A Mixture-Gas Edge-Computing Multi-Sensor Device with Generative Learning Framework
IEEE Sensors Journal(2024)
摘要
This paper presents a mixture-gas detectable edge-computing device with a generative learning framework for selectivity and accuracy. Mixture-gas detection capability is enabled through two proposed schemes of temperature modulation and cross-iterative-tuning artificial neural network (CIT-ANN). Their related computations are facilitated inside the edge device level, applying analog normalization concepts in the readout integrated circuit (ROIC). This proposed edge platform provides generative training data for mixture-gas detection, allowing much less empirical data for its learning process, especially under mixture gas environment. An edge-computing IoT device prototype was manufactured based on a fabricated ROIC and in-house metal-oxide-semiconductor sensor arrays embedding heater modulation function. Under mixture-gas experiments of NO
2
and CO gases, the proposed CIT-ANN together with the heater modulation demonstrated 44% higher recognition performance than in the conventional ANN. The proposed generative learning method showed higher relative label coincidence, achieving 17% higher correlation with real training data than in the conventional mathematical interpolation method.
更多查看译文
关键词
Mixture Gas Sensor,Readout Integrated Circuit,Edge Computing,Cross-Iterative-Tuning Artificial Neural Network,Generative Adversarial Networks,Metal-Oxide Semiconductor
AI 理解论文
溯源树
样例
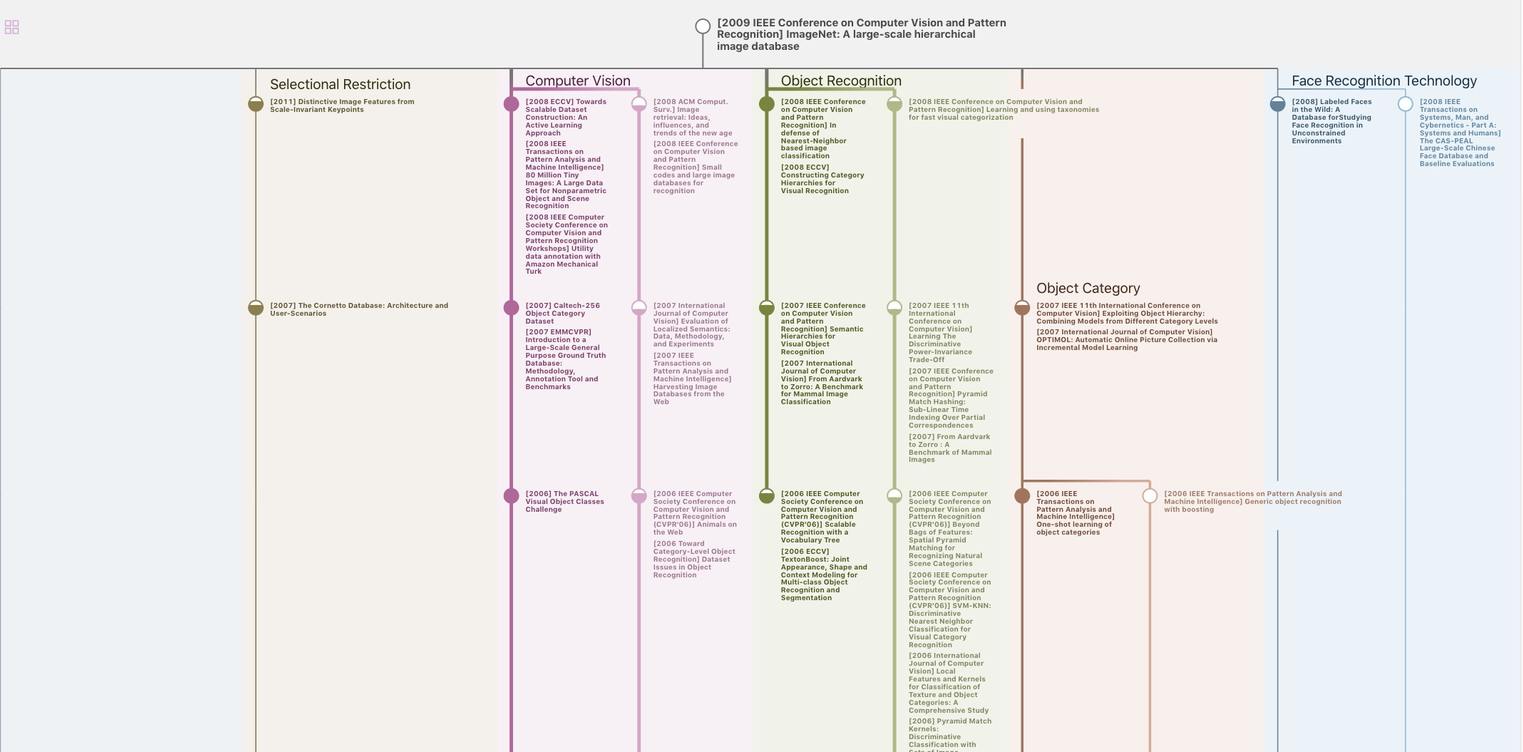
生成溯源树,研究论文发展脉络
Chat Paper
正在生成论文摘要