Hybrid Task Scheduling in Cloud Manufacturing With Sparse-Reward Deep Reinforcement Learning
IEEE TRANSACTIONS ON AUTOMATION SCIENCE AND ENGINEERING(2024)
摘要
Cloud manufacturing (CMfg) converts the traditional manufacturing system into an Internet-of-things-enabled (IoT-enabled) manufacturing system, where both manufacturing and computational tasks must be scheduled among distributed and heterogeneous resources. Deep reinforcement learning (DRL) has recently become a promising idea for task scheduling in CMfg. However, existing DRL-based methods depend heavily on problem-specific reward engineering and struggle to represent hybrid decision variables. To this end, this paper proposed the sparse-reward deep reinforcement learning (SDRL) method to solve the hybrid task scheduling problem in CMfg. First, the hybrid task scheduling model in CMfg is constructed to minimize the makespan. We reformulate the studied problem as a partially observable Markov decision process (POMDP). Then, the objective hindsight experience replay (objective HER) mechanism is proposed to alleviate the sparse reward issue, through which the scheduling policy can be effectively trained without problem-specific reward engineering. The continuous action space is defined to represent hybrid decision variables, and the implicit action-selection mapping is utilized to alleviate the boundary effect. Numerical experiments validated the effectiveness and superiority of our method compared to eleven popular scheduling algorithms including evolutionary algorithms and DRL. Compared to mainstream DRL scheduling methods, the proposed SDRL outperforms the second-best one at most by 23.6% regarding generalization, and a scheduling solution can be generated in 0.5 seconds.
更多查看译文
关键词
Job shop scheduling,Task analysis,Processor scheduling,Manufacturing,Dynamic scheduling,Computational modeling,Collaboration,Scheduling,cloud manufacturing,deep reinforcement learning,intelligent manufacturing,machine learning
AI 理解论文
溯源树
样例
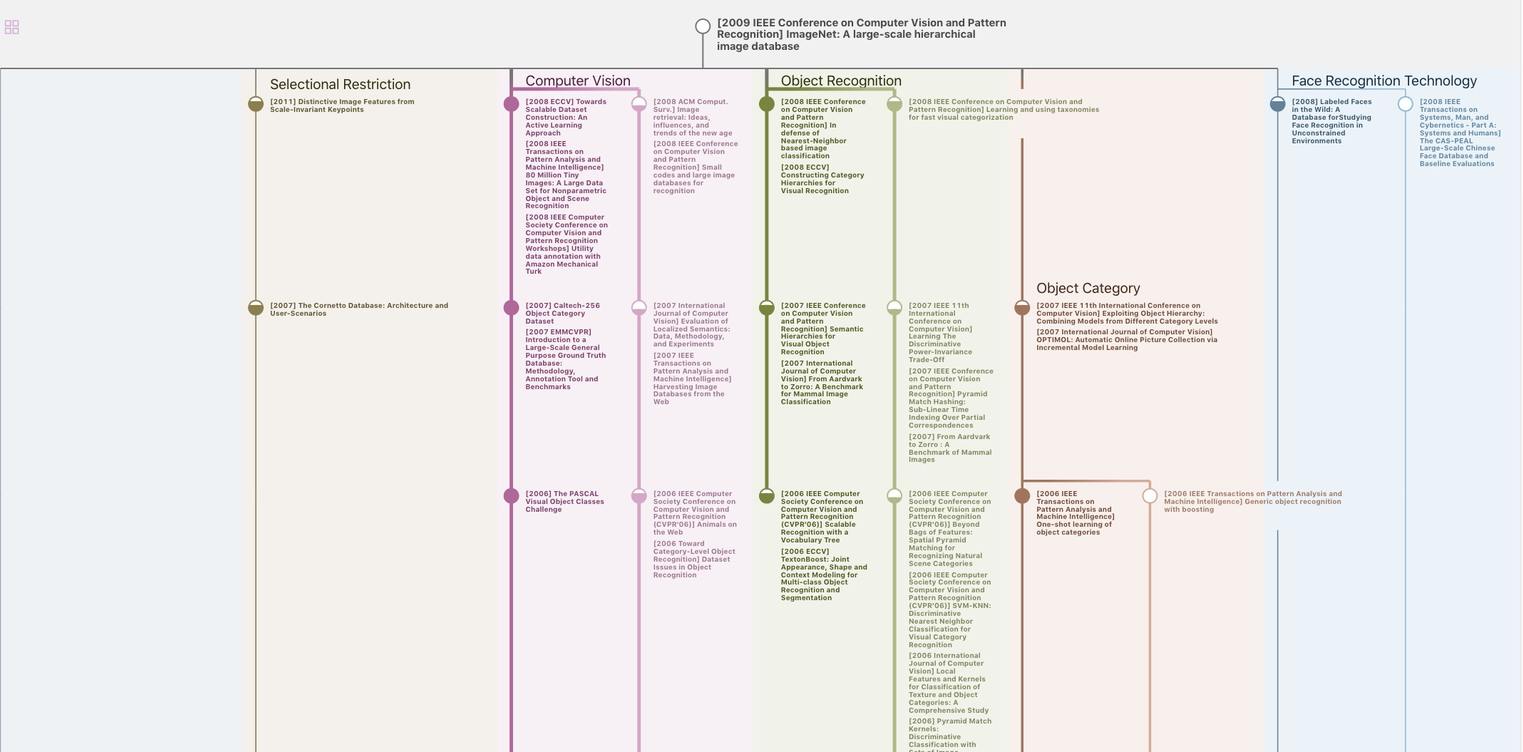
生成溯源树,研究论文发展脉络
Chat Paper
正在生成论文摘要