Noise misleads rotation invariant algorithms on sparse targets
arxiv(2024)
摘要
It is well known that the class of rotation invariant algorithms are
suboptimal even for learning sparse linear problems when the number of examples
is below the "dimension" of the problem. This class includes any gradient
descent trained neural net with a fully-connected input layer (initialized with
a rotationally symmetric distribution). The simplest sparse problem is learning
a single feature out of d features. In that case the classification error or
regression loss grows with 1-k/n where k is the number of examples seen.
These lower bounds become vacuous when the number of examples k reaches the
dimension d.
We show that when noise is added to this sparse linear problem, rotation
invariant algorithms are still suboptimal after seeing d or more examples. We
prove this via a lower bound for the Bayes optimal algorithm on a rotationally
symmetrized problem. We then prove much lower upper bounds on the same problem
for simple non-rotation invariant algorithms. Finally we analyze the gradient
flow trajectories of many standard optimization algorithms in some simple cases
and show how they veer toward or away from the sparse targets.
We believe that our trajectory categorization will be useful in designing
algorithms that can exploit sparse targets and our method for proving lower
bounds will be crucial for analyzing other families of algorithms that admit
different classes of invariances.
更多查看译文
AI 理解论文
溯源树
样例
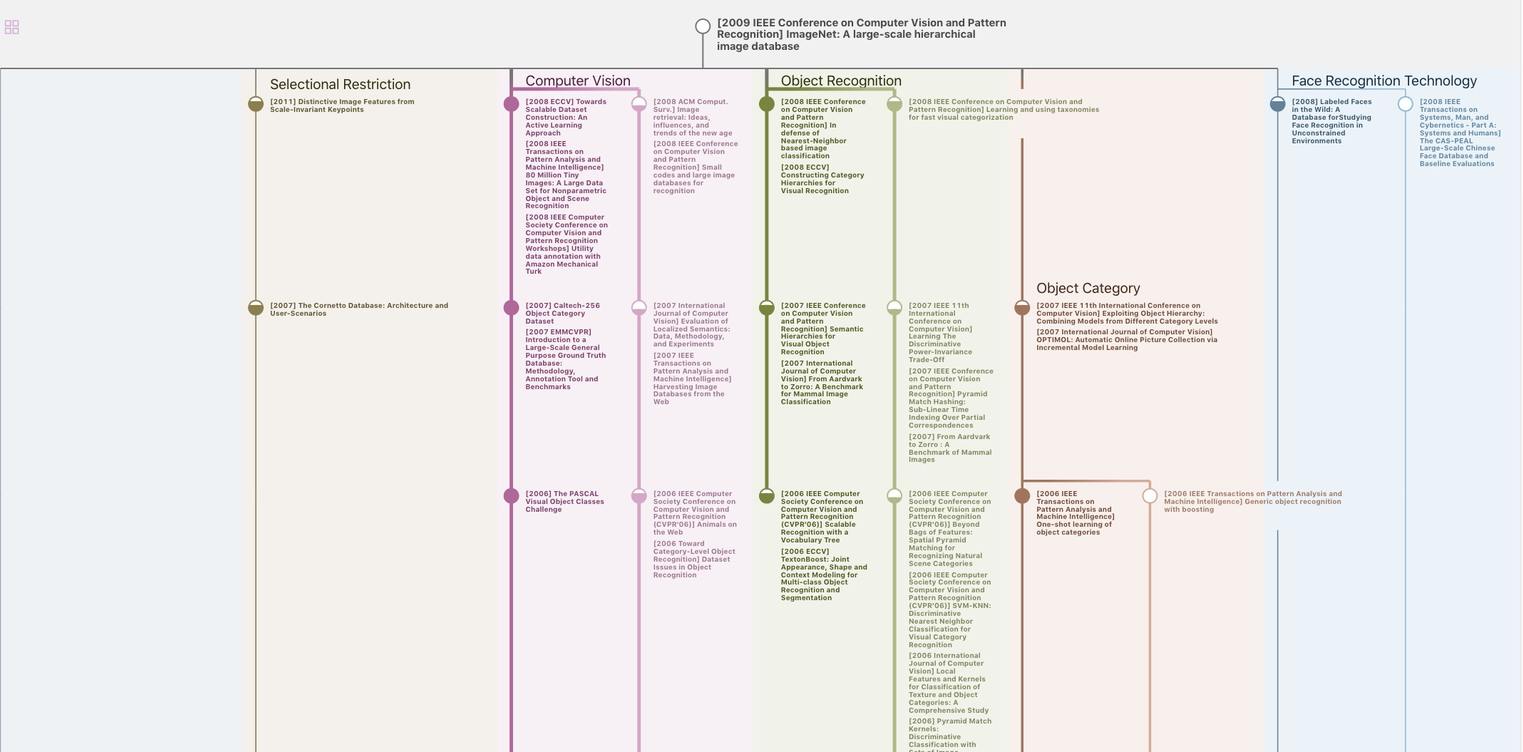
生成溯源树,研究论文发展脉络
Chat Paper
正在生成论文摘要