Local Search for Policy Iteration in Continuous Control
CoRR(2020)
Abstract
We present an algorithm for local, regularized, policy improvement in reinforcement learning (RL) that allows us to formulate model-based and model-free variants in a single framework. Our algorithm can be interpreted as a natural extension of work on KL-regularized RL and introduces a form of tree search for continuous action spaces. We demonstrate that additional computation spent on model-based policy improvement during learning can improve data efficiency, and confirm that model-based policy improvement during action selection can also be beneficial. Quantitatively, our algorithm improves data efficiency on several continuous control benchmarks (when a model is learned in parallel), and it provides significant improvements in wall-clock time in high-dimensional domains (when a ground truth model is available). The unified framework also helps us to better understand the space of model-based and model-free algorithms. In particular, we demonstrate that some benefits attributed to model-based RL can be obtained without a model, simply by utilizing more computation.
MoreTranslated text
Key words
policy iteration,continuous control,local search
PDF
View via Publisher
AI Read Science
Must-Reading Tree
Example
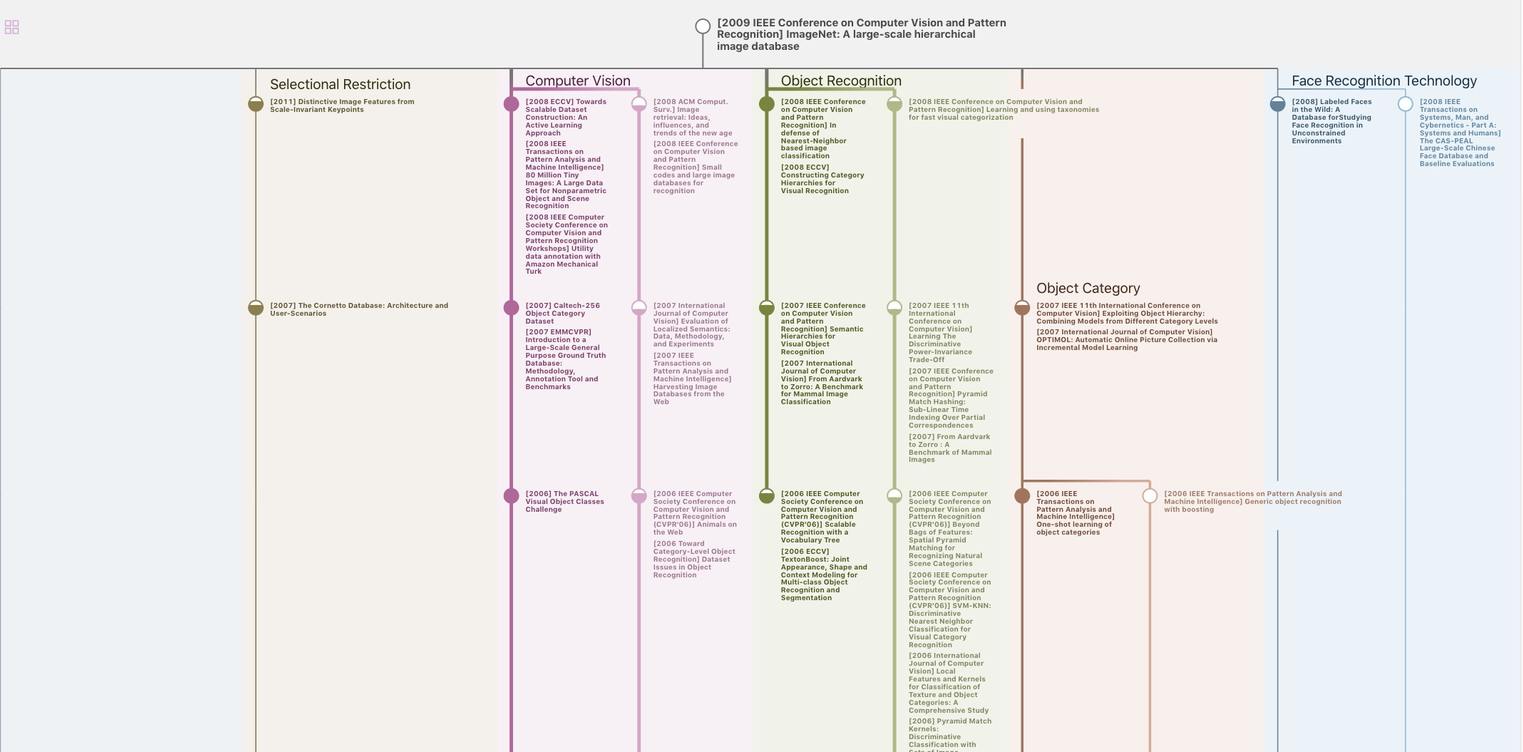
Generate MRT to find the research sequence of this paper
Data Disclaimer
The page data are from open Internet sources, cooperative publishers and automatic analysis results through AI technology. We do not make any commitments and guarantees for the validity, accuracy, correctness, reliability, completeness and timeliness of the page data. If you have any questions, please contact us by email: report@aminer.cn
Chat Paper
Summary is being generated by the instructions you defined