Amodal Scene Analysis via Holistic Occlusion Relation Inference and Generative Mask Completion
AAAI 2024(2024)
摘要
Amodal scene analysis entails interpreting the occlusion relationship among scene elements and inferring the possible shapes of the invisible parts. Existing methods typically frame this task as an extended instance segmentation or a pair-wise object de-occlusion problem. In this work, we propose a new framework, which comprises a Holistic Occlusion Relation Inference (HORI) module followed by an instance-level Generative Mask Completion (GMC) module.
Unlike previous approaches, which rely on mask completion results for occlusion reasoning, our HORI module directly predicts an occlusion relation matrix in a single pass. This approach is much more efficient than the pair-wise de-occlusion process and it naturally handles mutual occlusion, a common but often neglected situation.
Moreover, we formulate the mask completion task as a generative process and use a diffusion-based GMC module for instance-level mask completion. This improves mask completion quality and provides multiple plausible solutions.
We further introduce a large-scale amodal segmentation dataset with high-quality human annotations, including mutual occlusions. Experiments on our dataset and two public benchmarks demonstrate the advantages of our method. code public available at https://github.com/zbwxp/Amodal-AAAI.
更多查看译文
关键词
CV: Segmentation,CV: Scene Analysis & Understanding
AI 理解论文
溯源树
样例
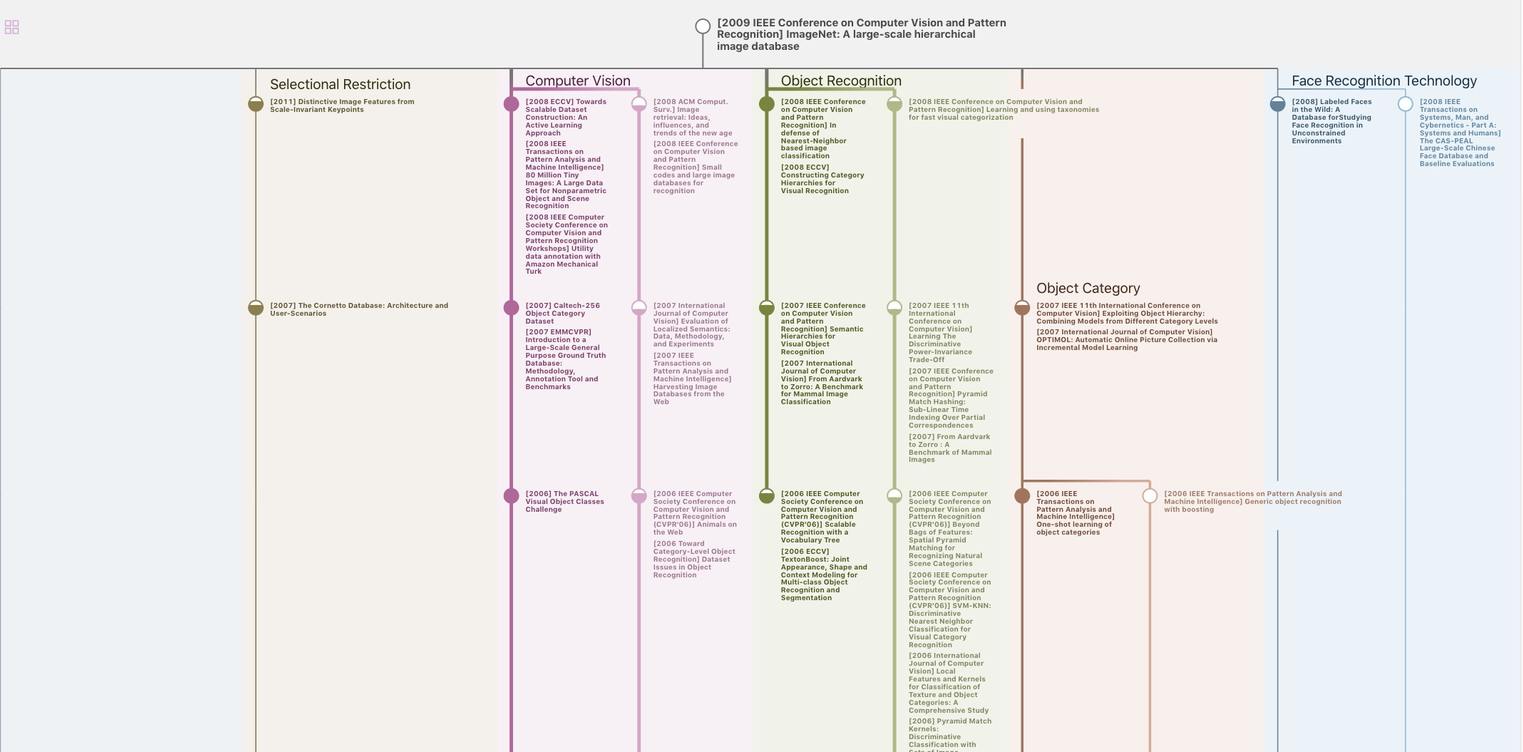
生成溯源树,研究论文发展脉络
Chat Paper
正在生成论文摘要