HEBO: Pushing The Limits of Sample-Efficient Hyperparameter Optimisation
JOURNAL OF ARTIFICIAL INTELLIGENCE RESEARCH(2022)
摘要
In this work we rigorously analyse assumptions inherent to black-box optimisation hyp er-parameter tuning tasks. Our results on the Bayesmark benchmark indicate that heteroscedasticity and non-stationarity pose significant challenges for black-box optimis-ers. Based on these findings, we propose a Heteroscedastic and Evolutionary Bayesian Optimisation solver (HEBO). HEBO performs non-linear input and output warping, admits exact marginal log-likelihood optimisation and is robust to the values of learned parameters. We demonstrate HEBO's empirical efficacy on the NeurIPS 2020 Black-Box Optimisation challenge, where HEBO placed first. Upon further analysis, we observe that HEBO significantly outperforms existing black-box optimisers on 108 machine learning hyperparameter tuning tasks comprising the Bayesmark benchmark. Our findings indicate that the majority of hyper-parameter tuning tasks exhibit heteroscedasticity and non-stationarity, multi-objective acquisition ensembles with Pareto front solutions improve queried configurations, and robust acquisition maximisers afford empirical advantages relative to their non-robust counterparts. We hope these findings may serve as guiding principles for practitioners of Bayesian optimisation.
更多查看译文
关键词
sample-efficient
AI 理解论文
溯源树
样例
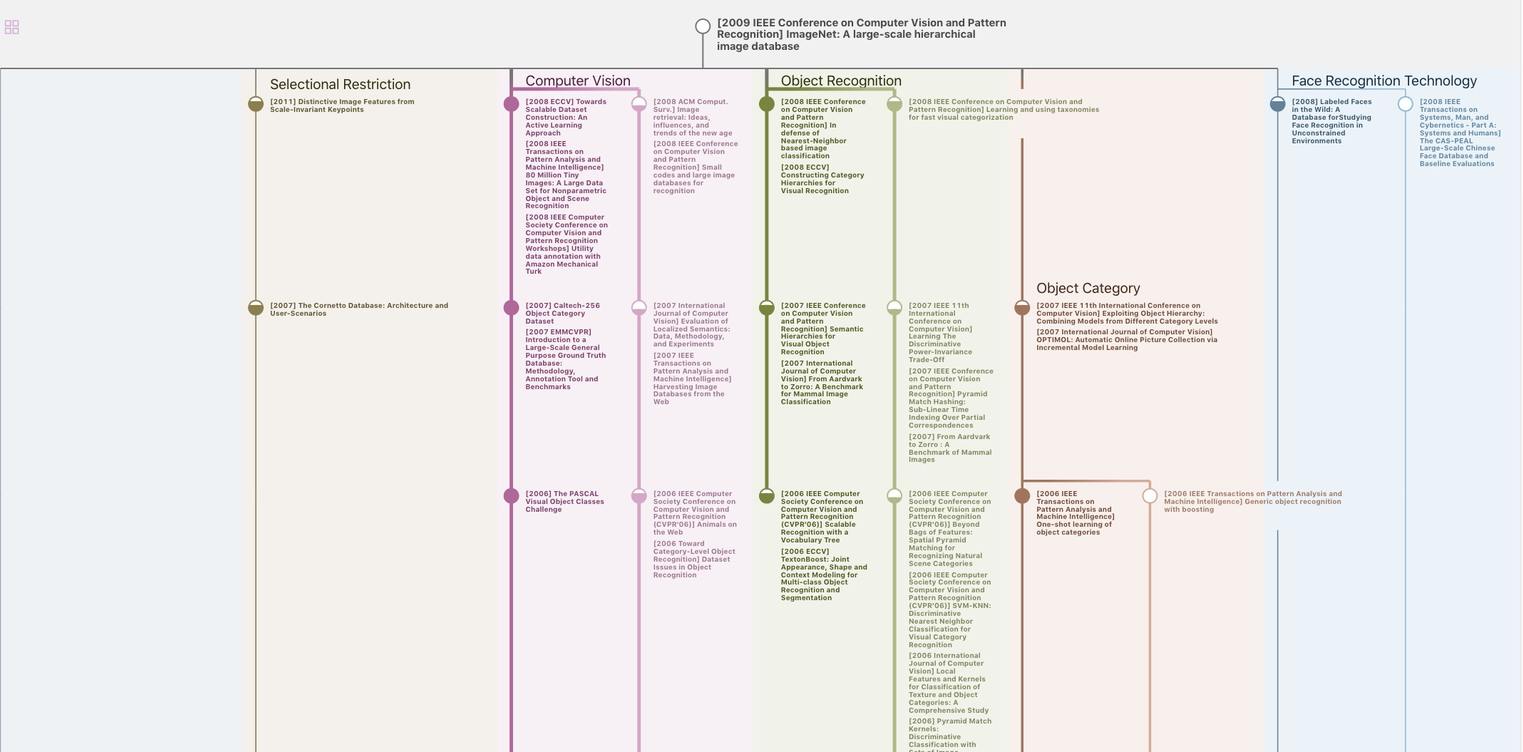
生成溯源树,研究论文发展脉络
Chat Paper
正在生成论文摘要