Research on Weld Defect Detection of Multi-Scale Normalized Gray-Level Co-Occurrence Matrix
2023 International Conference on Sensing, Measurement & Data Analytics in the era of Artificial Intelligence (ICSMD)(2023)
摘要
Defect detection is a very important link in many manufacturing and processing applications, which can be used for quality control and performance evaluation. However, faced with the industrial environment of weak texture and low contrast, high-precision defect detection still faces challenges due to the diversity and complexity of defects. With the help of feature extractors and classifiers, detection models based on machine learning have shown good detection performance and nonlinear ability in weld defect detection. Nevertheless, there are still some shortcomings, such as traditional gray-level co-occurrence matrix (GLCM) cannot avoid image texture directional suppression and insufficient texture feature extraction. To address these problems, this paper proposes an X-ray weld defect detection system based on multi-scale normalized GLCM (MN-GLCM). The proposed system mainly consists of three modules. In the first module, the weld image is preprocessed through mean filtering and sine function transformation to protect the edges of the weld images and enhance the contrast. Additionally, eleven texture features are extracted through optimized MN-GLCM. Finally, the performance of the proposed system is validated through various classifiers in a self-built datasets and the international public datasets (GDX-ray). The experiments demonstrate that MN-GLCM effectively extract the texture features of weld defects, which improves the recognition accuracy of X-ray weld defect detection system by 0.8% to 12% and reaches a maximum of 100%.
更多查看译文
关键词
X-ray weld defect detection,Texture features,Gray-level co-occurrence matrix
AI 理解论文
溯源树
样例
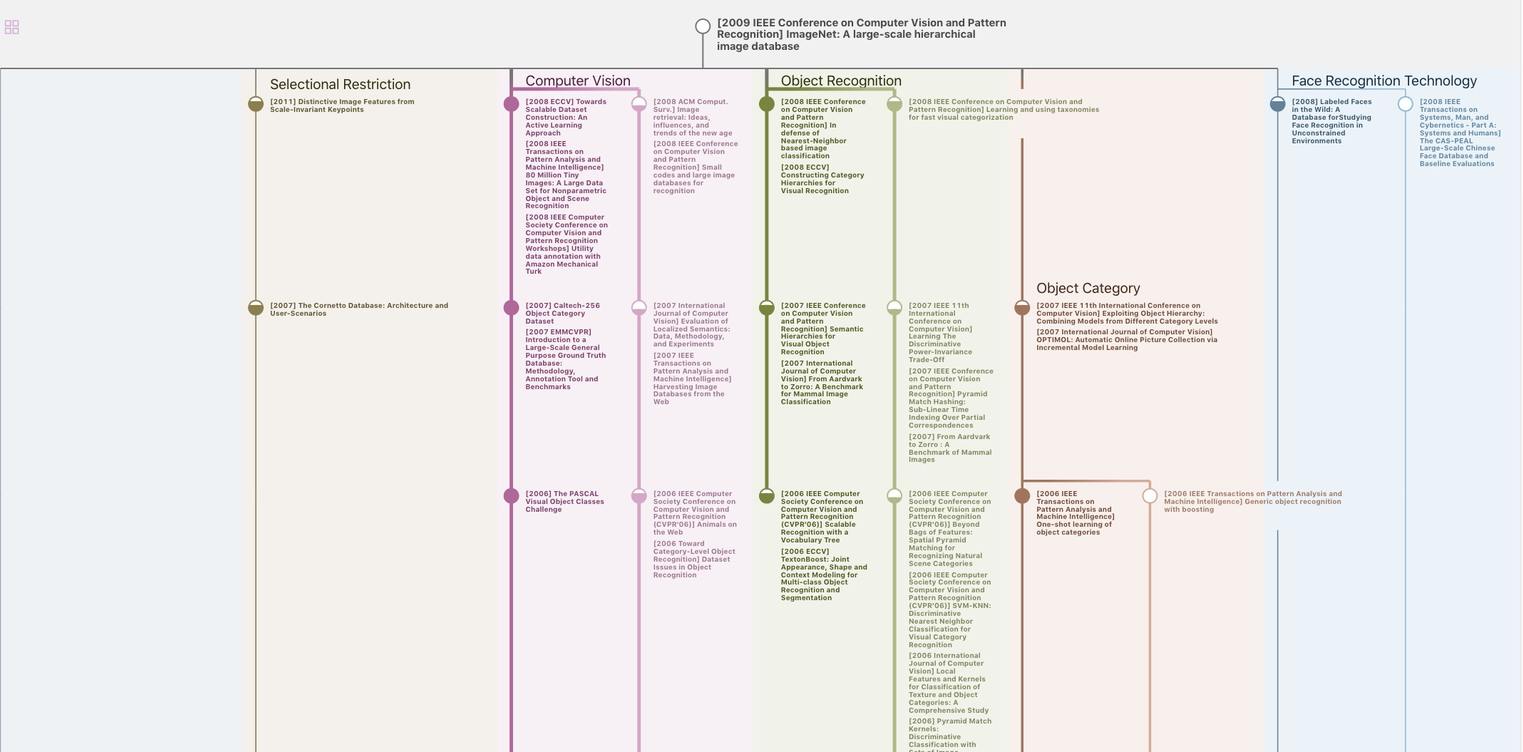
生成溯源树,研究论文发展脉络
Chat Paper
正在生成论文摘要