Smaller Texture Improves Flexibility of Crystalline Silicon Solar Cells
Materials Letters(2024)
Key words
Solar energy materials,Surfaces,Texture
AI Read Science
Must-Reading Tree
Example
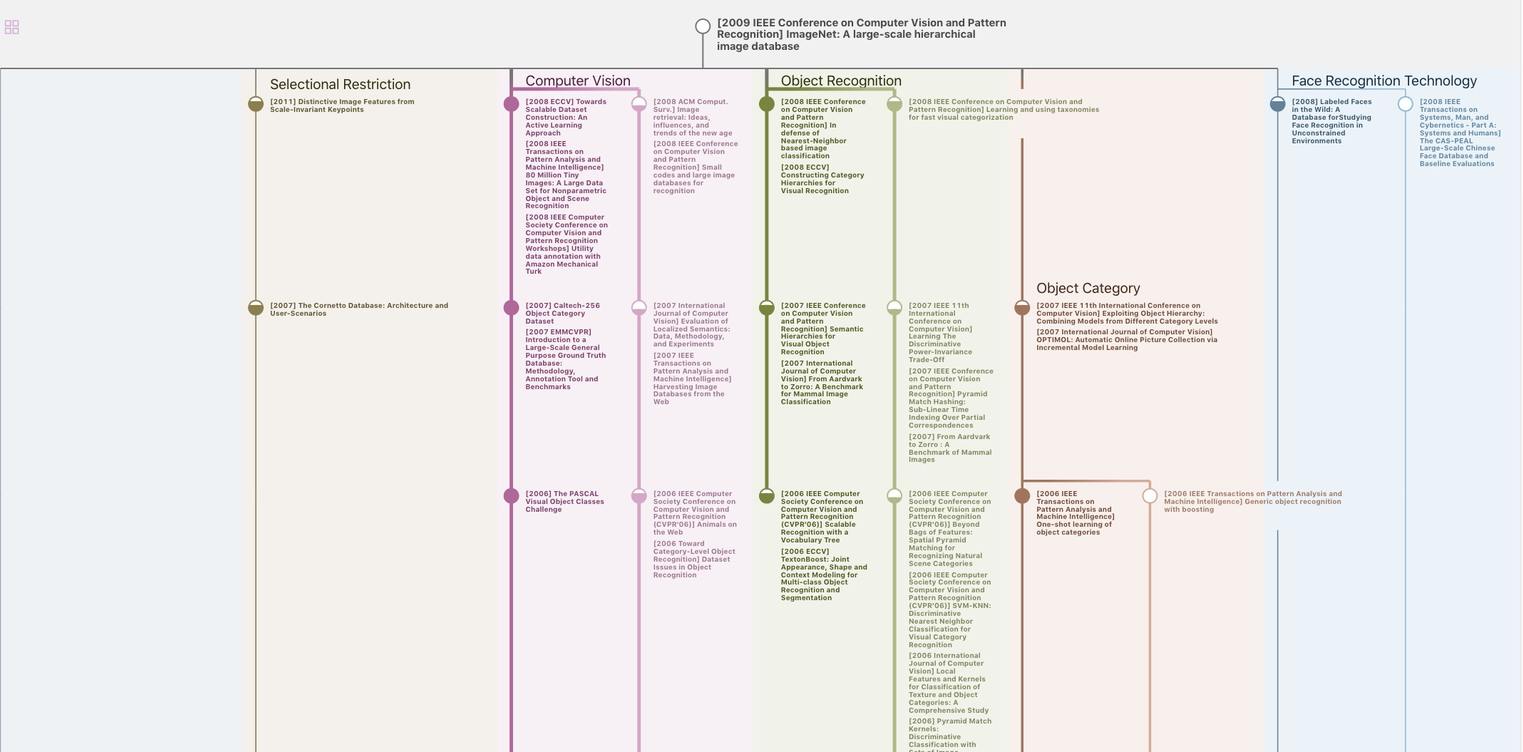
Generate MRT to find the research sequence of this paper
Chat Paper
Summary is being generated by the instructions you defined