High-Accuracy Anxiety Disorder Identification Through Subspace-Enhanced Hypergraph Neural Network
IEEE International Conference on Acoustics, Speech, and Signal Processing(2024)
Key words
AD classification,hypergraph neural network,multimodal data,subspace enhancement
AI Read Science
Must-Reading Tree
Example
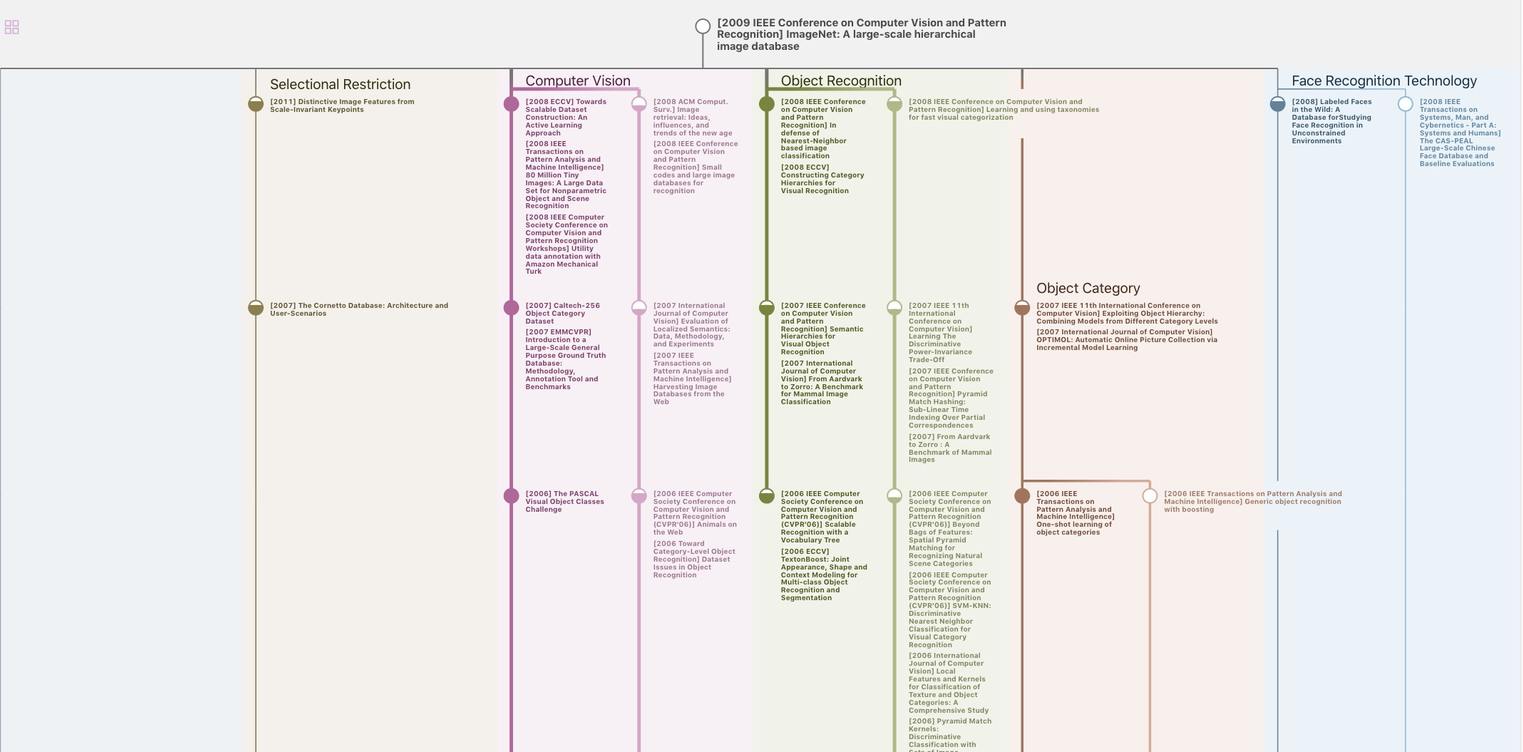
Generate MRT to find the research sequence of this paper
Chat Paper
Summary is being generated by the instructions you defined