Shear Bearing Capacity Prediction of STRC Shear Walls Using Data‐augmented Fusion Model
STRUCTURAL CONCRETE(2024)
关键词
fusion model,machine learning,shear bearing capacity,steel tube-reinforced concrete shear walls
AI 理解论文
溯源树
样例
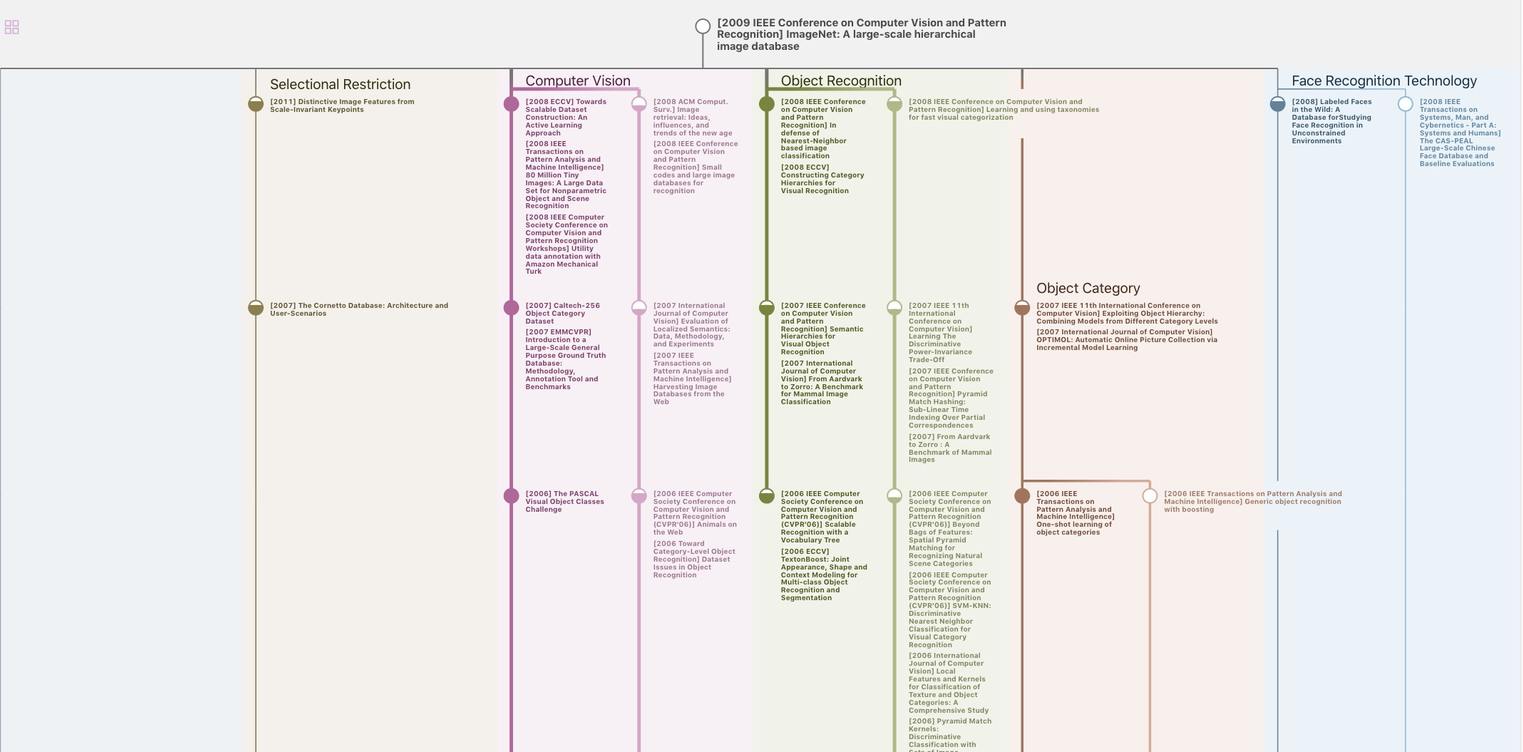
生成溯源树,研究论文发展脉络
Chat Paper
正在生成论文摘要