Adaptive Drop Approaches to Train Spiking-YOLO Network for Traffic Flow Counting
crossref(2024)
摘要
Abstract Traffic flow counting is an object detection problem. YOLO (" You Only Look Once ") is a popular object detection network. Spiking-YOLO converts the YOLO network from ANN(artificial neural network) to SNN (spiking neural network), which consumes less power than YOLO. However, Spiking-YOLO is difficult to train and has poor accuracy because of overfitting. Dropout and DropConnect are two methods to regularize neural network models and have produced cutting-edge outcomes in many benchmarks. The two drop approaches are first applied to Spiking-YOLO. Considering that a higher membrane potential of a biological neuron implies a higher probability of neural activation, we propose three adaptive drop algorithms—Spiking-YOLO with adaptive Dropout, Spiking-YOLO with adaptive DropConnect, and Spiking-YOLO with group adaptive Drop, which adaptively adjusts the keep probability for training SNNs. The experimental results show that the adaptive algorithms have fast convergence speed with high detection accuracy and mAP value, and work well in the case of high-speed streaming video, making them suitable for online learning scenarios.
更多查看译文
AI 理解论文
溯源树
样例
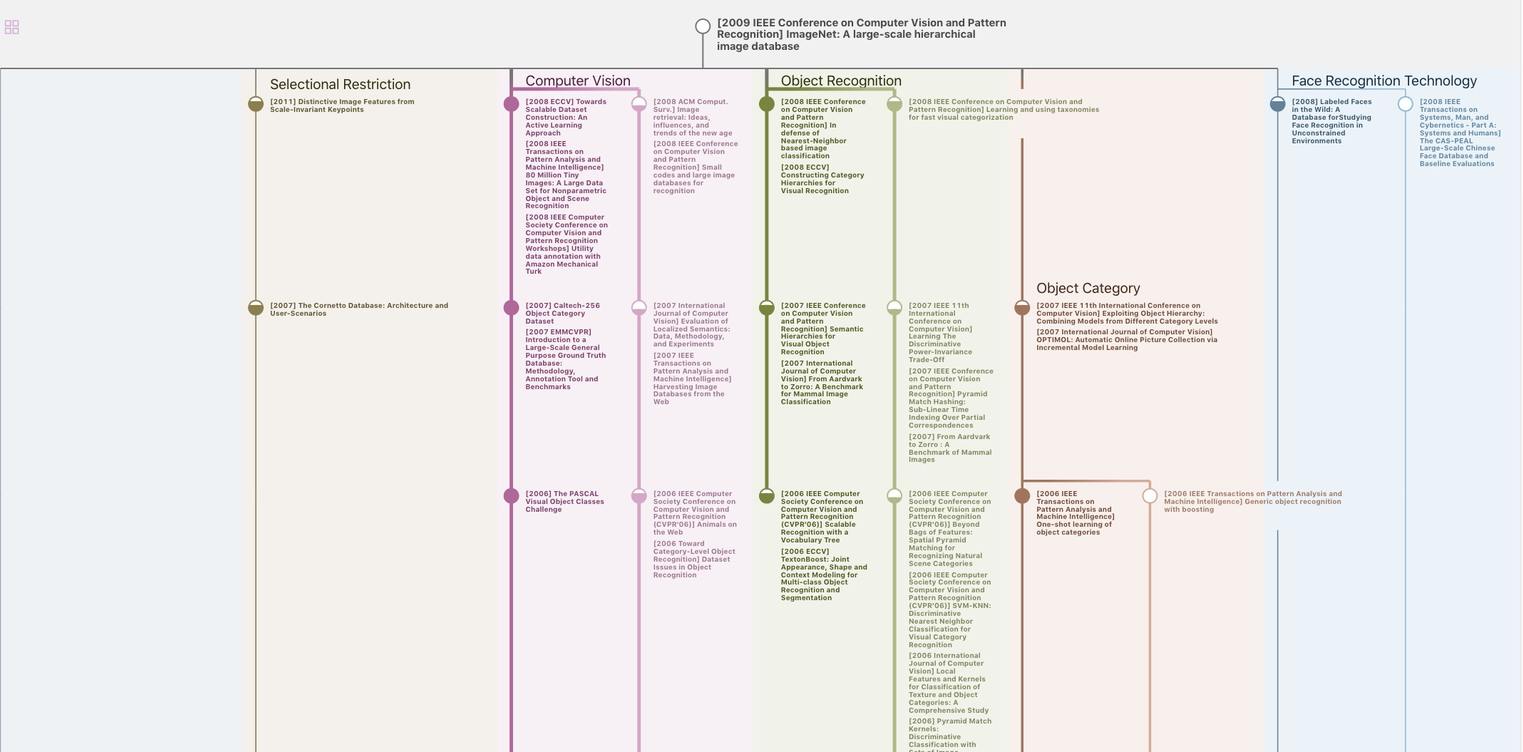
生成溯源树,研究论文发展脉络
Chat Paper
正在生成论文摘要