AI model to improve the mountain boundary layer height of ERA5
Atmospheric Research(2024)
摘要
The mountain boundary layer height (MBLH) plays a crucial role in determining the exchange of heat, momentum, and composition between the low atmosphere and free troposphere. To obtain accurate quantities of the MBLH, we propose a Data Reinforced Ensemble Machine Learning Model (DREM) to estimate the MBLH using both ERA5 reanalysis multi-variables and thermodynamic profiles observed in two mountainous stations of the foot of Qinling Mountain and the peak of Damaojian mountain in southeast China (Shanghuang site). The observations reveal that the MBLH has resemblances characteristics over the foot of mountains or over the top, featuring daytime high convective boundary layer over 2 km and low stable boundary layer at night about 200 m. But the boundary layer height of EAR5 (ERA5-BLH) has very large error, the Mean Absolute Error (MAE) are nearly 500 m at the valley. The errors between DREM and observations are estimated to be 43% at the peak and 22% at the valley, whereas the corresponding errors from ERA5 reanalysis data are 183% and 70%, respectively. In comparison with the baseline models such as Random Forest (RF), Adaboost, and EM, our statistical results based on all samples demonstrate that DREM can improve the error by 10 m. Subsequent analysis of the Shapley Additive Explanations (SHAP) method demonstrates that the contributions of various meteorological variables in MBLH estimation vary greatly. Among them, Surface Thermal Radiation Downwards (STRD) dominates at both the peak and valley, with lower STRD leading to higher accuracy and vice versa. However, the valley MBLH estimation is not solely determined by thermal factors, but also controlled by dynamic factors and Relative Humidity (RH). Case studies indicate that DREM can significantly minimize deviations, especially at the mountain peak, where it can even capture the abrupt variation of MBLH. In conclusion, the study presents a precise and robust method for estimating the MBLH over complex terrain, which provides an invaluable asset for improving the mountainous weather forecasting, as well as deepening the understanding of the environmental and climatic effects of mountain ecosystems.
更多查看译文
关键词
Mountain boundary layer height,Complex terrains,Data reinforced ensemble model,ERA5 reanalysis data,Shapley additive explanations
AI 理解论文
溯源树
样例
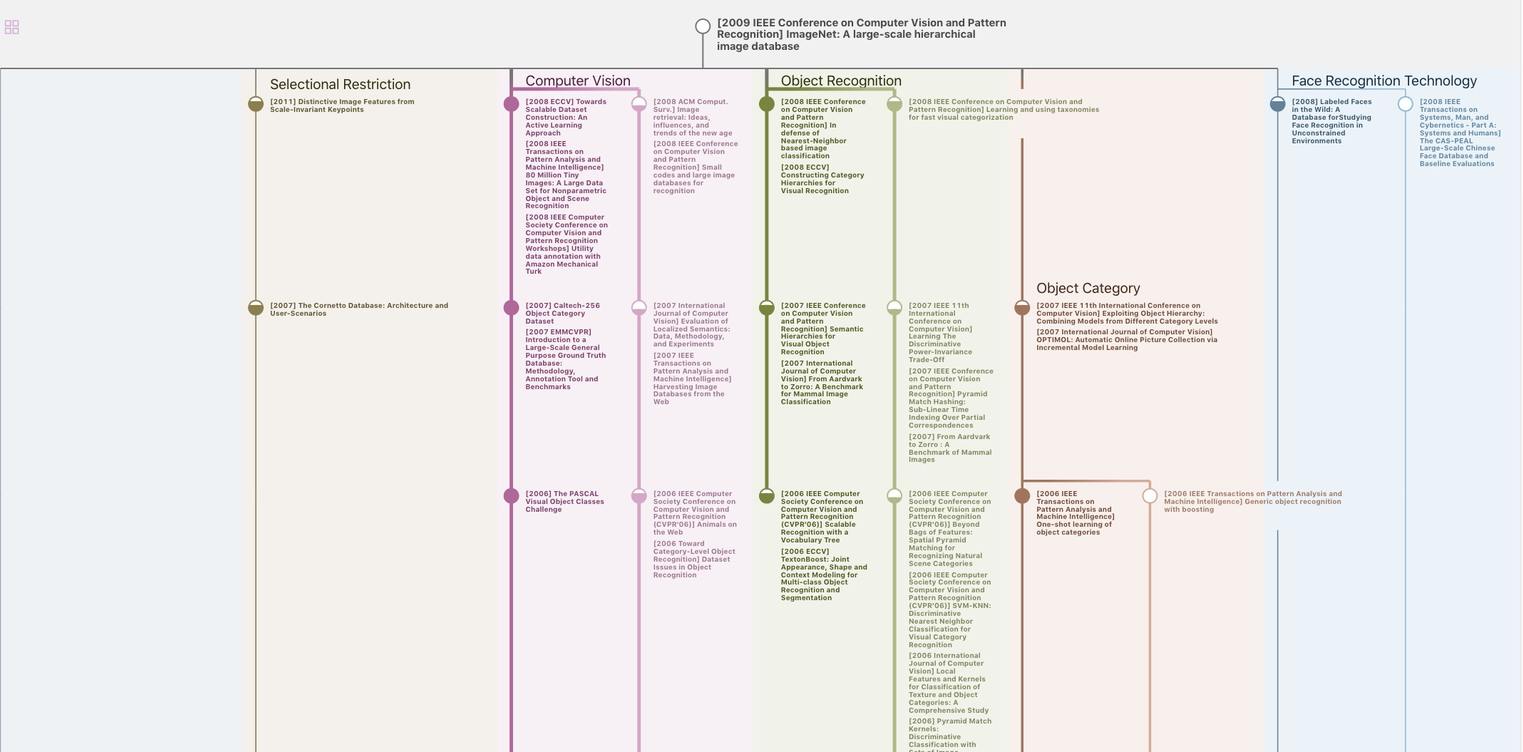
生成溯源树,研究论文发展脉络
Chat Paper
正在生成论文摘要