DLKNet: Dual Large Kernel Network for Real-Time Semantic Segmentation with Transformer Architecture
2024 4th International Conference on Consumer Electronics and Computer Engineering (ICCECE)(2024)
Key words
LKBlock,Real Time semantic,BHead
AI Read Science
Must-Reading Tree
Example
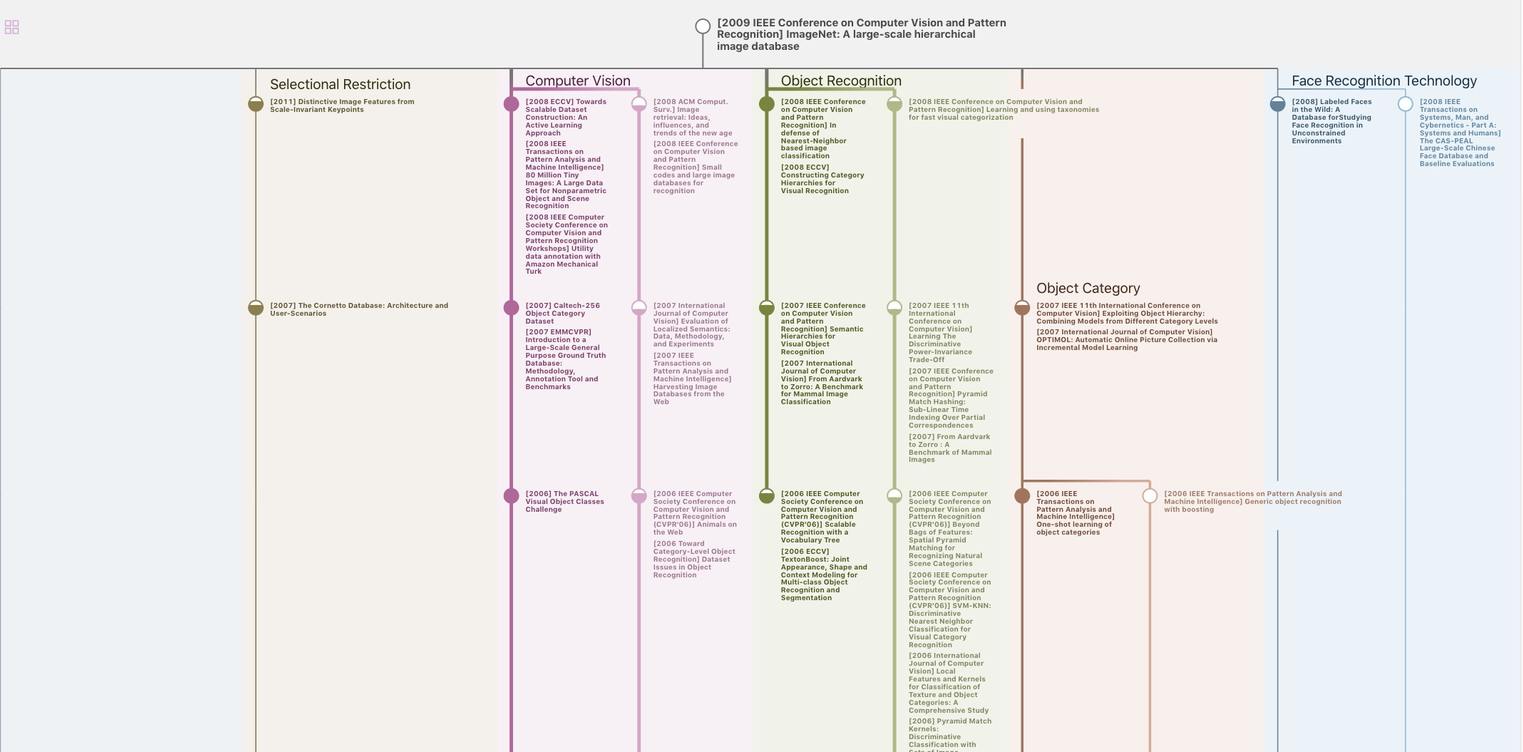
Generate MRT to find the research sequence of this paper
Chat Paper
Summary is being generated by the instructions you defined