Bivariate Q- Generalized Extreme Value Distribution: A Comparative Approach with Applications to Climate Related Data
HELIYON(2024)
Key words
Generalized extreme value distributions,Goodness-of-fit statistic,Gumbel distribution,Bi-variate distribution,Moments,Monte Carlo simulation
AI Read Science
Must-Reading Tree
Example
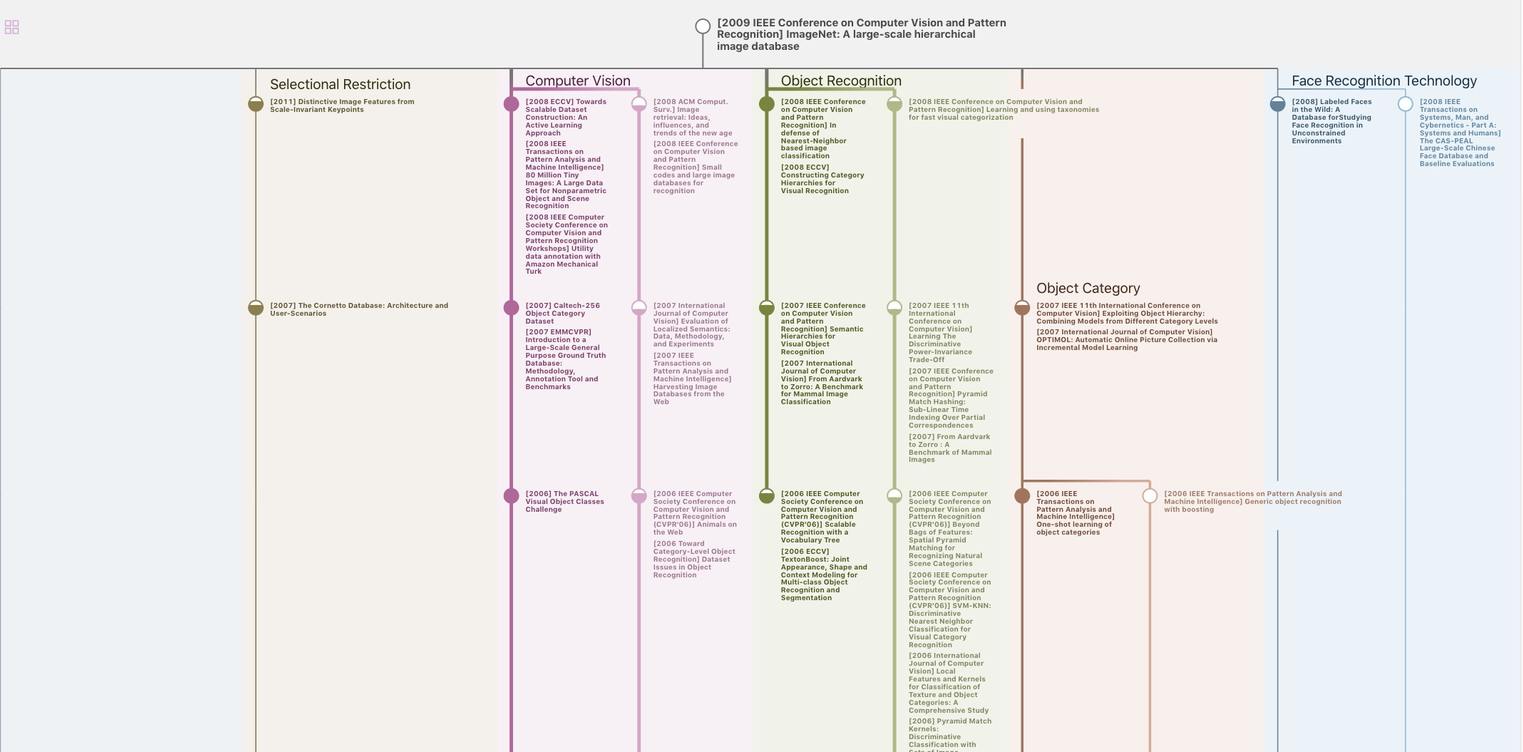
Generate MRT to find the research sequence of this paper
Chat Paper
Summary is being generated by the instructions you defined