Constantly optimized mean teacher for semi-supervised 3D MRI image segmentation
Medical & Biological Engineering & Computing(2024)
摘要
The mean teacher model and its variants, as important methods in semi-supervised learning, have demonstrated promising performance in magnetic resonance imaging (MRI) data segmentation. However, the superior performance of teacher model through exponential moving average (EMA) is limited by the unreliability of unlabeled image, resulting in potentially unreliable predictions. In this paper, we propose a framework to optimized the teacher model with reliable expert-annotated data while preserving the advantages of EMA. To avoid the tight coupling that results from EMA, we leverage data augmentations to provide two distinct perspectives for the teacher and student models. The teacher model adopts weak data augmentation to provide supervision for the student model and optimizes itself with real annotations, while the student uses strong data augmentation to avoid overfitting on noise information. In addition, double softmax helps the model resist noise and continue learning meaningful information from the images, which is a key component in the proposed model. Extensive experiments show that the proposed method exhibits competitive performance on the Left Atrium segmentation MRI dataset (LA) and the Brain Tumor Segmentation MRI dataset (BraTS2019). For the LA dataset, we achieved a dice of 91.02% using only 20% labeled data, which is close to the dice of 91.14% obtained by the supervised approach using 100% labeled data. For the BraTs2019 dataset, the proposed method achieved 1.02% and 1.92% improvement on 5% and 10% labeled data, respectively, compared to the best baseline method on this dataset. This study demonstrates that the proposed model can be a potential candidate for medical image segmentation in semi-supervised learning scenario. Graphical abstract
更多查看译文
关键词
Semi-supervised learning,Data augmentation,Medical image segmentation,Mean teacher
AI 理解论文
溯源树
样例
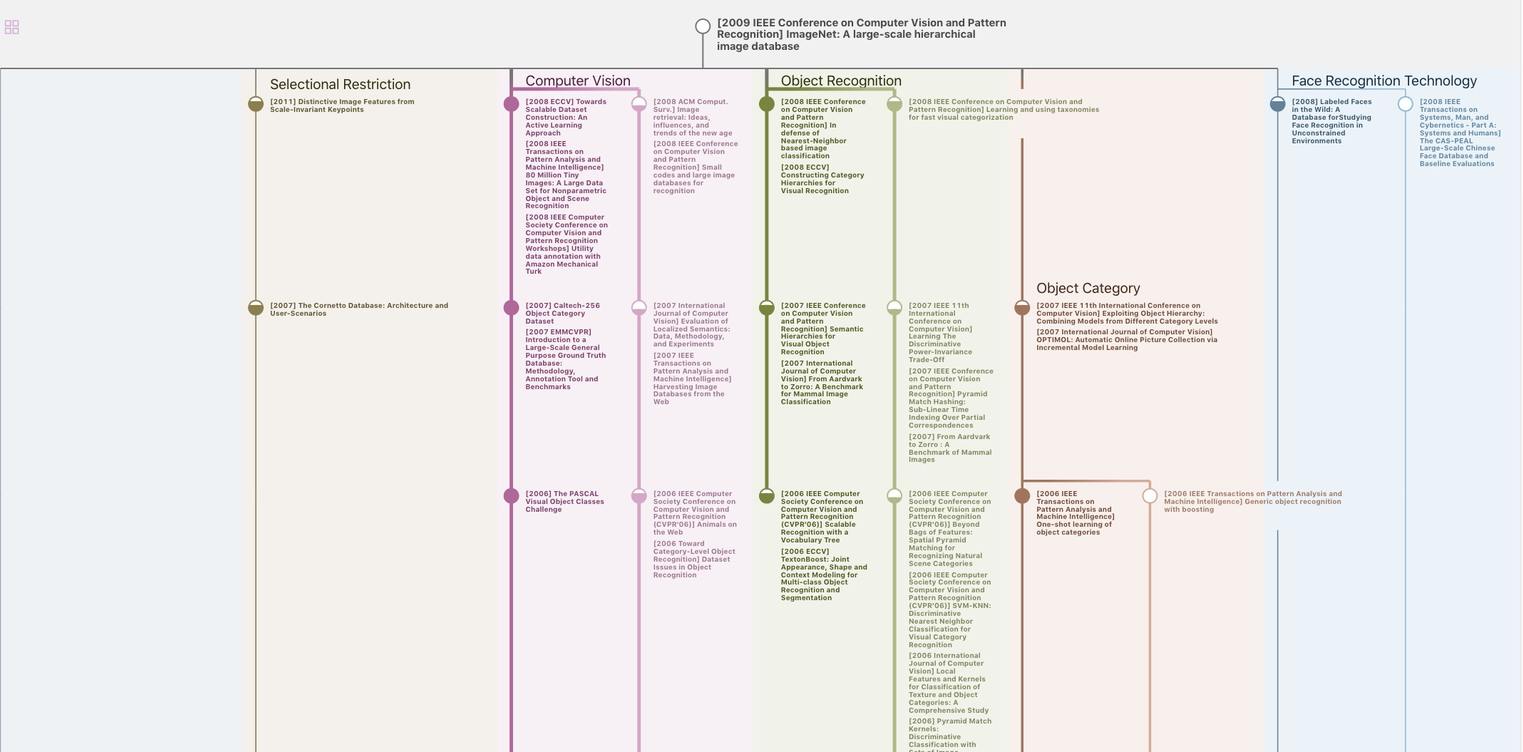
生成溯源树,研究论文发展脉络
Chat Paper
正在生成论文摘要