Video generalized semantic segmentation via Non-Salient Feature Reasoning and Consistency
Knowledge-Based Systems(2024)
摘要
Video semantic segmentation is beneficial for dynamic scene processing in real-world environments, and achieves superior performance on independent and identically distributed data. However, it suffers from performance degradation in environments with various domain styles, which is known as the distribution shift problem. Although some previous studies on image generalized semantic segmentation considered the distribution shift problem, temporal-frame information could not be used to obtain more accurate prediction. Thus, in this study, we explore a new task, known as the video generalized semantic segmentation (VGSS) task, which establishes a connection between continuous frames and domain generalization. We propose a novel method named Non-Salient Feature Reasoning and Consistency (NSFRC) for this task. Specifically, we first define the class-wise non-salient feature, which describes the features of the class-wise non-salient region that carry more generalized information. We then propose a class-wise non-salient feature reasoning strategy to select and enhance generalized channels adaptively. This strategy adopts a new form to use domain-invariant features by treating the domain-invariant features as prior information to assist domain-invariant model learning. Finally, we propose a non-salient centroid alignment loss to alleviate the temporally inconsistent and negative transfer problems in the VGSS task. We also extend our video-based framework to the image generalized semantic segmentation (IGSS) task. Experiments demonstrate that our NSFRC framework yields significant improvements in both the VGSS and IGSS tasks. To explain the idea of this research in a clear and attractive way, we provide the visual abstract shown in Fig. 1.
更多查看译文
关键词
Semantic segmentation,Video domain generalization,Non-salient region,Class-wise relationship reasoning,Domain-invariant feature
AI 理解论文
溯源树
样例
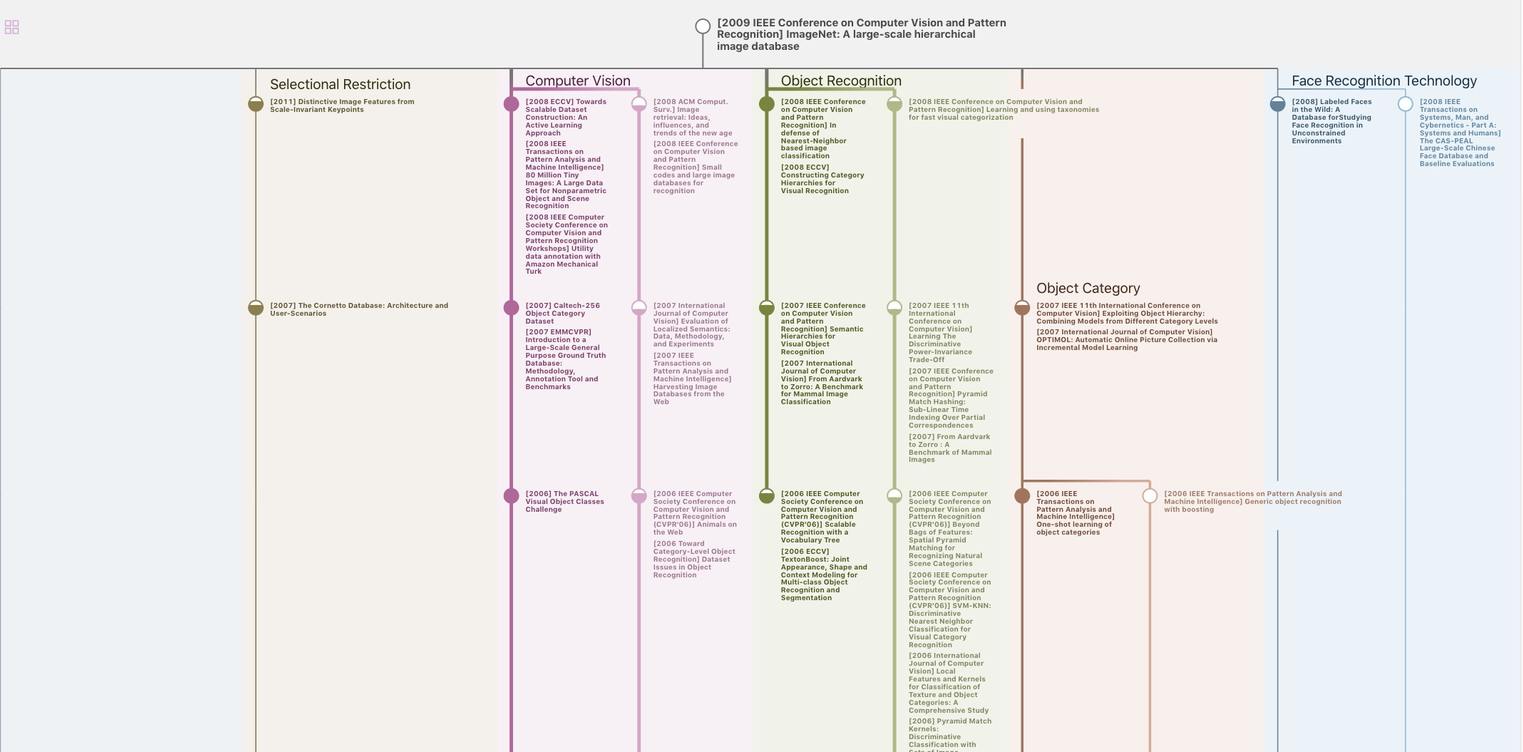
生成溯源树,研究论文发展脉络
Chat Paper
正在生成论文摘要