A novel hybrid model for bridge dynamic early warning using LSTM-EM-GMM
Advances in Bridge Engineering(2024)
摘要
Early warning of existing bridges is now predominated by deterministic methods. However, these methods face challenges in expressing uncertain factors (such as wind load, temperature load, and other variables, etc.). These problems directly impact the timeliness and accuracy of bridge early warning. This study develops an innovative method for bridge dynamic early warning with high versatility and accuracy. Long short-term memory network model (LSTM), expectation maximization (EM) and Gaussian mixture model (GMM) were employed in the proposed method. Firstly, the LSTM model is used to predict the measured monitoring data (such as deflection, strain, cable force, etc.) in real time to obtain the predicted results. Next, the number of clusters for the EM-GMM model is determined using the Calinski-Harabasz (CH) index. The method aims to comprehensively consider the internal cohesion of the clustering, ensuring accurate and reliable clustering results. Then, the EM-GMM model is used to cluster the random influence error and the predicted value, which can get the probabilistic prediction result of each corresponding random influence error. On this basis, the dynamic early warning interval under 95
更多查看译文
关键词
Bridge,Structural health monitoring,Probabilistic method,Dynamic early warning,Uncertain factors
AI 理解论文
溯源树
样例
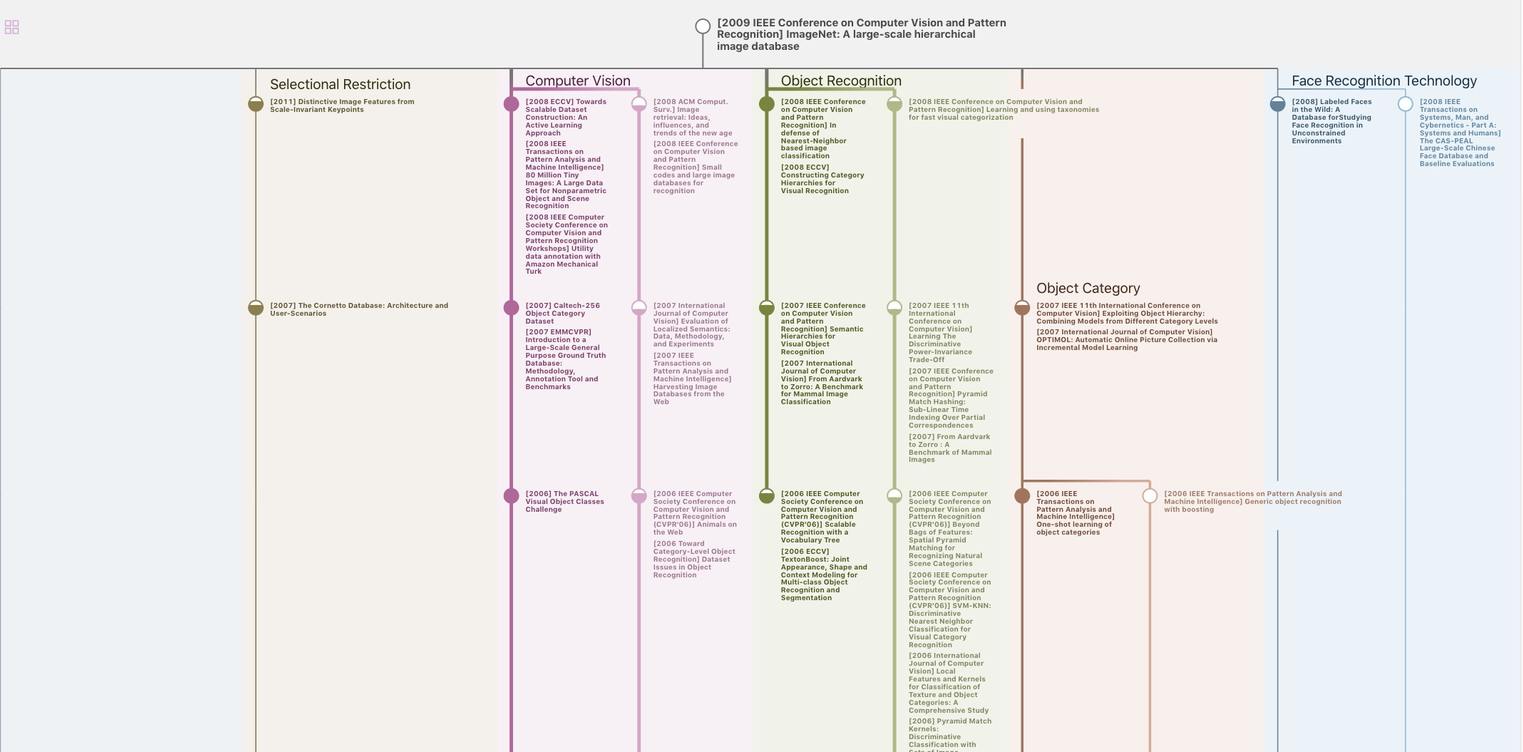
生成溯源树,研究论文发展脉络
Chat Paper
正在生成论文摘要