Surrogate-assisted Monte Carlo Tree Search for real-time video games
Engineering Applications of Artificial Intelligence(2024)
摘要
Monte Carlo Tree Search (MCTS) is a pronounced empirical search algorithm for agent decision-making, especially when enhanced by Deep Learning (DL), in mastering board games that were once thought to be unconquerable. However, it does not appear to be as equally successful in the domain of real-time video games, where the simulation time limit for exploration is a crucial factor, since they are generally designed to be played by human users and hence require a significant amount of resources for simulation. We in this paper propose a surrogate-assisted MCTS approach, specifically targeting commercial real-time video games by approximating the result of gameplay with a deep-learning-based surrogate model. The key contribution of our work is that we designed a modified MCTS for video games that are both commercial and processed in real-time. Since commercial video games include considerably more complex and dynamic gameplays to satisfy their market consumers, as opposed to their non-commercial analogs, our work can be regarded as having challenged the domain unattempted by precedent studies. We validated the performance of our method by conducting a comparative experiment with other algorithms, including the traditional MCTS, under the environment of a commercial real-time video game.
更多查看译文
关键词
Monte Carlo Tree Search,Surrogate model,Neural network,Commercial real-time video game
AI 理解论文
溯源树
样例
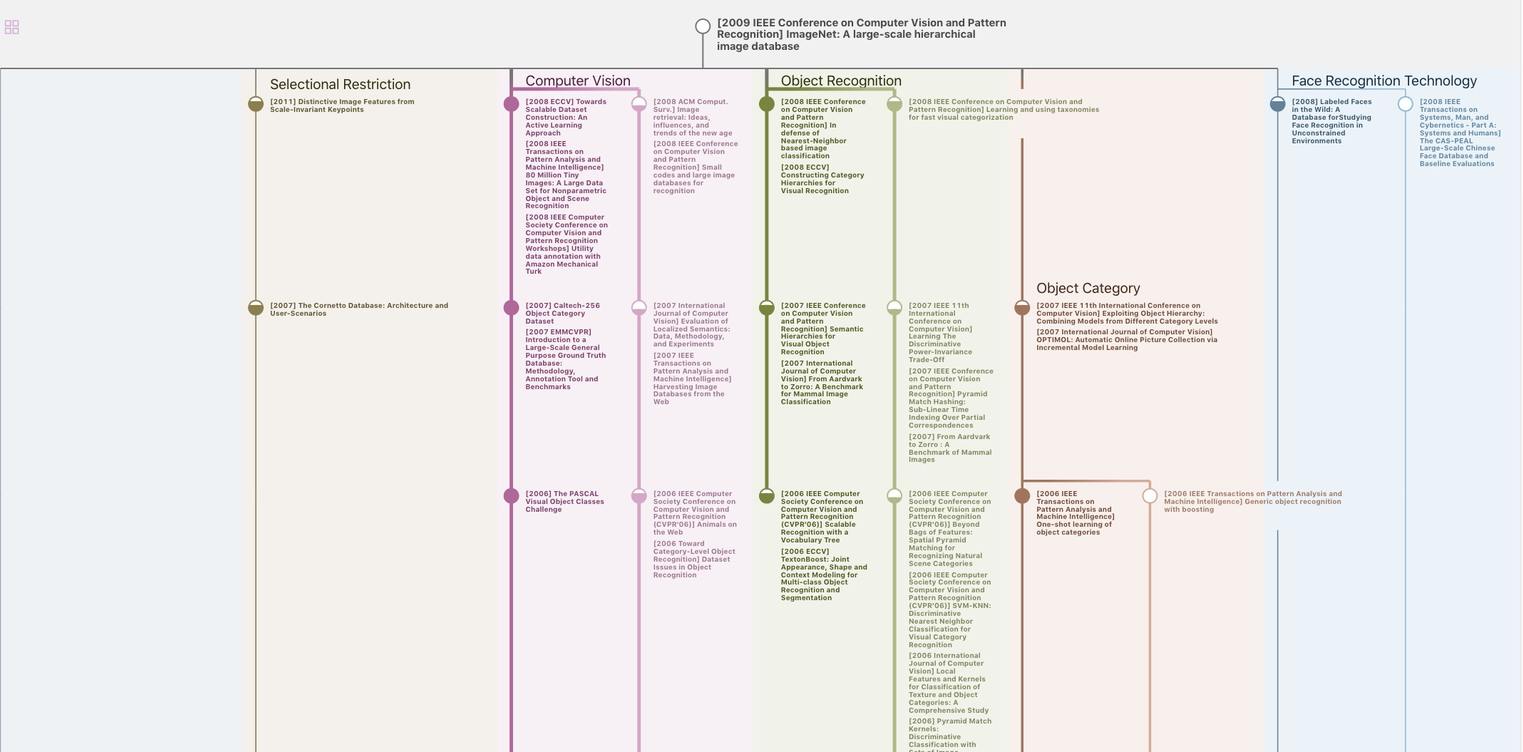
生成溯源树,研究论文发展脉络
Chat Paper
正在生成论文摘要