Prediction of Condition Monitoring Signals Using Scalable Pairwise Gaussian Processes and Bayesian Model Averaging
IEEE Transactions on Automation Science and Engineering(2024)
摘要
Predicting condition monitoring signals has become a critical task for health status assessment and monitoring of industrial systems. It is crucial to incorporate correlated historical data when making predictions for the target signal. As a flexible nonparametric approach, the multi-output Gaussian process (MOGP) model can be employed for this nonlinear regression problem. One effective way to construct MOGP is through convolving a latent function drawn from a Gaussian process. While leveraging the convolution process makes MOGP expressive, there are several challenges that remain to be addressed. First, the scalability of MOGP is always an important issue since the computational demands would increase drastically as the dimension of output variables grows. Besides, the negative transfer should be mitigated when the target variable and the source variable share little commonality. In this study, a pairwise structure is adopted by decomposing the full multivariate model into a group of bi-output models. Furthermore, a Bayesian model averaging approach is utilized to combine the prediction results of the bi-output models. A model selection scheme based on Bayes factor is employed to alleviate negative transfer and facilitate model scalability further by discarding the weakly correlated outputs. The key advantage of the proposed model lies in the improved prediction and uncertainty quantification performance compared with traditional MOGP models. The superiority of the proposed method is validated by numerical studies and a case study.
Note to Practitioners
—This study addresses the challenge of predicting condition monitoring signals in a high-dimensional setting. Existing nonparametric approaches suffer from high computation complexity or ineffective information integration from different signals. We propose a novel approach using a scalable pairwise MOGP model based on Bayesian model averaging. Our method decomposes the full model into bi-output submodels and averages them in a Bayesian way. We also employ a model selection scheme based on the Bayes factor to alleviate negative transfer by discarding weakly correlated outputs. Numerical experiments suggest that this approach can improve prediction accuracy and uncertainty quantification performance for the prediction of condition monitoring signals. Our approach offers a promising solution for condition monitoring signal prediction in automatic and industrial systems where condition monitoring data are readily available.
更多查看译文
关键词
Multi-output Gaussian process,high-dimensional outputs,pairwise models,Bayesian model averaging,model selection
AI 理解论文
溯源树
样例
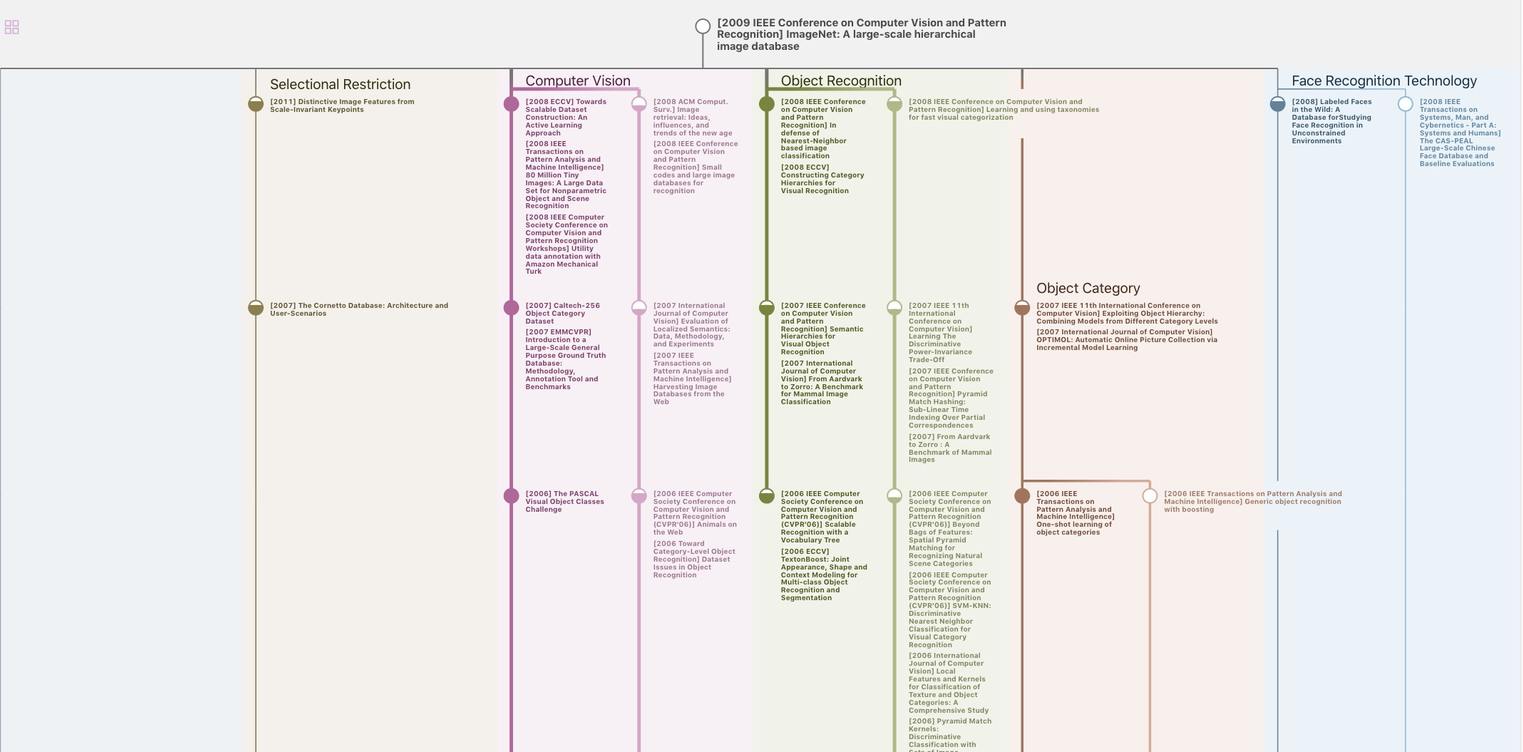
生成溯源树,研究论文发展脉络
Chat Paper
正在生成论文摘要