Cone penetration test-based assessment of liquefaction potential using machine and hybrid learning approaches
Multiscale and Multidisciplinary Modeling, Experiments and Design(2024)
摘要
In soil mechanics, liquefaction is the phenomenon that occurs when saturated, cohesionless soils temporarily lose their strength and stiffness under cyclic loading shaking or earthquake. The present work introduces an optimal performance model by comparing two baselines, thirty tree-based, thirty support vector classifier-based, and fifteen neural network-based models in assessing the liquefaction potential. One hundred and seventy cone penetration test results (liquefied and non-liquefied) have been compiled from the literature for this aim. Earthquake magnitude, vertical-effective stress, mean grain size, cone tip resistance, and peak ground acceleration parameters have been used as input parameters to predict the soil liquefaction potential for the first time. Performance metrics, accuracy, an area under the curve (AUC), precision, recall, and F1 score have measured the training and testing performances. The comparison of performance metrics reveals that the model Runge–Kutta optimized extreme gradient boosting (RUN_XGB) has assessed the liquefaction potential with an overall accuracy of 99
更多查看译文
关键词
Liquefaction potential,Optimization techniques,Xtreme-gradient boosting,Neural networks,Multicollinearity
AI 理解论文
溯源树
样例
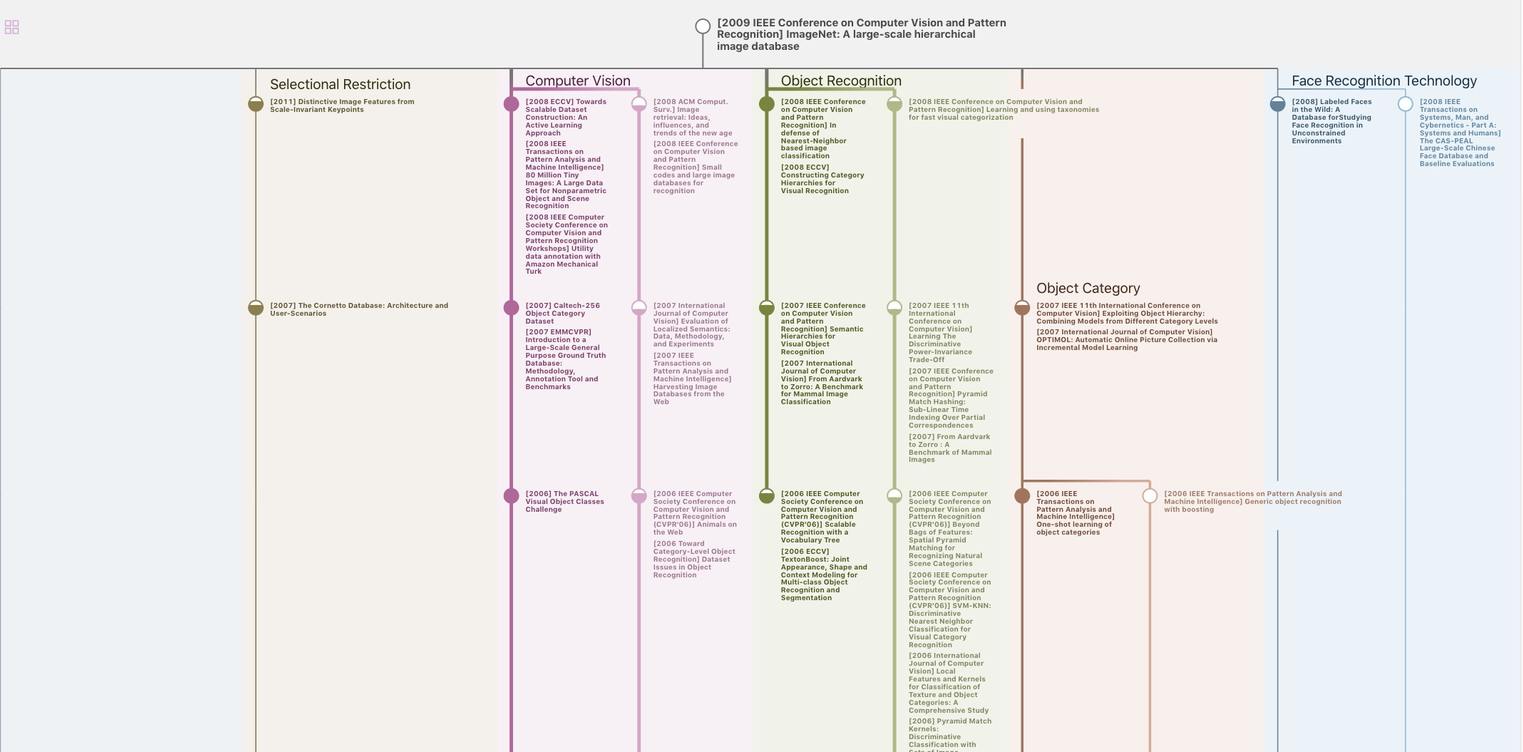
生成溯源树,研究论文发展脉络
Chat Paper
正在生成论文摘要