Highly Imbalanced Classification of Gout Using Data Resampling and Ensemble Method
ALGORITHMS(2024)
Key words
gout disease,resampling method,machine learning,disease diagnosis,imbalance data,ensemble learning
AI Read Science
Must-Reading Tree
Example
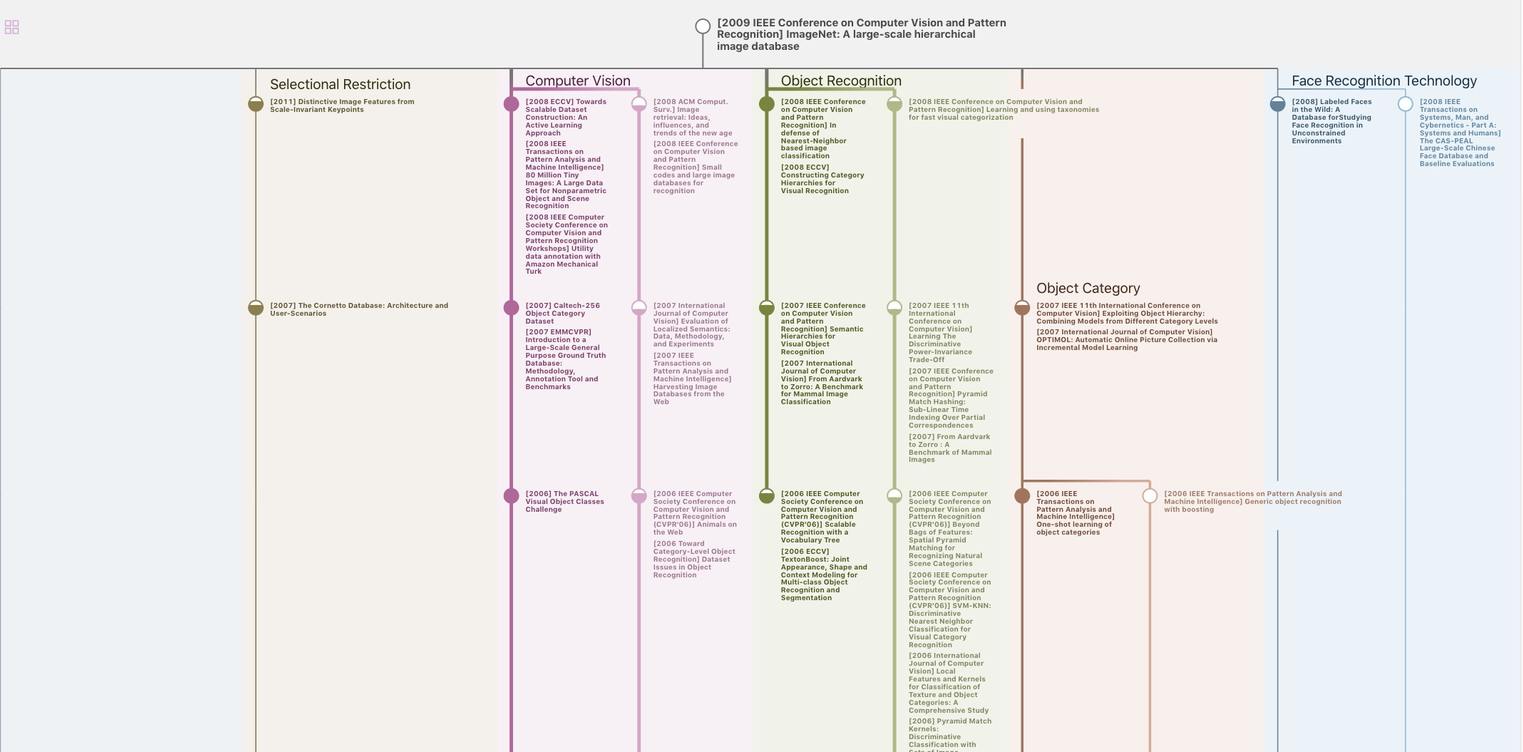
Generate MRT to find the research sequence of this paper
Chat Paper
Summary is being generated by the instructions you defined