Predicting Intubation for Intensive Care Units Patients: A Deep Learning Approach to Improve Patient Management
International Journal of Medical Informatics(2024)
Key words
Deep learning,Time series,Intubation prediction,Intensive care unit
AI Read Science
Must-Reading Tree
Example
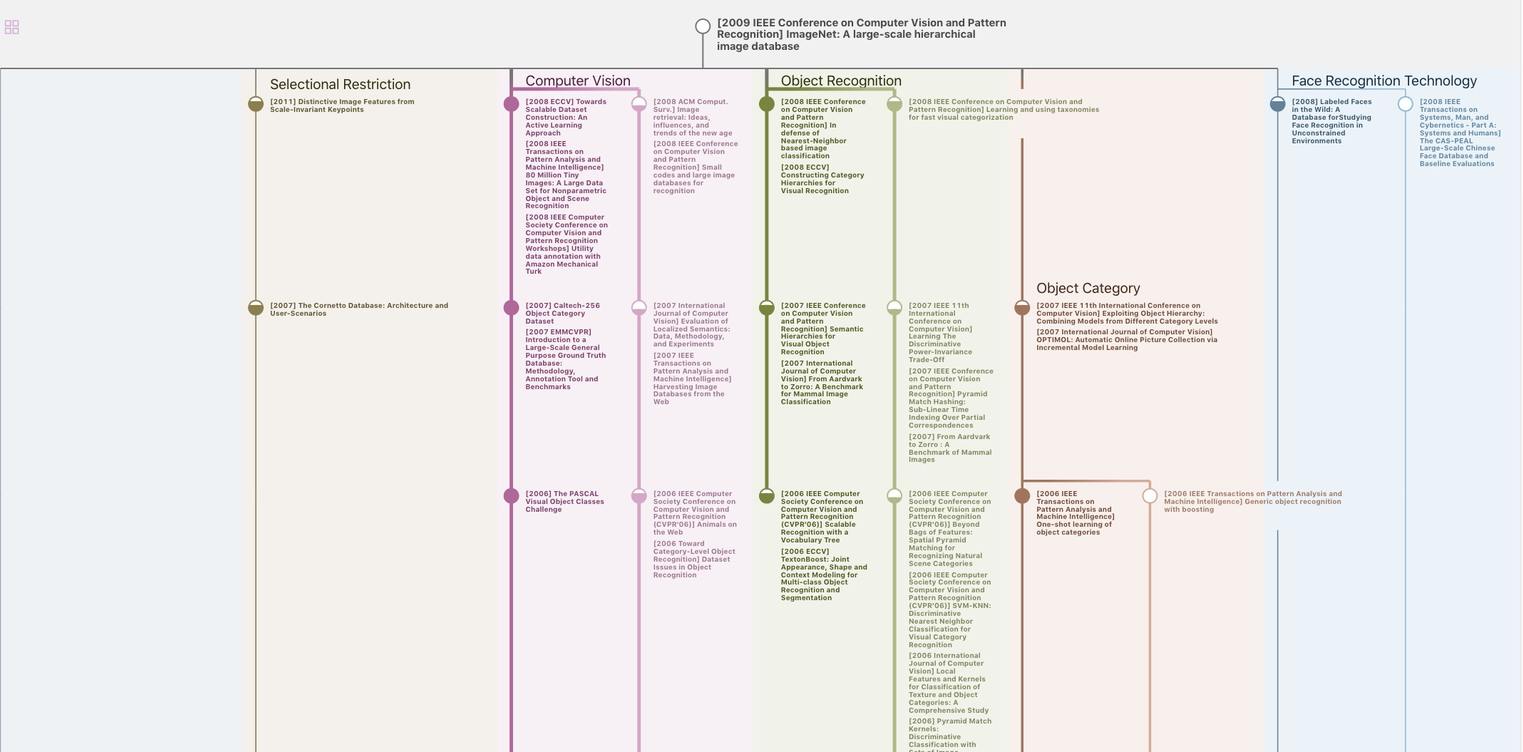
Generate MRT to find the research sequence of this paper
Chat Paper
Summary is being generated by the instructions you defined