Efficient Learning on Successive Test Time Augmentation
IEEE International Conference on Acoustics, Speech, and Signal Processing(2024)
Key words
Test time augmentation,Corruption,Robustness,Recurrent neural network
AI Read Science
Must-Reading Tree
Example
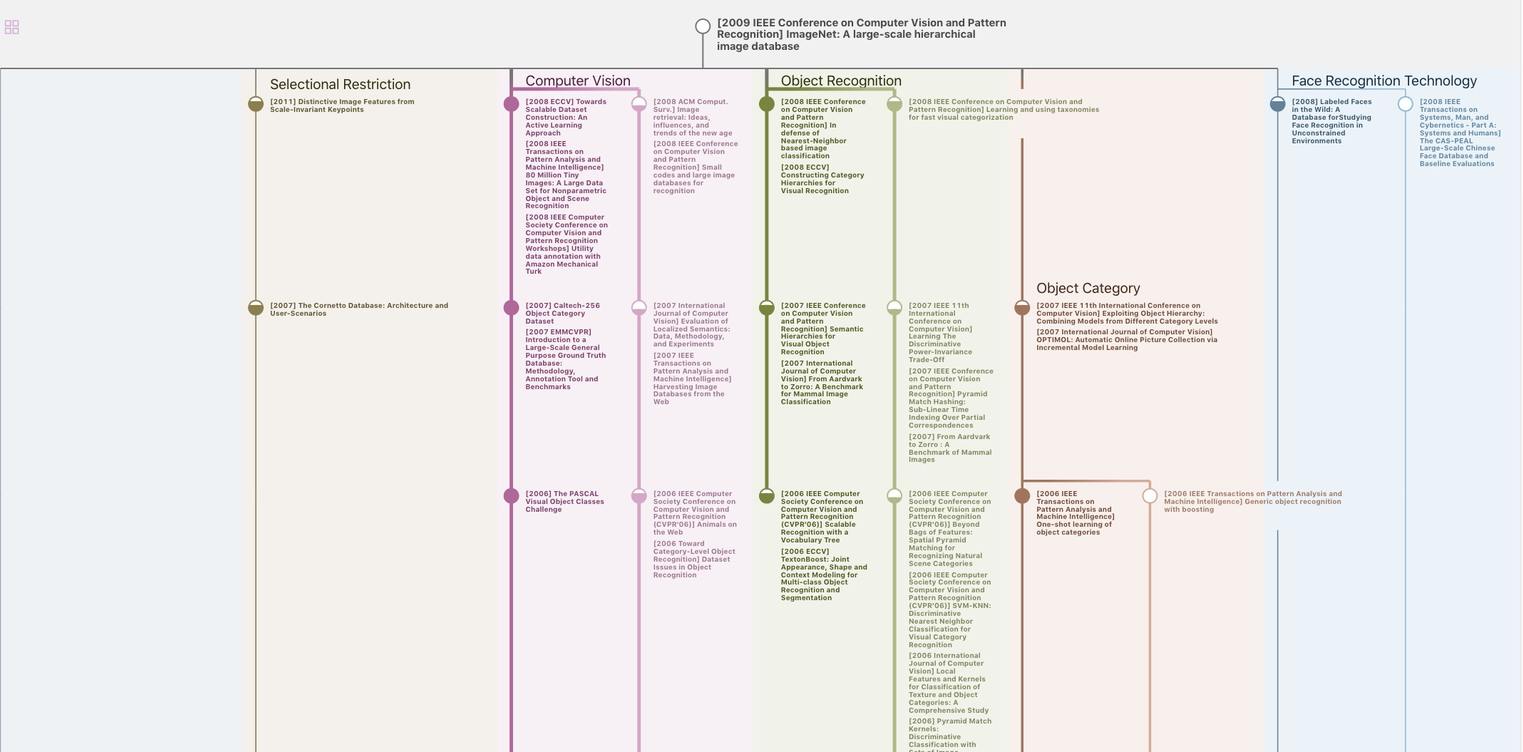
Generate MRT to find the research sequence of this paper
Chat Paper
Summary is being generated by the instructions you defined