SKIM: Skeleton-Based Isolated Sign Language Recognition With Part Mixing
IEEE TRANSACTIONS ON MULTIMEDIA(2024)
摘要
In this article, we present skeleton-based isolated sign language recognition (IsoSLR) with part mixing - SKIM. An IsoSLR model that solely takes the skeleton representation of the human body as input. Previous skeleton-based works either perform worse when compared to RGB-based counterparts or require fusion with other modalities to obtain competitive results. With SKIM, a single skeleton-based model without complex pre-training can obtain similar or even higher accuracy than current state-of-the-art methods. This margin can be further increased by simple late fusion within the same modality. To achieve this, we first develop a novel data augmentation technique called part mixing. It swaps the corresponding keypoints within one region (e.g. hand) between two randomly selected samples and combines their labels linearly as the new label. As regions like hand and face are key articulators for sign language, direct swapping of such parts creates a believable pseudo sign that promotes the model to recognize the true pairs. Secondly, following current advances in skeleton-based action recognition, we devise a channel-wise graph neural network with multi-scale awareness and per-keypoint temporal re-weighting. With this design, the backbone is capable of leveraging both manual and non-manual features. The combination of hand mixing and the channel-wise multi-scale GCN backbone allows us to achieve state-of-the-art accuracy on both WLASL and NMFs-CSL benchmarks.
更多查看译文
关键词
Sign language,Face recognition,Biological system modeling,Manuals,Benchmark testing,Assistive technologies,Data augmentation,sign language recognition,skeleton
AI 理解论文
溯源树
样例
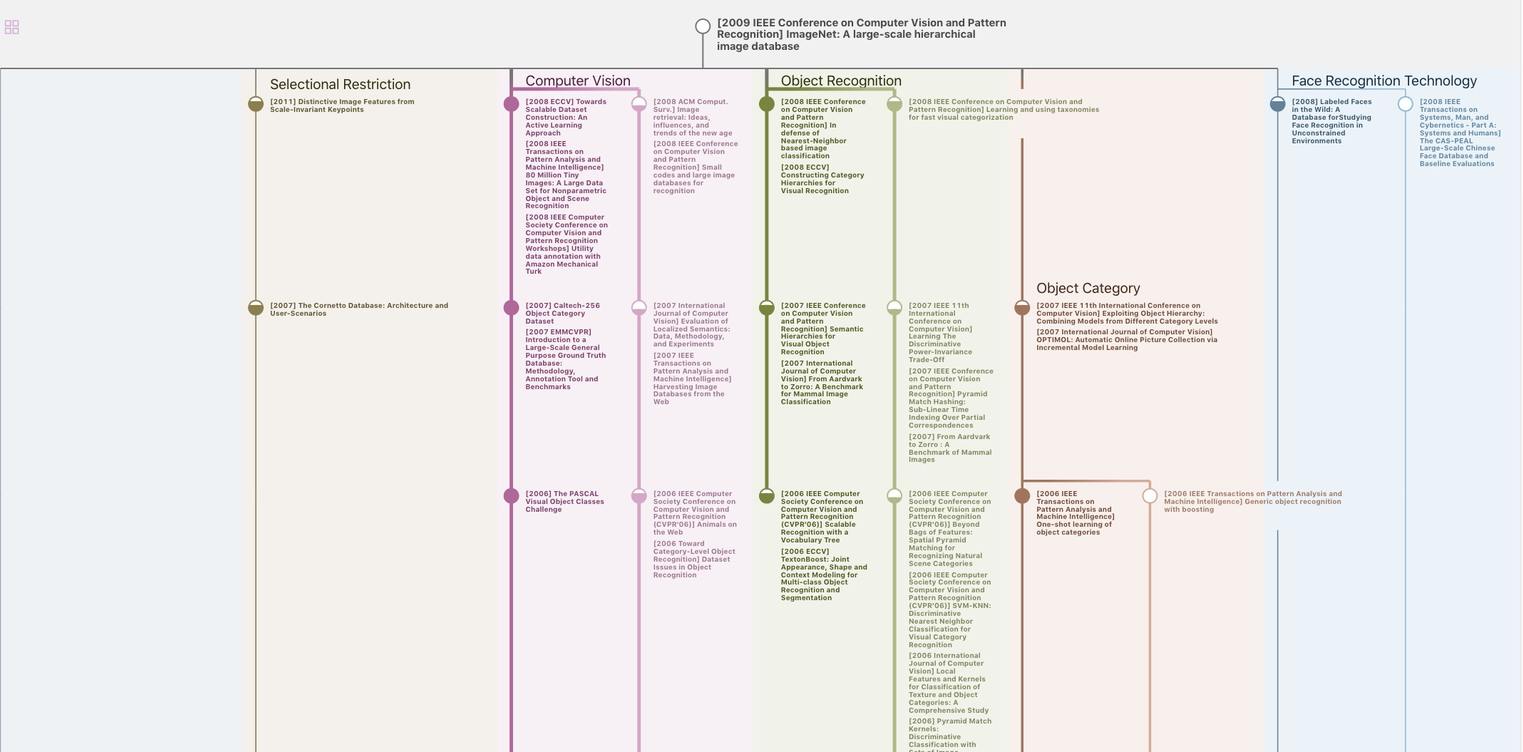
生成溯源树,研究论文发展脉络
Chat Paper
正在生成论文摘要