Operation Condition Assessment for Elevators Based on Deep Siamese Network and T-S Semi-Supervision Model
IEEE TRANSACTIONS ON INSTRUMENTATION AND MEASUREMENT(2024)
摘要
As a significant vertical means of transportation in daily life, the elevator's operation condition and performance level are closely related to the passengers' safety. However, there still lacks systematic and advanced method for the condition evaluation at present. Therefore, a semi-supervised two-stage deep learning (DL) network composed of feature selector and classifier is proposed to implement elevator condition assessment in this article. First, through the analysis of elevator mechanism and structure, vibration and noise indicators are selected as evaluation indexes to evaluate the elevator's state during operation process. Second, a comparative experiment is designed to collect signal data that reflect different performance levels, and the time-frequency feature parameters that can comprehensively provide the operation process information are extracted from the indicators' data. In addition, with purpose of carrying out effective space conversion and explore the complex relationship between features and categories, a deep Siamese multihead self-attention network is established to spatially map the extracted feature data, contributing to the clustering effect of data in high-dimensional space. To deal with the difficult access to label data in the actual assessment process, the teacher-student (T-S) network is adopted to carry out the semi-supervised training through the mixture of labeled data obtained from designed experiment and unlabeled data. Moreover, the Soft-match is introduced to address the imbalance between quantity and quality of pseudolabels in semi-supervised training. Finally, different comparative experiments and ablation experiment are conducted to verify the effectiveness and feasibility of the proposed method on the test dataset.
更多查看译文
关键词
Elevators,Feature extraction,Automobiles,Vibrations,Safety,Indexes,Training,Condition assessment,deep Siamese multihead self-attention network,soft-match,teacher-student (T-S) network,time-frequency feature
AI 理解论文
溯源树
样例
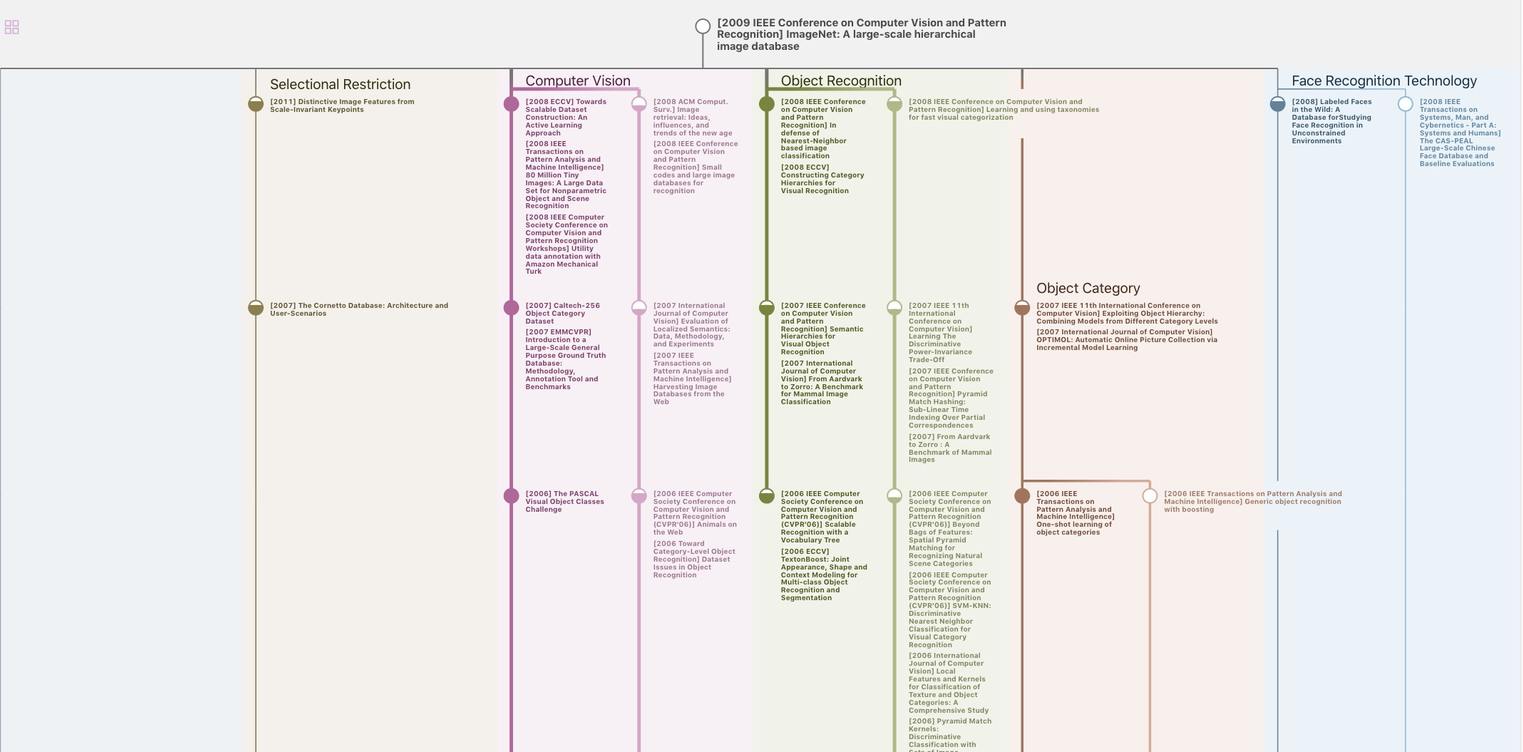
生成溯源树,研究论文发展脉络
Chat Paper
正在生成论文摘要