Machine Learning Aided Optimization of P1 Laser Scribing Process on Indium Tin Oxide Substrates
ADVANCED INTELLIGENT SYSTEMS(2024)
摘要
Present study employes a picosecond laser (532 nm) for selective P1 laser scribing on the indium tin oxide (ITO) layer and subsequent fine-tuning of P1 scribing conditions with machine learning (ML) techniques. Initially, the scribing is performed by varying different laser parameters and further evaluate them via an optical microscope and two probe resistivity measurements. The corresponding scribing width and sheet resistance data are used as input databases for ML analysis. The classification and regression tree (CART) -based ML analysis revealed that median pulse energy <5.7 mu J insufficient to separate the adjacent scribing regions. While pulse energy >5.7 mu J, APL > 35%, LSO > 46%, and processing speed >= 1250 mm s(-1) gives >= 16 mu m of scribing width. Further, the decision tree (DT) analysis showed that pulse energy of >= 8.1 mu J, and LSO >= 37% are required for electrically isolated lines. The feature importance score suggests that laser fluence and pulse energy determined the scribing width, whereas electrical isolation strongly depends on LSO and processing speed. Finally, the ML achieved conditions experimentally validated and reassessed via scanning electron microscope, and atomic force microscopy aligns well with optical microscope measurements.
更多查看译文
关键词
CART,ITO,laser scribing,machine learning,solar module
AI 理解论文
溯源树
样例
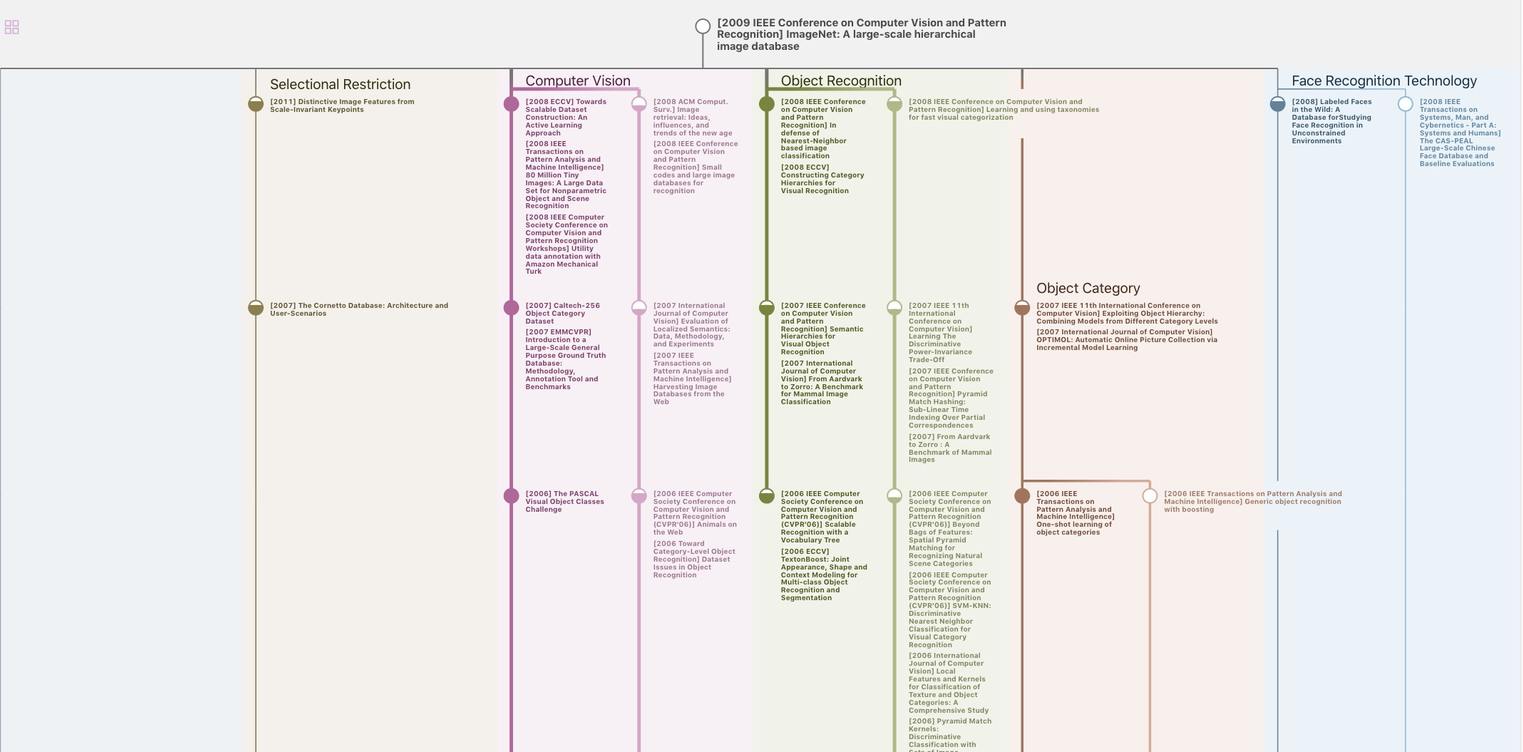
生成溯源树,研究论文发展脉络
Chat Paper
正在生成论文摘要