Named Entity Recognition of Automotive Parts Based on RoBERTa-CRF Model
2024 4th International Conference on Neural Networks, Information and Communication (NNICE)(2024)
摘要
Named entity recognition (NER) serves as the foundation for constructing Knowledge Graphs (KG) and facilitating question answering. Traditional NER methods encounter challenges during the training process due to the limited size of annotated datasets. Additionally, the absence of word segmentation between Chinese characters further complicates the extraction of specific data from automotive accessory literature, given the extensive scale of the data involved. However, with the advent of pre-training models in natural language processing (NLP), remarkable advancements have been made in terms of semantic representation on large-scale, unlabeled corpora, yielding favorable outcomes for various NLP tasks. In this study, we compile a substantial amount of automotive accessory data and online texts for manual annotation, resulting in the establishment of automotive accessory datasets. Leveraging a pre-trained model, we aim to enhance the performance of NER within the automotive accessory domain. Firstly, our proposed approach treats NER as a downstream task of the pre-trained model, incorporating the acquired semantic representation into the model during the training process. Secondly, we conduct validation experiments to compare the effectiveness of the pre-training model with other deep learning models in querying named entities within the field of automotive accessory. Lastly, we employ the ERNIE model to augment the original datasets, thereby expanding their scope. The experimental results substantiate that the RoBERTa-CRF pre- training model outperforms previous methods, delivering the best performance in terms of F1 score.
更多查看译文
关键词
Named entity recognition(NER),Natural language processing(NLP),Pre-trained models,Automotive accessory
AI 理解论文
溯源树
样例
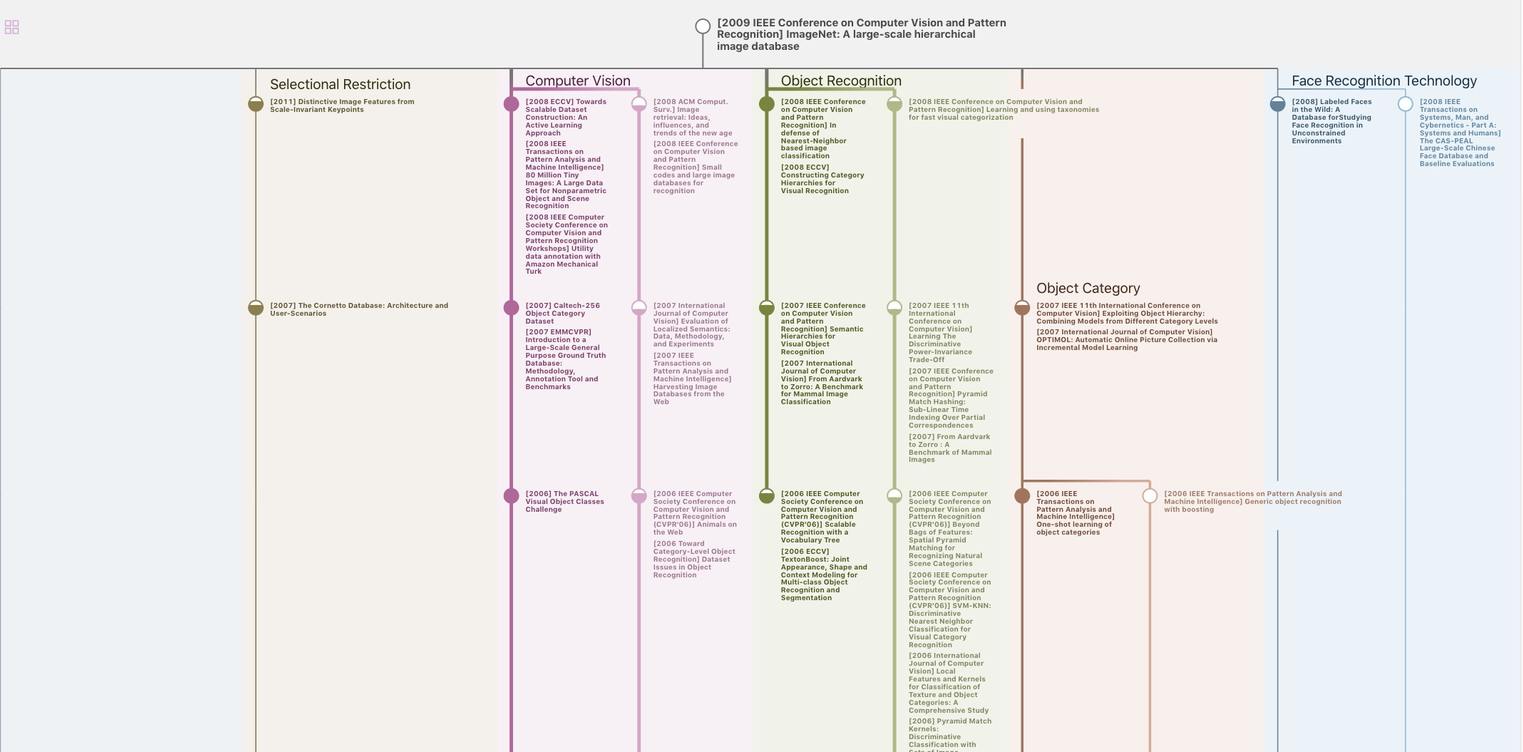
生成溯源树,研究论文发展脉络
Chat Paper
正在生成论文摘要