Targeting protein-ligand neosurfaces using a generalizable deep learning approach
biorxiv(2024)
摘要
Molecular recognition events between proteins drive biological processes in living systems. However, higher levels of mechanistic regulation have emerged, where protein-protein interactions are conditioned to small molecules. Here, we present a computational strategy for the design of proteins that target neosurfaces, i.e. surfaces arising from protein-ligand complexes. To do so, we leveraged a deep learning approach based on learned molecular surface representations and experimentally validated binders against three drug-bound protein complexes. Remarkably, surface fingerprints trained only on proteins can be applied to neosurfaces emerging from small molecules, serving as a powerful demonstration of generalizability that is uncommon in deep learning approaches. The designed chemically-induced protein interactions hold the potential to expand the sensing repertoire and the assembly of new synthetic pathways in engineered cells.
### Competing Interest Statement
Ecole Polytechnique Federale de Lausanne (EPFL) has filed a patent application that incorporates findings presented previously in MaSIF-seed. P.G., A.M., M.B. and B.E.C. are named as co-inventors on this patent (US Patent Office, US20230395187A1).
更多查看译文
AI 理解论文
溯源树
样例
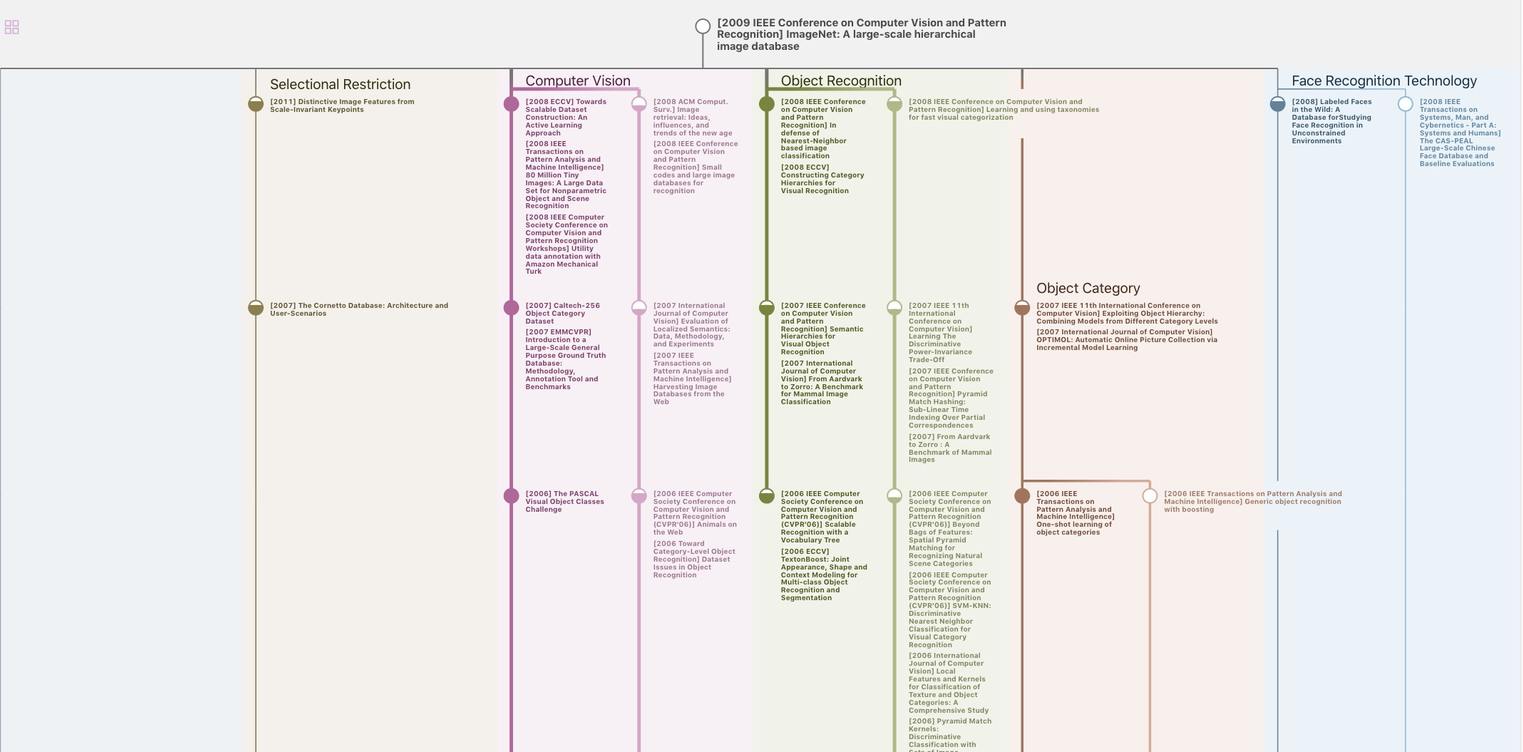
生成溯源树,研究论文发展脉络
Chat Paper
正在生成论文摘要