N-MlpE: Optimizing Multilayer Perceptron Network-based Knowledge Graph Embedding Model with Neighborhood Information
International Conference on Parallel and Distributed Systems(2023)
Key words
knowledge graph completion,knowledge graph embedding,link prediction,distributed representation inference
AI Read Science
Must-Reading Tree
Example
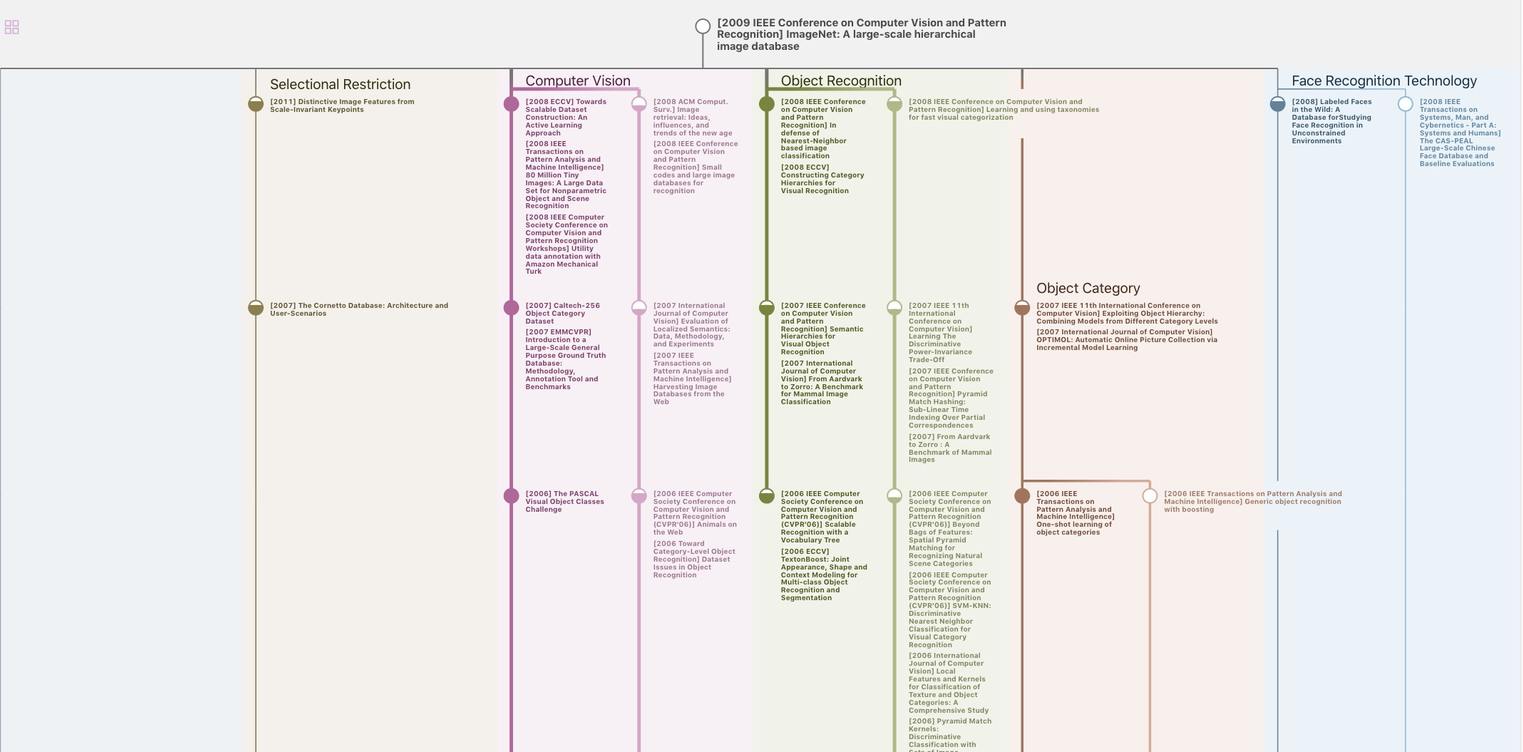
Generate MRT to find the research sequence of this paper
Chat Paper
Summary is being generated by the instructions you defined