Considerations in the use of ML interaction potentials for free energy calculations
CoRR(2024)
摘要
Machine learning potentials (MLPs) offer the potential to accurately model
the energy and free energy landscapes of molecules with the precision of
quantum mechanics and an efficiency similar to classical simulations. This
research focuses on using equivariant graph neural networks MLPs due to their
proven effectiveness in modeling equilibrium molecular trajectories. A key
issue addressed is the capability of MLPs to accurately predict free energies
and transition states by considering both the energy and the diversity of
molecular configurations. We examined how the distribution of collective
variables (CVs) in the training data affects MLP accuracy in determining the
free energy surface (FES) of systems, using Metadynamics simulations for butane
and alanine dipeptide (ADP). The study involved training forty-three MLPs, half
based on classical molecular dynamics data and the rest on ab initio computed
energies. The MLPs were trained using different distributions that aim to
replicate hypothetical scenarios of sampled CVs obtained if the underlying FES
of the system was unknown. Findings for butane revealed that training data
coverage of key FES regions ensures model accuracy regardless of CV
distribution. However, missing significant FES regions led to correct potential
energy predictions but failed free energy reconstruction. For ADP, models
trained on classical dynamics data were notably less accurate, while ab
initio-based MLPs predicted potential energy well but faltered on free energy
predictions. These results emphasize the challenge of assembling an
all-encompassing training set for accurate FES prediction and highlight the
importance of understanding the FES in preparing training data. The study
points out the limitations of MLPs in free energy calculations, stressing the
need for comprehensive data that encompasses the system's full FES for
effective model training.
更多查看译文
AI 理解论文
溯源树
样例
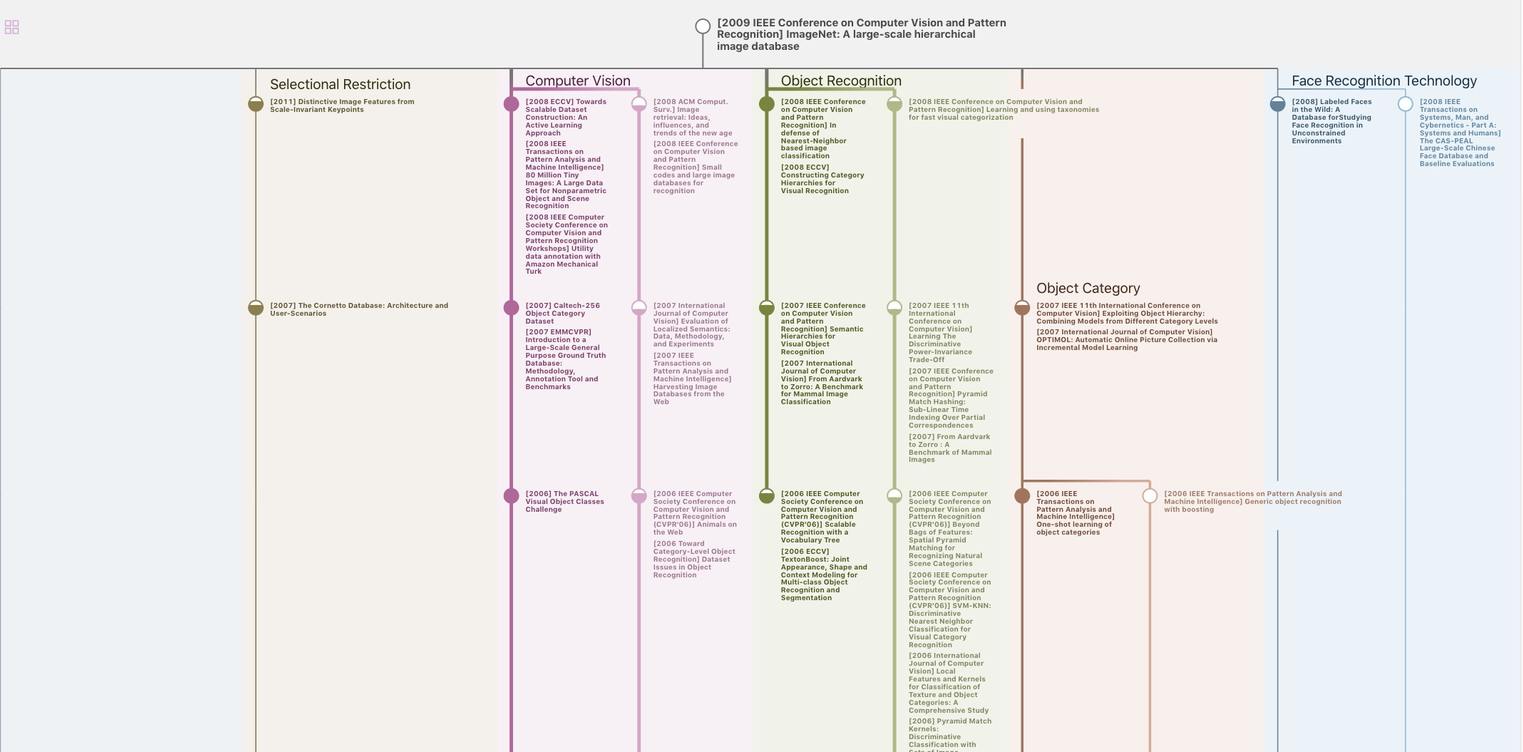
生成溯源树,研究论文发展脉络
Chat Paper
正在生成论文摘要