High-resolution spatiotemporal measurement and city-scale modelling of air and noise pollution in Accra Ghana: sensors, deep learning, and street-view imagery
crossref(2024)
摘要
Air and noise pollution are significant emerging environmental health hazards in African cities, with potentially complex spatial and temporal patterns. Limited local data are a major barrier to the formulation and evaluation of policies to reduce air and noise pollution.We designed and carried out an innovative 3-year measurement campaign to characterise air and noise pollution and their sources at high-resolution within the Greater Accra Metropolitan Area (GAMA), Ghana. Our design used a combination of fixed (3 year-long, n=10) and rotating (week-long over 1 year, n =136) sites, selected to represent a range of land uses and source influences. We collected data on PM2.5, black carbon (BC), nitrogen oxides (NOx), weather variables, noise pollution, along with street level time-lapse images with cameras. To do this, we strategically deployed low-cost, low-power, lightweight monitoring devices in an integrated station that was robust, socially unobtrusive, and able to function in the West African coastal climate. We used spatiotemporal land use regression models to predict PM2.5, NO2, BC and noise pollution across the city in high spatial resolution, and state-of-the-art methods in deep learning to predict pollution levels in high temporal resolution by training classification algorithms on 2 million time-lapse images captured at street level with corresponding pollution measurements. Most measurement sites recorded air pollution and noise levels above the WHO health-based guidelines. Spatiotemporal LUR models achieved good out of sample R2’s of 0.51-0.54 (noise), 0.58 – 0.83 (PM2.5), 0.78 - 0.80 (NO2) and 0.79 – 0.88 (BC). From the deep learning image-based analysis, the classification (prediction) accuracy of noise levels in space and time was higher (40-70%) than PM2.5 (30-55%), due to the localised nature of noise source emissions, and the fine-grained nature of our classes, which distinguish between small changes than previous studies. Our approach to monitoring and modelling air and noise pollution can be scaled up in other SSA cities to fill critical data gaps, and is already being successfully piloted in Kigali, Rwanda. The exposure surfaces developed with the LUR models are now supporting ongoing epidemiological studies assessing the impact of exposure to air and noise on birth outcomes and child health and development in Accra. Street view imagery are an increasingly available resource in cities around the world (from CCTV; Google Street View), and results from our deep learning image-based analysis show that the time lapsed images are a uniquely informative source of data for predicting high resolution temporal change in exposure, simultaneously with the presence or absence of potential determinants, though integrating with high spatial resolution remains a challenge.
更多查看译文
AI 理解论文
溯源树
样例
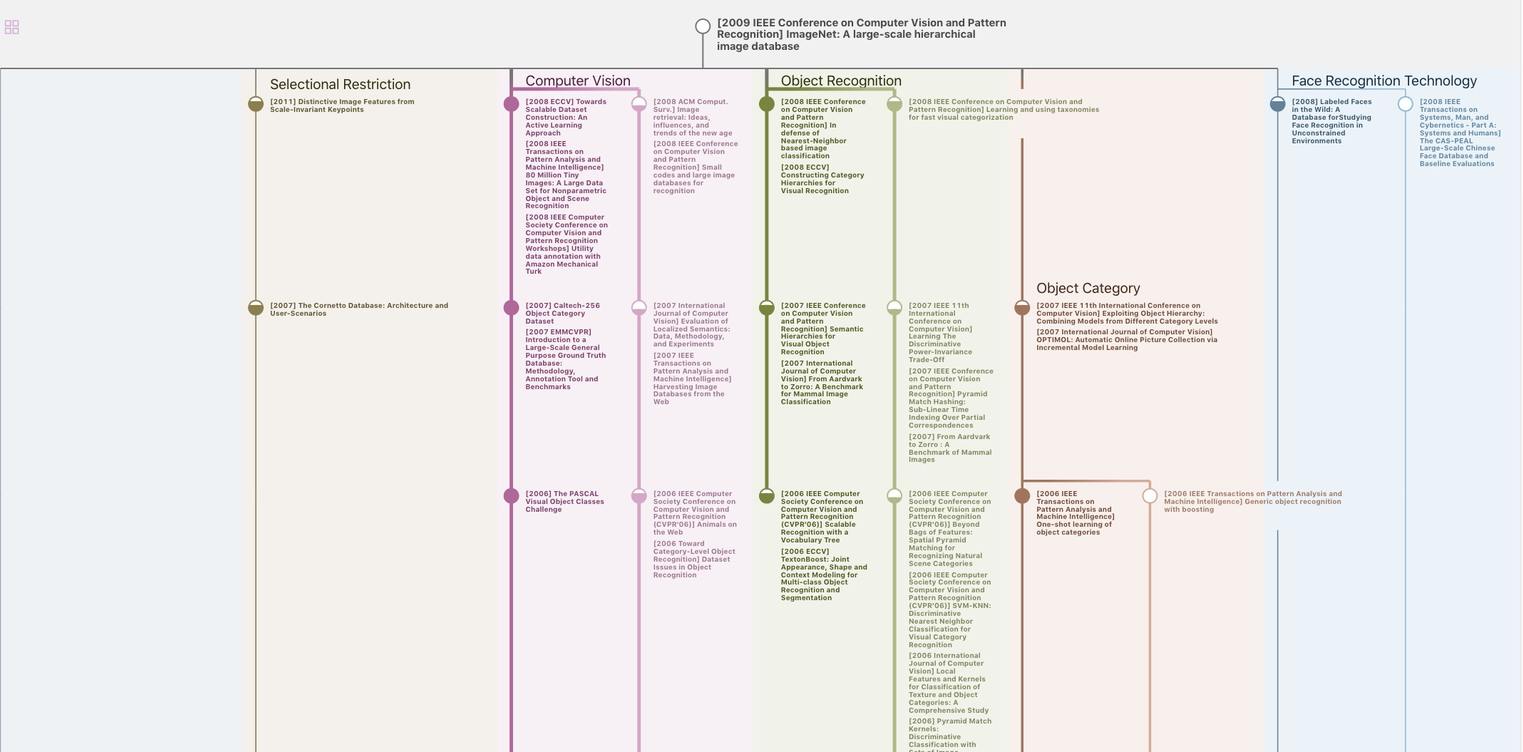
生成溯源树,研究论文发展脉络
Chat Paper
正在生成论文摘要