Physically Informed Graph-Based Deep Reasoning Net for Efficient Combinatorial Phase Mapping
International Conference on Machine Learning and Applications(2023)
关键词
phase mapping,unsupervised pattern demixing,physically informed decoding,graph-based smoothness
AI 理解论文
溯源树
样例
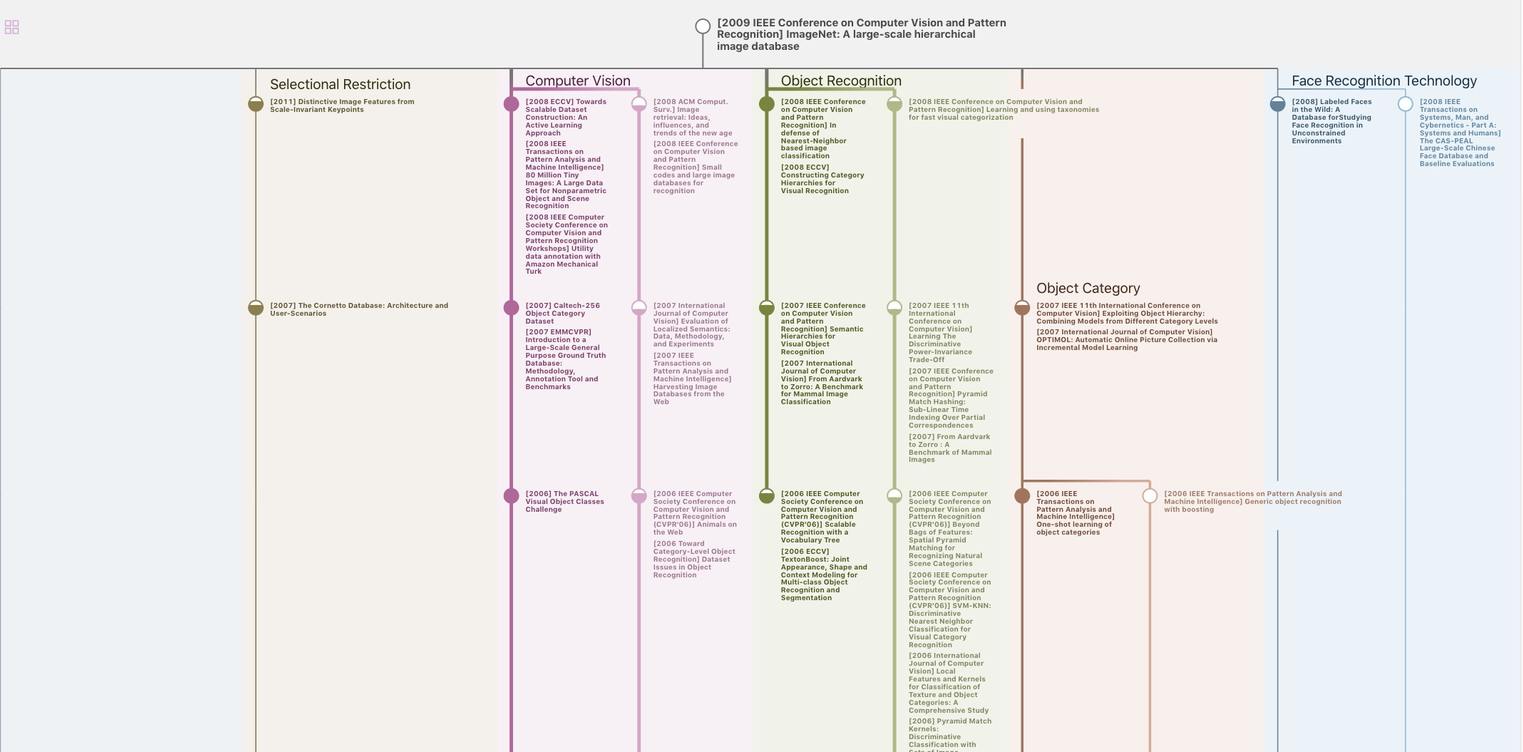
生成溯源树,研究论文发展脉络
Chat Paper
正在生成论文摘要