Deep reinforcement learning for the direct optimization of gradient separations in liquid chromatography
JOURNAL OF CHROMATOGRAPHY A(2024)
摘要
While Reinforcement Learning (RL) has already proven successful in performing complex tasks, such as controlling large-scale epidemics, mitigating influenza and playing computer games beyond expert level, it is currently largely unexplored in the field of separation sciences. This paper therefore aims to introduce RL, specifically proximal policy optimization (PPO), in liquid chromatography, and evaluate whether it can be trained to optimize separations directly, based solely on the outcome of a single generic separation as input, and a reward signal based on the resolution between peak pairs (taking a value between [-1,1]). More specifically, PPO algorithms or agents were trained to select linear (1-segment) or multi-segment (2-, 3-, or 16-segment) gradients in 1 experiment, based on the outcome of an initial, generic linear gradient (phi(start) = 0.3, phi(end) = 1.0, and t(g) = 20 min), to improve separations. The size of the mixtures to be separated varied between 10 and 20 components. Furthermore, two agents, selecting 16-segment gradients, were trained to perform this optimization using either 2 or 3 experiments, in sequence, to investigate whether the agents could improve separations further, based on previous outcomes. Results showed that the PPO agent can improve separations given the outcome of one generic scouting run as input, by selecting phi-programs tailored to the mixture under consideration. Allowing agents more freedom in selecting multi-segment gradients increased the reward from 0.891 to 0.908 on average; and allowing the agents to perform an additional experiment increased the reward from 0.908 to 0.918 on average. Finally, the agent outperformed random experiments as well as standard experiments (phi(start) = 0.0, phi(end) = 1.0, and t(g) = 20 min) significantly; as random experiments resulted in average rewards between 0.220 and 0.283, and standard experiments resulted in average rewards of 0.840. In conclusion, while there is room for improvement, the results demonstrate the potential of RL in chromatography and present an interesting future direction for the automated optimization of separations.
更多查看译文
关键词
Proximal Policy Optimization,Agents,Deep learning,Method optimization
AI 理解论文
溯源树
样例
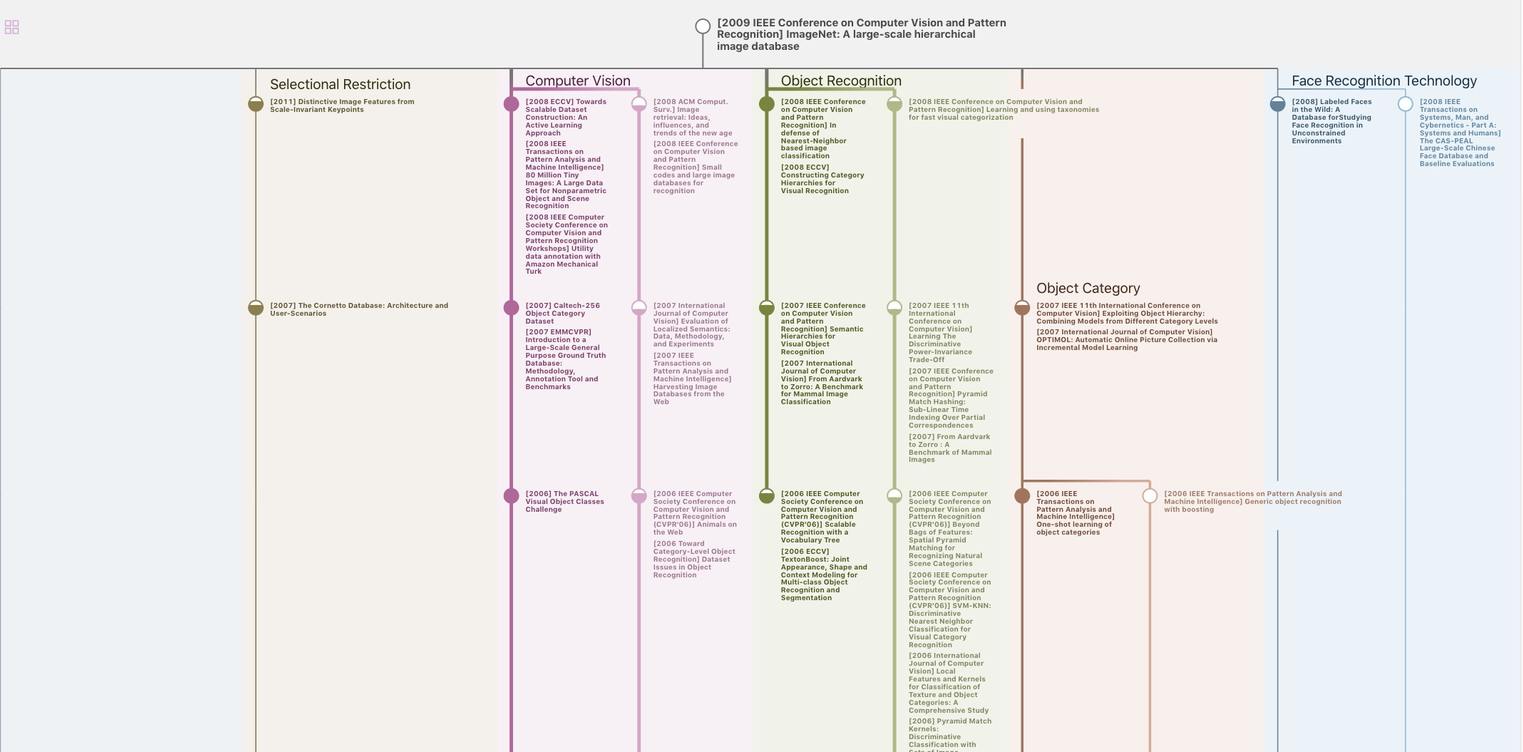
生成溯源树,研究论文发展脉络
Chat Paper
正在生成论文摘要