Similarity Knowledge Distillation with Calibrated Mask
IEEE International Conference on Acoustics, Speech, and Signal Processing(2024)
Key words
Computer Vision,Deep Learning,Classification,Model Compression,Knowledge Distillation
AI Read Science
Must-Reading Tree
Example
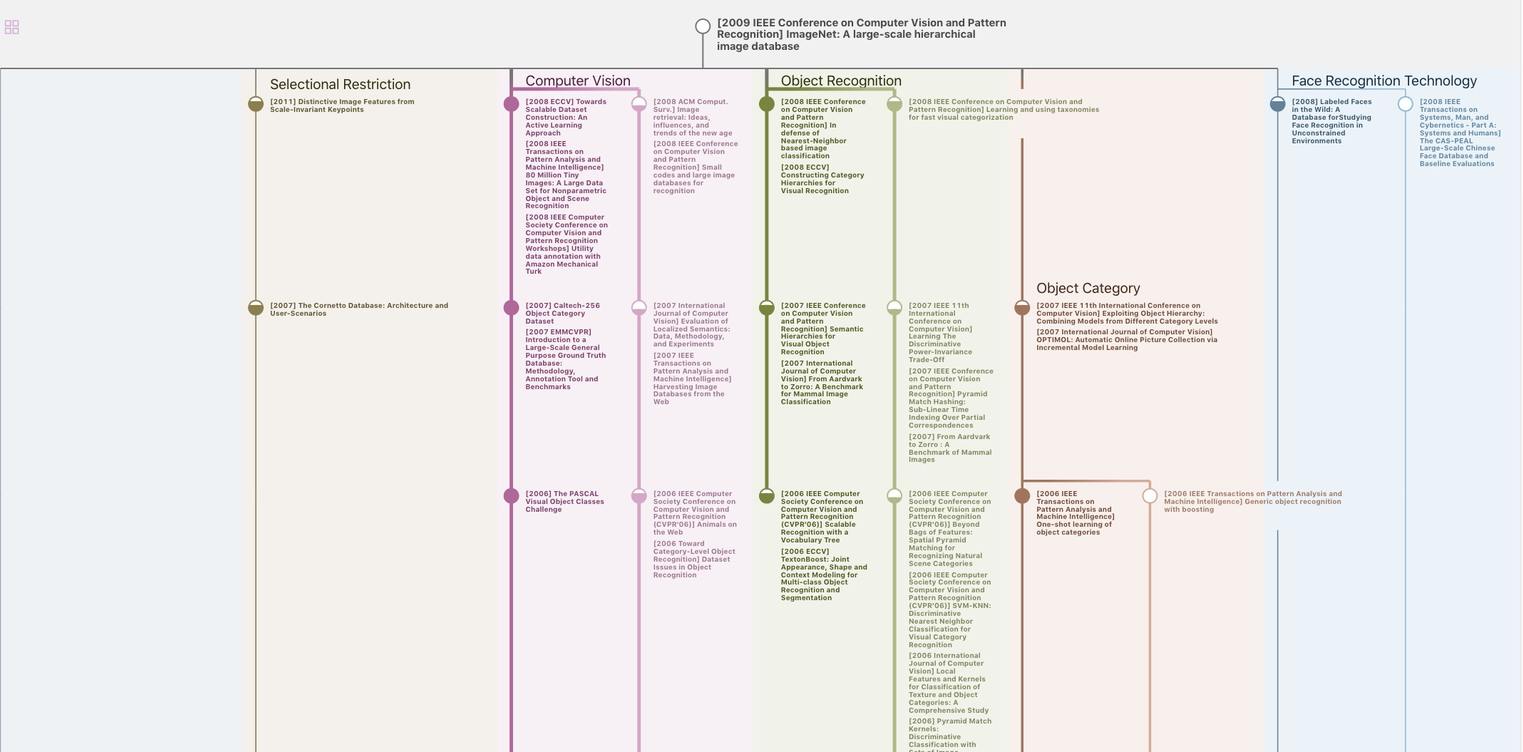
Generate MRT to find the research sequence of this paper
Chat Paper
Summary is being generated by the instructions you defined