Pooling Image Datasets with Multiple Covariate Shift and Imbalance
ICLR 2024(2024)
Key words
image harmonization,medical imaging
AI Read Science
Must-Reading Tree
Example
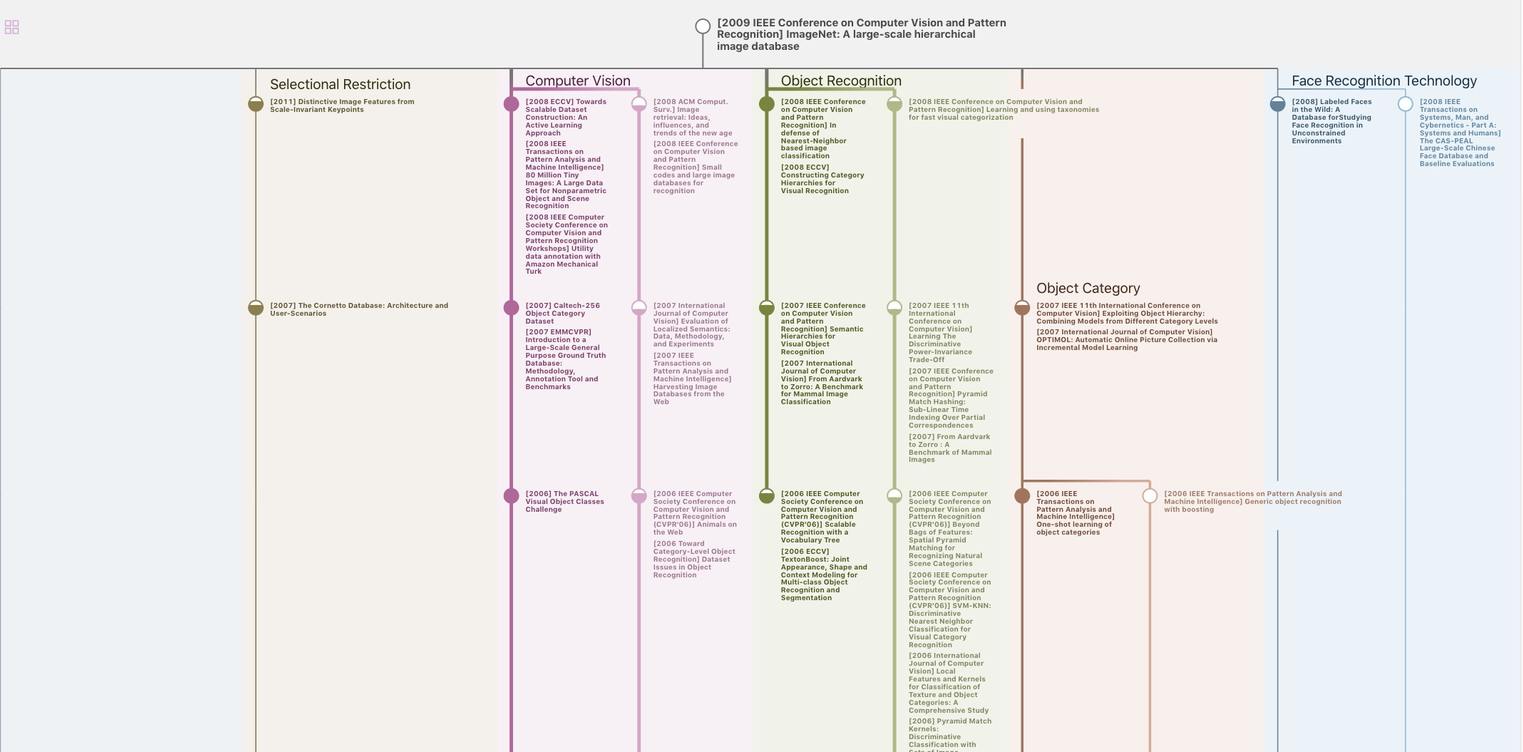
Generate MRT to find the research sequence of this paper
Chat Paper
Summary is being generated by the instructions you defined