COSM2IC: Optimizing Real-Time Multi-Modal Instruction Comprehension
IEEE ROBOTICS AND AUTOMATION LETTERS(2022)
摘要
Supporting real-time, on-device execution of
multi-modal referring instruction comprehension
models is an important challenge to be tackled in embodied Human-Robot Interaction. However, state-of-the-art deep learning models are resource-intensive and unsuitable for real-time execution on embedded devices. While model compression can achieve a reduction in computational resources up to a certain point, further optimizations result in a severe drop in accuracy. To minimize this loss in accuracy, we propose the
COSM2IC
framework, with a lightweight Task Complexity Predictor, that uses multiple sensor inputs to assess the
instructional complexity
and thereby dynamically switch between a set of models of varying computational intensity such that computationally less demanding models are invoked whenever possible. To demonstrate the benefits of
COSM2IC
, we utilize a representative human-robot collaborative “table-top target acquisition” task, to curate a new multi-modal instruction dataset where a human issues instructions in a natural manner using a combination of visual, verbal, and gestural (pointing) cues. We show that
COSM2IC
achieves a 3-fold reduction in comprehension latency when compared to a baseline DNN model while suffering an accuracy loss of only
$\sim$
5%. When compared to state-of-the-art model compression methods,
COSM2IC
is able to achieve a further 30% reduction in latency and energy consumption for a comparable performance.
更多查看译文
关键词
Data sets for robotic vision, deep learning for visual perception, embedded systems for robotic and automation, human-robot collaboration, RGB-D perception
AI 理解论文
溯源树
样例
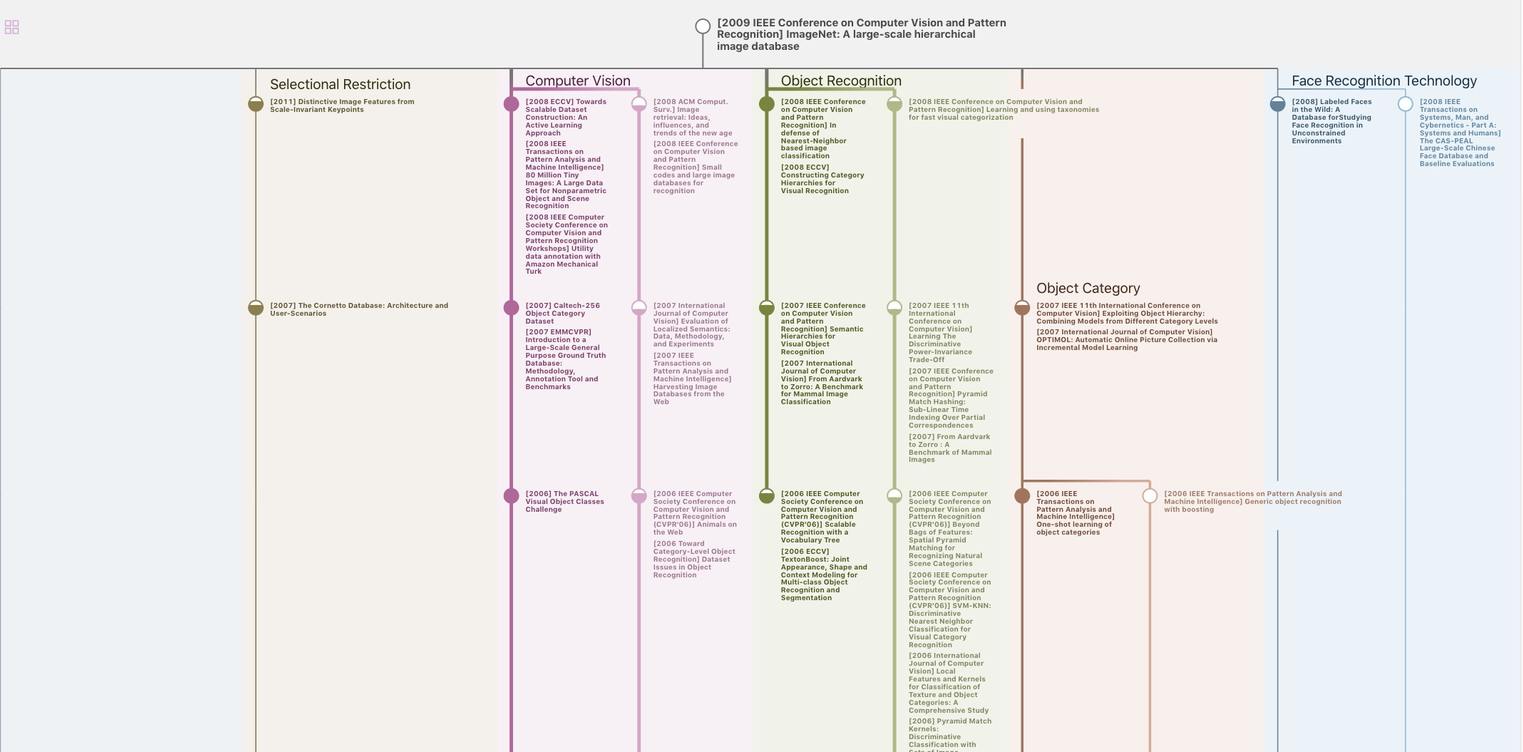
生成溯源树,研究论文发展脉络
Chat Paper
正在生成论文摘要