Improving the Learning Performance of Client’s Local Distribution in Cyclic Federated Learning
Image Analysis & Stereology(2024)
Key words
federated learning,medical image processing,transfer learning
AI Read Science
Must-Reading Tree
Example
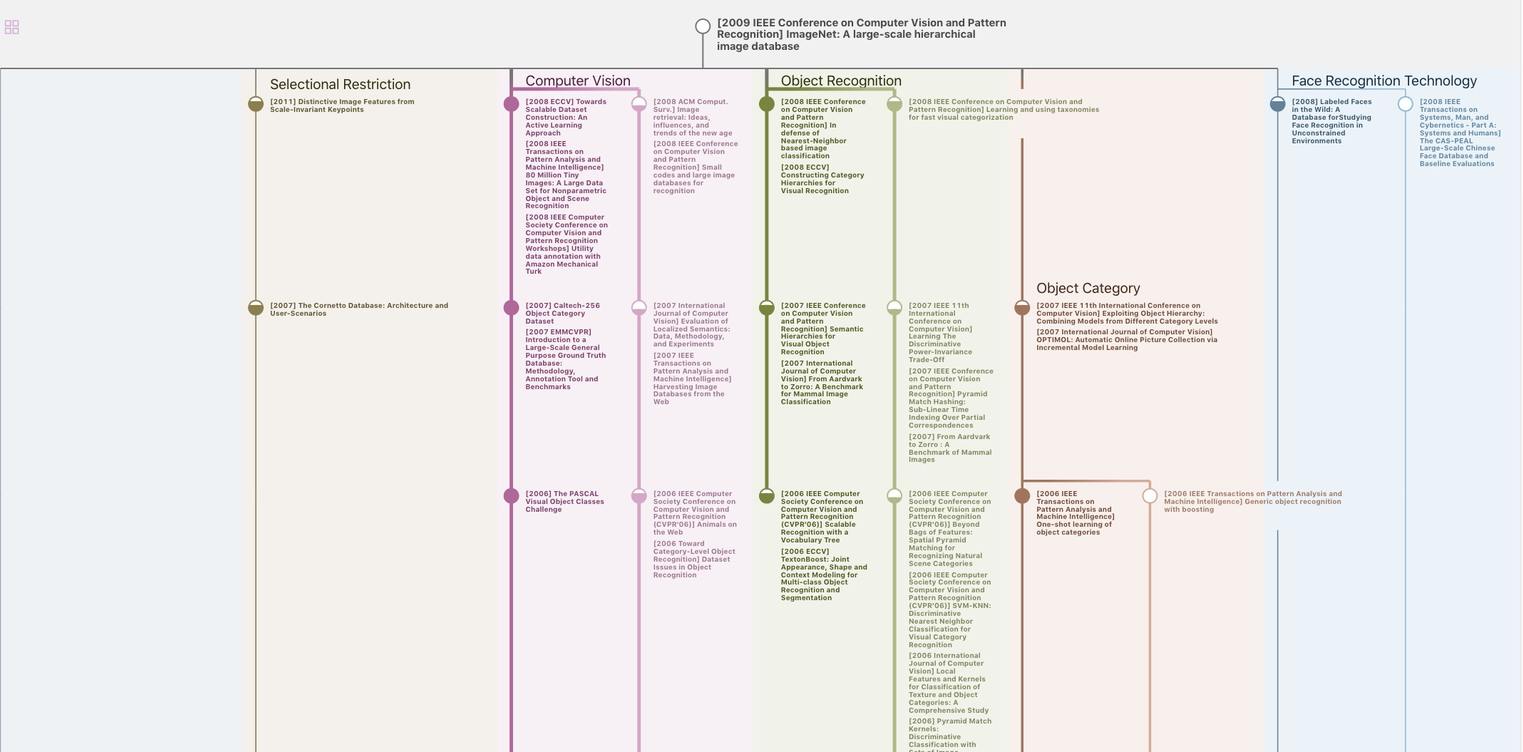
Generate MRT to find the research sequence of this paper
Chat Paper
Summary is being generated by the instructions you defined