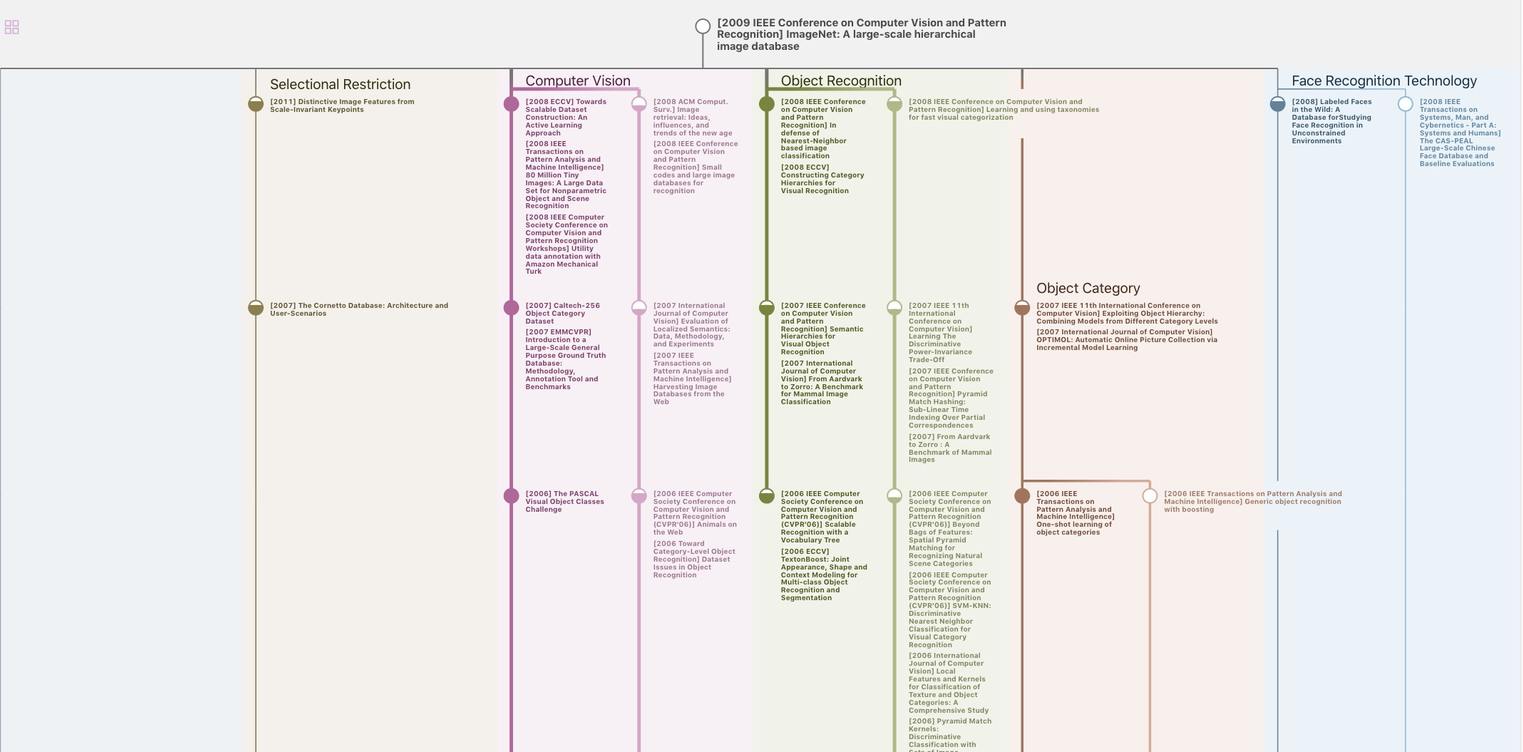
Generate MRT to find the research sequence of this paper
Data Disclaimer
The page data are from open Internet sources, cooperative publishers and automatic analysis results through AI technology. We do not make any commitments and guarantees for the validity, accuracy, correctness, reliability, completeness and timeliness of the page data. If you have any questions, please contact us by email: report@aminer.cn
Chat Paper
Summary is being generated by the instructions you defined