ACMFNet: Asymmetric Convolutional Feature Enhancement and Multiscale Fusion Network for Change Detection
IEEE JOURNAL OF SELECTED TOPICS IN APPLIED EARTH OBSERVATIONS AND REMOTE SENSING(2024)
关键词
Feature extraction,Convolution,Remote sensing,Decoding,Deep learning,Network architecture,Fuses,Asymmetric convolution,change detection (CD),multiscale fully convolutional Siamese network,multiscale fusion (MSF)
AI 理解论文
溯源树
样例
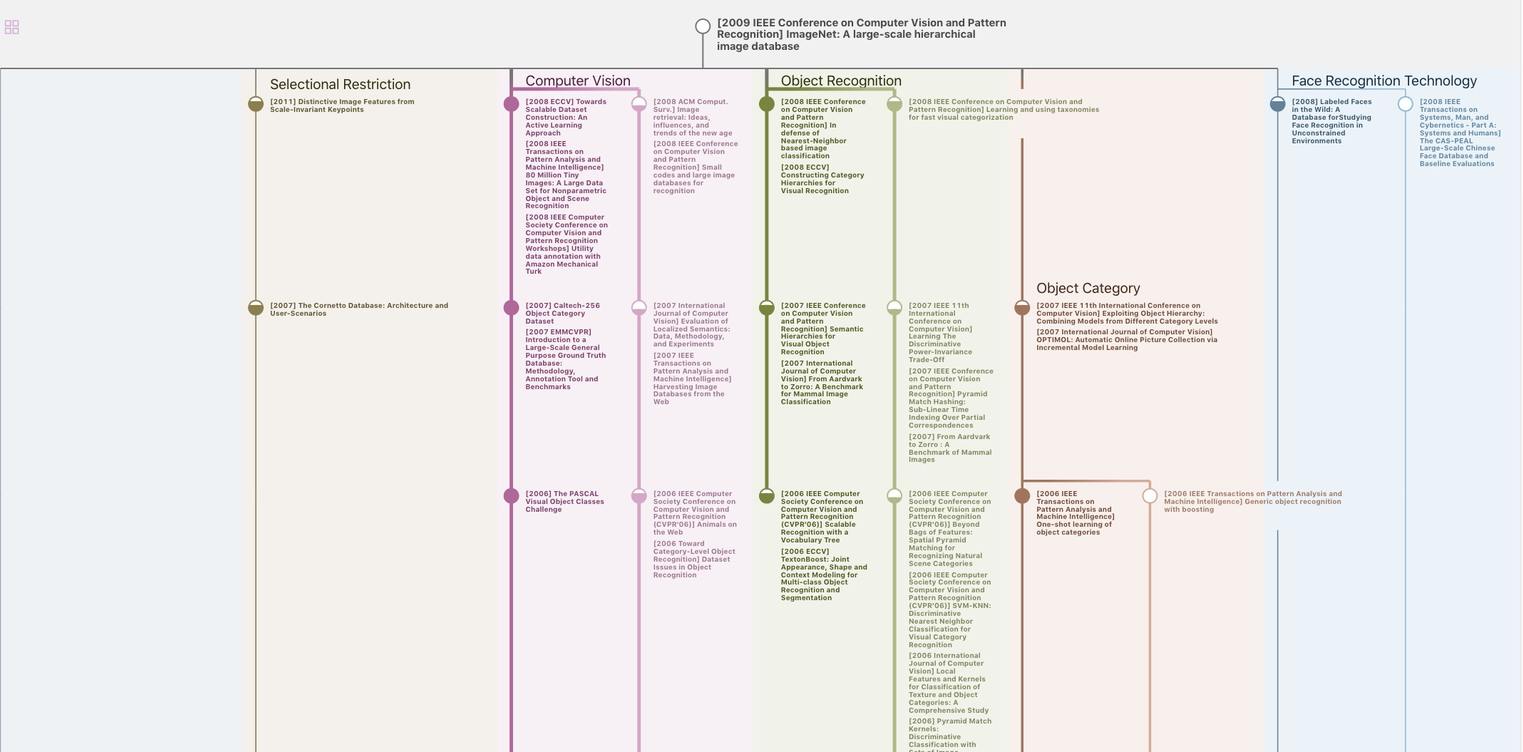
生成溯源树,研究论文发展脉络
Chat Paper
正在生成论文摘要