Training Unbiased Diffusion Models From Biased Dataset
ICLR 2024(2024)
摘要
With significant advancements in diffusion models, addressing the potential
risks of dataset bias becomes increasingly important. Since generated outputs
directly suffer from dataset bias, mitigating latent bias becomes a key factor
in improving sample quality and proportion. This paper proposes time-dependent
importance reweighting to mitigate the bias for the diffusion models. We
demonstrate that the time-dependent density ratio becomes more precise than
previous approaches, thereby minimizing error propagation in generative
learning. While directly applying it to score-matching is intractable, we
discover that using the time-dependent density ratio both for reweighting and
score correction can lead to a tractable form of the objective function to
regenerate the unbiased data density. Furthermore, we theoretically establish a
connection with traditional score-matching, and we demonstrate its convergence
to an unbiased distribution. The experimental evidence supports the usefulness
of the proposed method, which outperforms baselines including time-independent
importance reweighting on CIFAR-10, CIFAR-100, FFHQ, and CelebA with various
bias settings. Our code is available at https://github.com/alsdudrla10/TIW-DSM.
更多查看译文
关键词
diffusion model,density ratio estimation,dataset bias
AI 理解论文
溯源树
样例
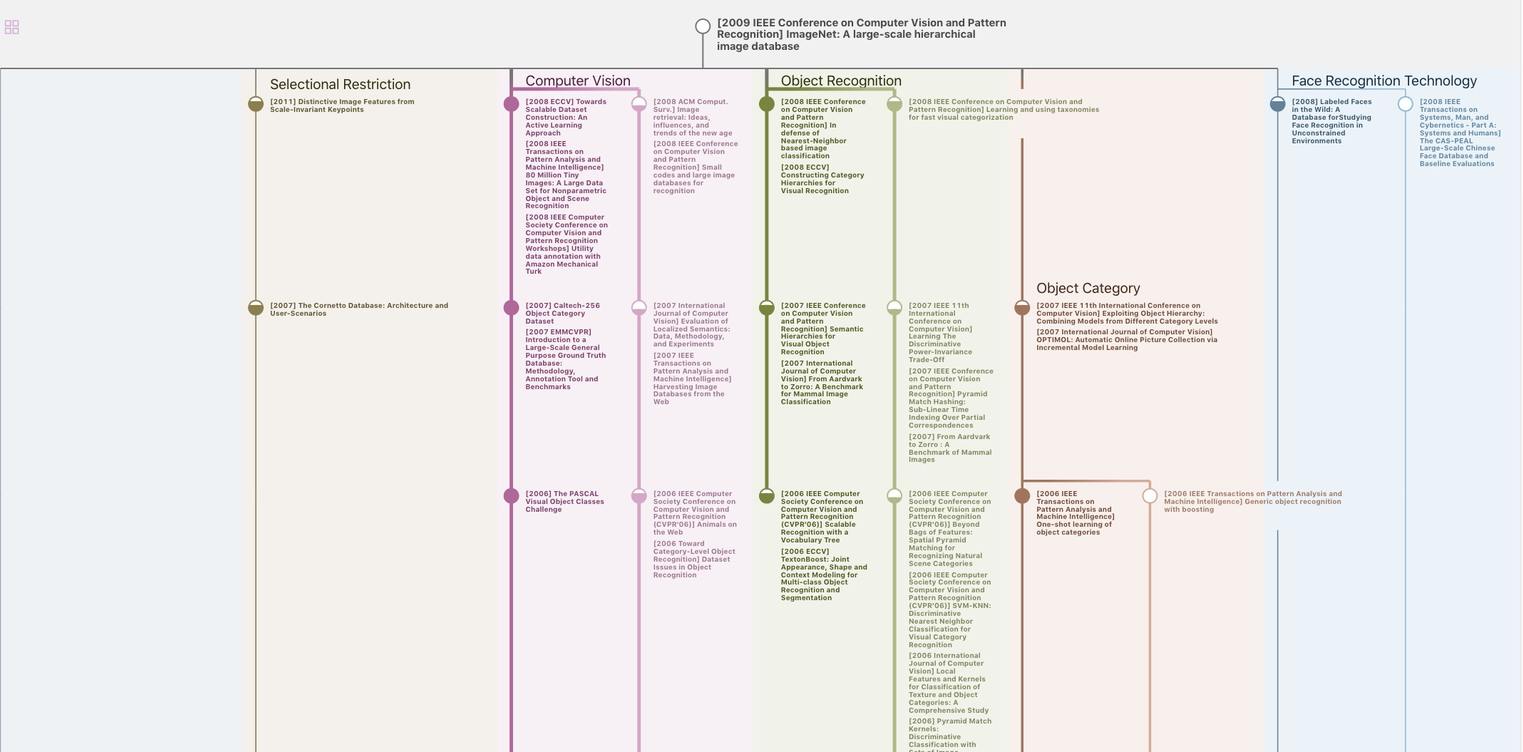
生成溯源树,研究论文发展脉络
Chat Paper
正在生成论文摘要