Graph-based EEG Approach for Depression Prediction: Integrating Time-Frequency Complexity and Spatial Topology
FRONTIERS IN NEUROSCIENCE(2024)
关键词
EEG signal,depression prediction,graph convolutional network,time-frequency complexity,spatial topology,brain network
AI 理解论文
溯源树
样例
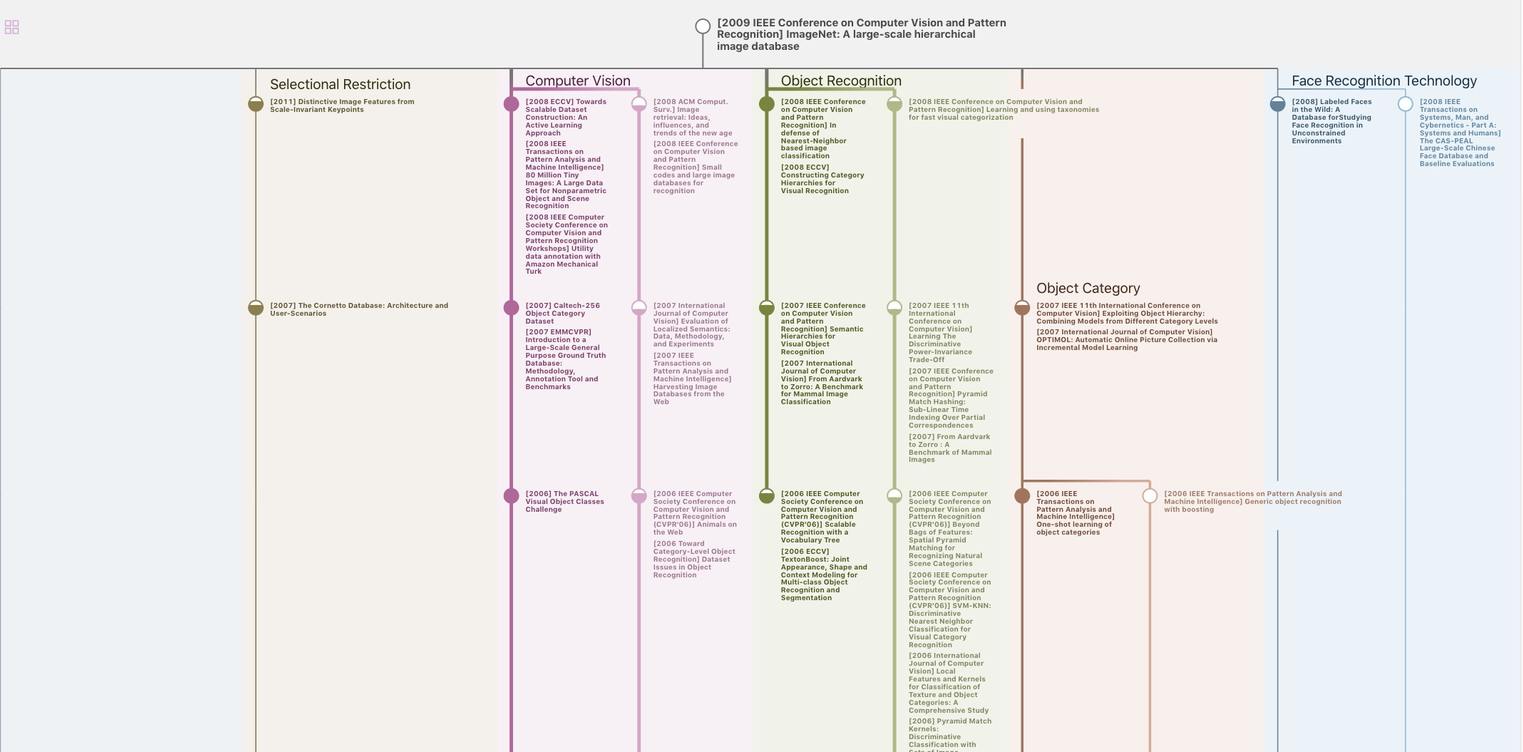
生成溯源树,研究论文发展脉络
Chat Paper
正在生成论文摘要