On Fault-Tolerant Microarchitectural Techniques for Voltage Underscaling in On-Chip Memories of CNN Accelerators
2023 26TH EUROMICRO CONFERENCE ON DIGITAL SYSTEM DESIGN, DSD 2023(2023)
摘要
Aggressively underscaling the supply voltage (V-dd) below the safe voltage (V-min) margin is an effective solution to attain substantial energy savings. Unfortunately, operating at such low voltages is challenging due to the high number of permanent faults as a result of variations in the manufacturing process of current technology nodes. This work characterizes the impact of permanent faults on the accuracy of a Convolutional Neural Network (CNN) inference accelerator with on-chip activation memories supplied at low V-dd below Vmin. Based on these observations, this paper proposes a couple of low-cost microarchitectural techniques, referred to as flipping and patching, that ensure the accuracy of CNN applications despite the presence of permanent faults. Contrary to prior work, the proposed techniques are transparent to the programmer and do not depend on application characteristics. Experimental results show that the proposed techniques maintain the original CNN accuracy with a minimal impact on system performance (less than 0.05%), while reducing the energy consumption of activation memories by 11.2% and 46.7% compared to those of a conventional accelerator operating at safe and nominal supply voltages, respectively.
更多查看译文
关键词
Deep learning,energy efficiency,network accuracy,permanent faults
AI 理解论文
溯源树
样例
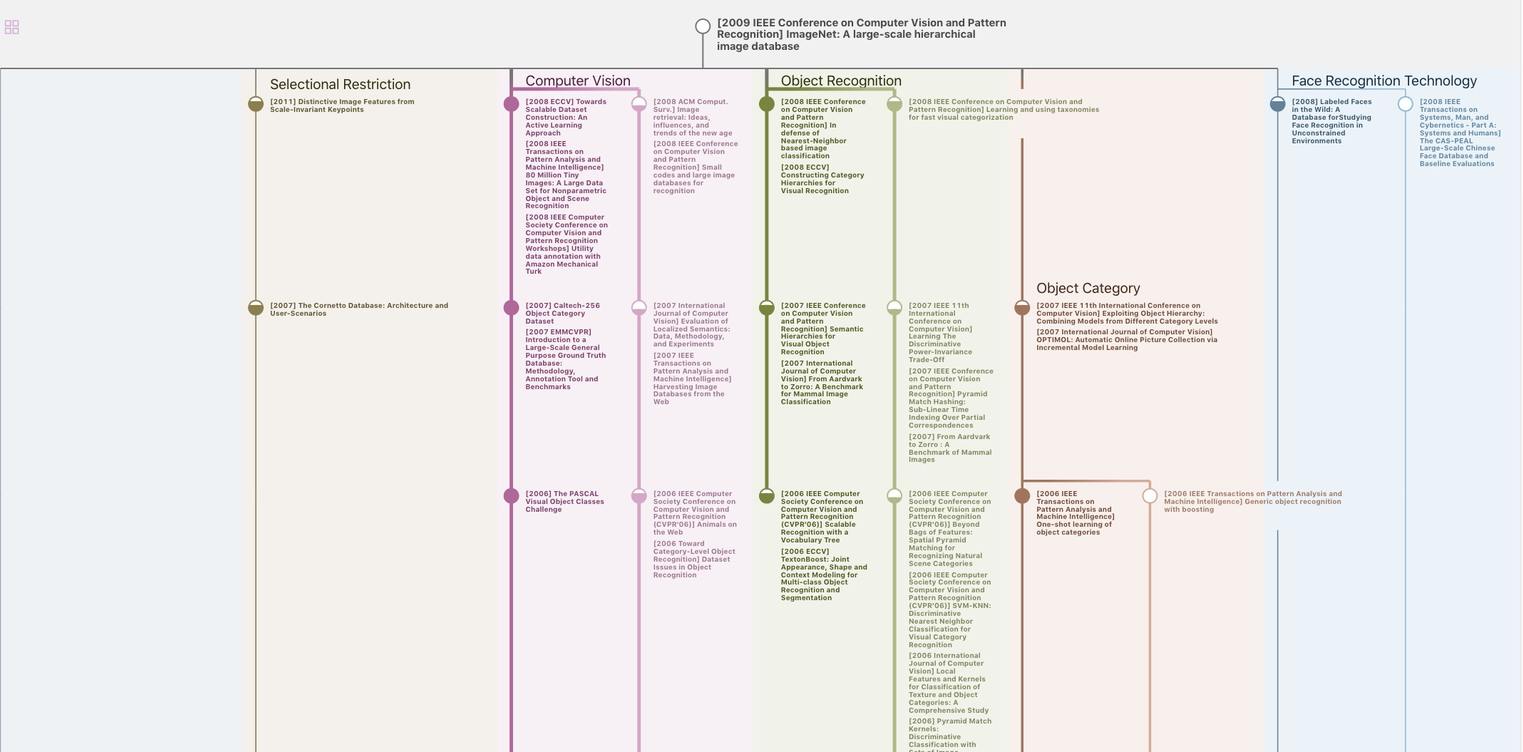
生成溯源树,研究论文发展脉络
Chat Paper
正在生成论文摘要