Deep Learning-Driven One-Shot Dual-View 3D Reconstruction for Dual-Projector System
IEEE TRANSACTIONS ON INSTRUMENTATION AND MEASUREMENT(2024)
Key words
Deep learning,fringe projection profilometry (FPP),real dataset,single-shot superimposed phase-shifting grating,two projection views 3-D reconstruction
AI Read Science
Must-Reading Tree
Example
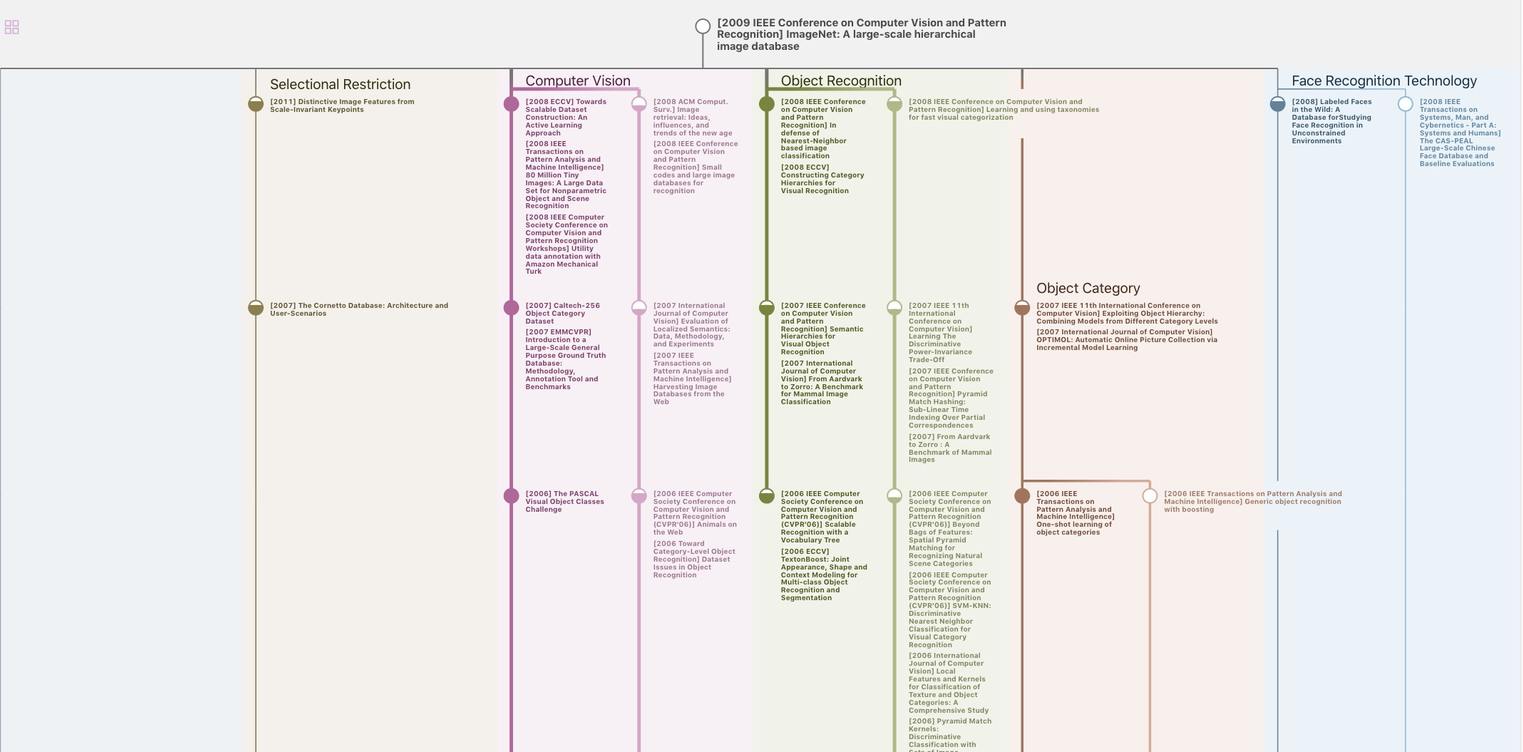
Generate MRT to find the research sequence of this paper
Chat Paper
Summary is being generated by the instructions you defined