Estimation of Spatiotemporal Variability of Global Surface Ocean DIC Fields Using Ocean Color Remote Sensing Data
IEEE TRANSACTIONS ON GEOSCIENCE AND REMOTE SENSING(2024)
关键词
Oceans,Sea measurements,Sea surface,Spatiotemporal phenomena,Satellites,Surface treatment,Ocean temperature,Climate change,Carbon cycle,dissolved inorganic carbon (DIC),global ocean,multiparametric regression (MPR)
AI 理解论文
溯源树
样例
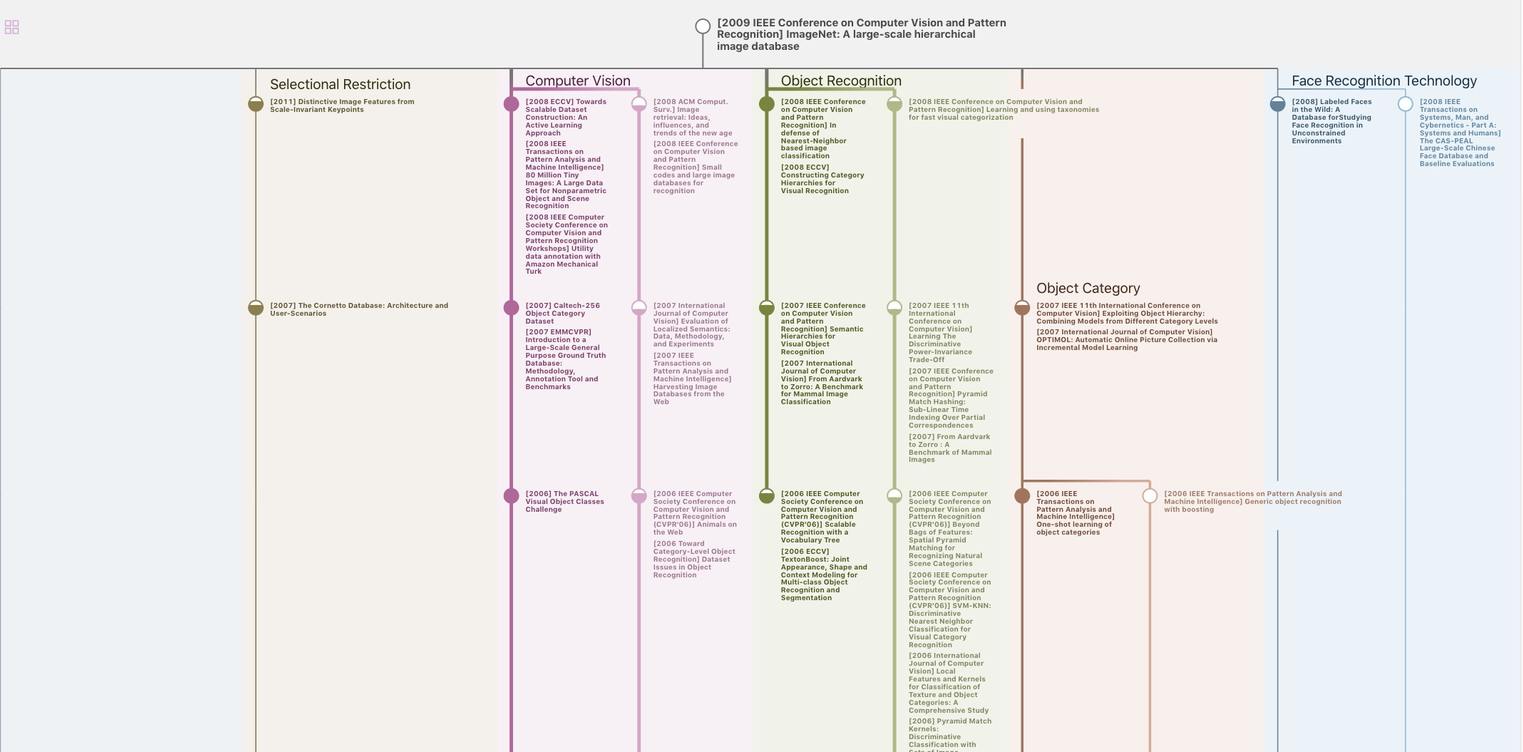
生成溯源树,研究论文发展脉络
Chat Paper
正在生成论文摘要