Wind Power Forecasting: A Transfer Learning Approach Incorporating Temporal Convolution and Adversarial Training
RENEWABLE ENERGY(2024)
Key words
Transfer learning,Adversarial training,Temporal convolutional network,Distribution shift
AI Read Science
Must-Reading Tree
Example
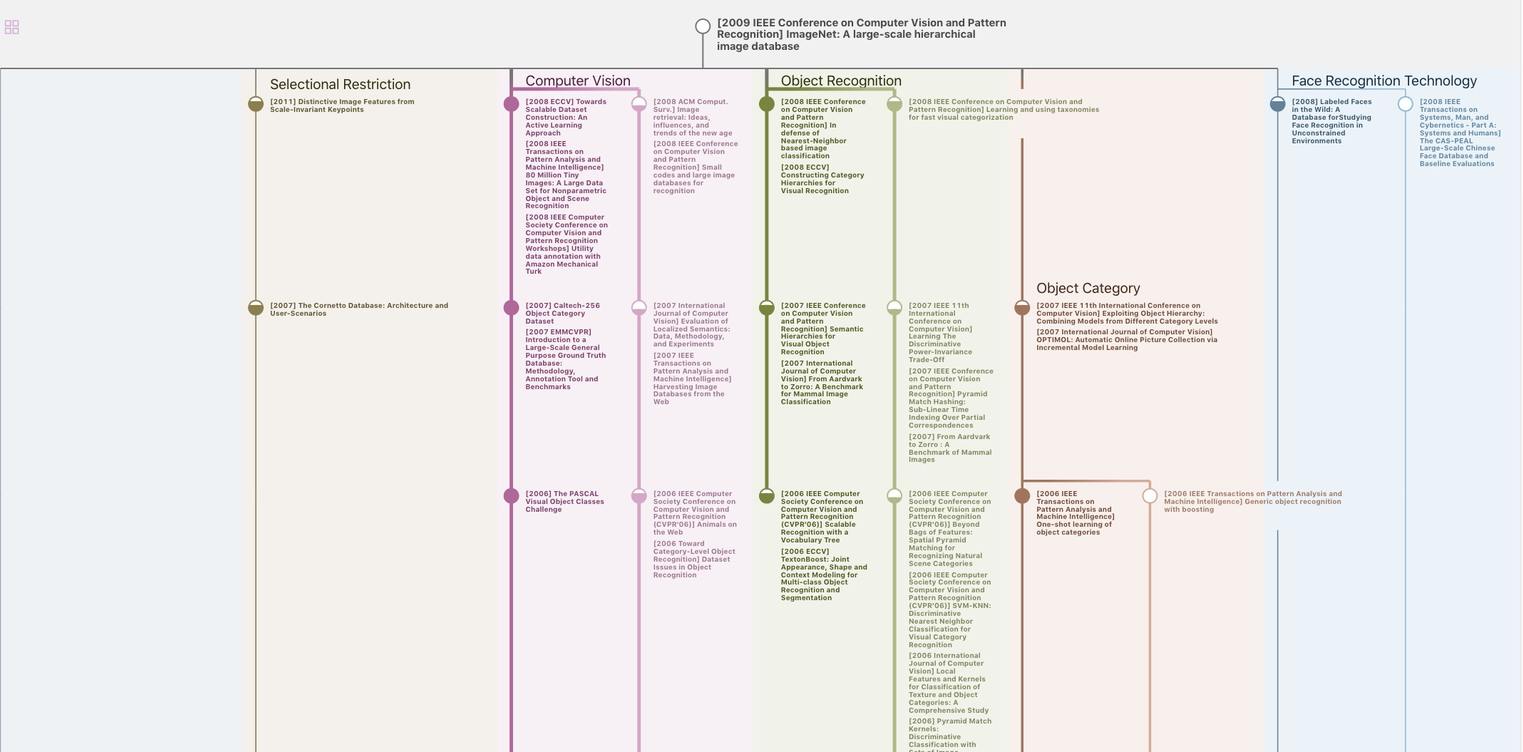
Generate MRT to find the research sequence of this paper
Chat Paper
Summary is being generated by the instructions you defined