Identifying Anterior Cruciate Ligament Injuries Through Automated Video Analysis of In-Game Motion Patterns
ORTHOPAEDIC JOURNAL OF SPORTS MEDICINE(2024)
关键词
anterior cruciate ligament injury,artificial intelligence,biomechanics,motion analysis,video analysis
AI 理解论文
溯源树
样例
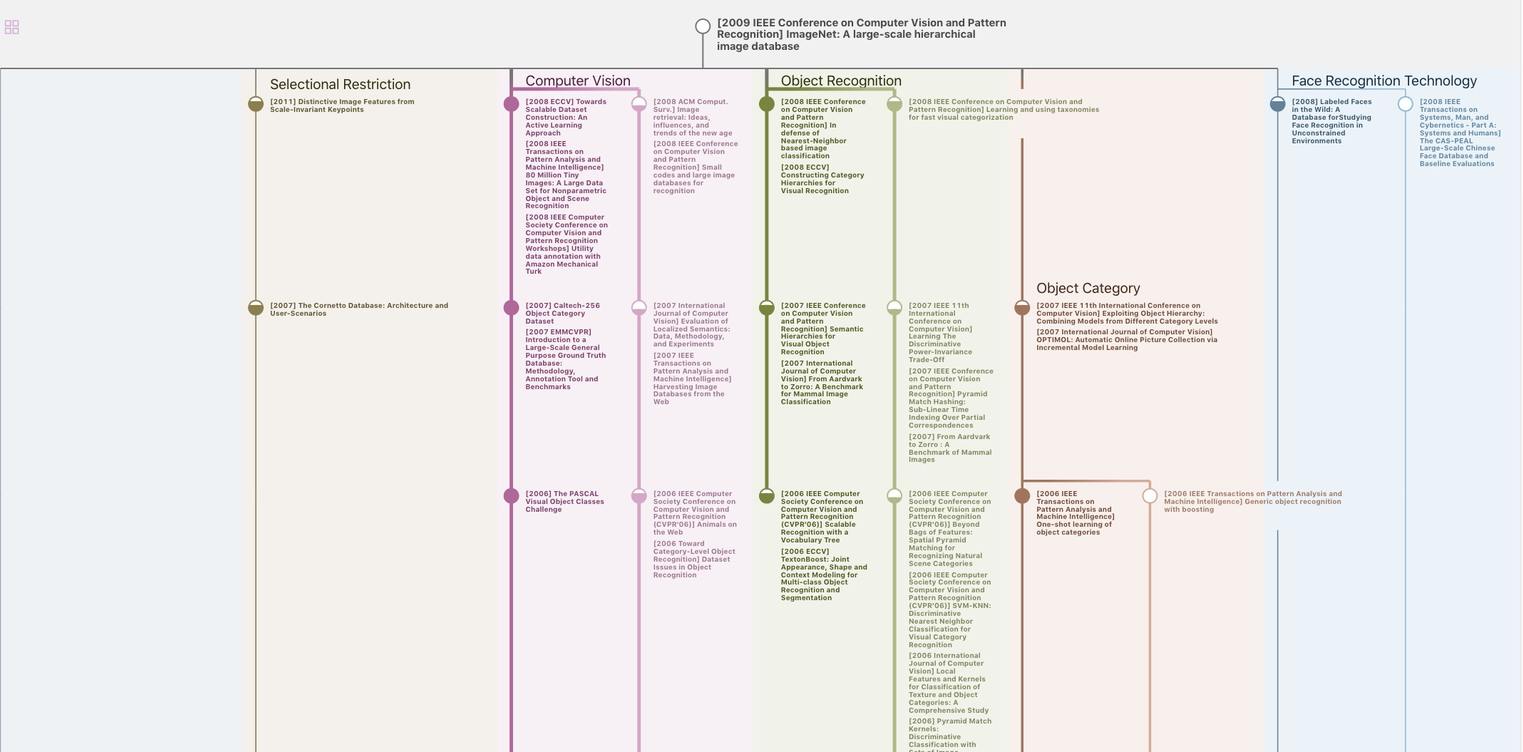
生成溯源树,研究论文发展脉络
Chat Paper
正在生成论文摘要